Cost-Sensitive Tensor-Based Dual-stage Attention LSTM with Feature Selection for Data Center Server Power Forecasting
ACM Transactions on Intelligent Systems and Technology(2022)
摘要
The power forecasting has a guiding effect on power-aware scheduling strategies to reduce unnecessary power consumption in data centers. Many metrics related to power consumption can be collected in physical servers, such as the status of CPU, memory, and other components. However, most existing methods empirically exploit a small number of metrics to forecast power consumption. To this end, this paper uses feature selection based on causality to explore the metrics that strongly influence the power consumption of different tasks. Moreover, we propose a tensor-based dual-stage attention LSTM to forecast the non-linear and non-periodic power consumption. In the proposed model, a multi-way delay embedding transform is utilized to convert the time series into tensors along the temporal direction. The LSTM combines with the tensor technique and the attention mechanism to capture the temporal pattern effectively. In addition, we adopt the cost-sensitive loss function to optimize the specific power forecasting problem in data center. The experimental results demonstrate that our method can achieve up to 1.4% - 4.3% forecasting accuracy improvement compared with the state-of-the-art models.
更多查看译文
关键词
Power forecasting,data center,time series,tensor,LSTM,feature selection
AI 理解论文
溯源树
样例
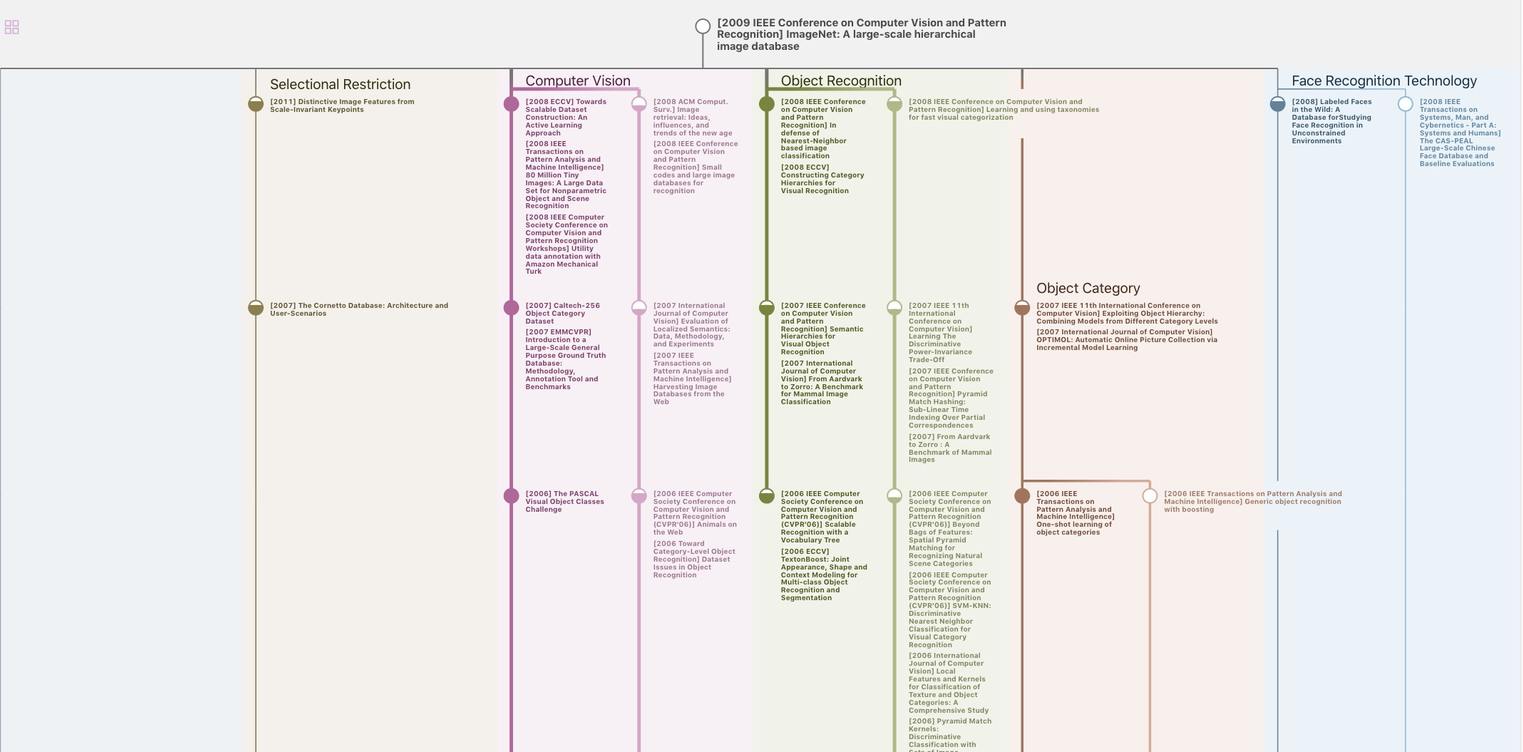
生成溯源树,研究论文发展脉络
Chat Paper
正在生成论文摘要