Data-Driven Modal Equivalent Standardization for Early Damage Detection in Bridge Structural Health Monitoring
Journal of Engineering Mechanics(2023)
摘要
Environment-induced nonlinear and seasonal variabilities in modal frequency are still a major challenge for bridge damage detection and condition assessment. Almost all techniques focus on the method's improvement and ignore its linear or multivariate Gaussian distribution assumptions with unsatisfactory detection accuracy. Little attention was given to the feasibility of environmental suppression before damage detection. A data-driven modal equivalent standardization (MES) method is developed without environmental measurements. The nearest neighbor modal set is first searched from the bridge modal baseline training database based on a similarity measure that corresponds to several smaller Euclidean distances. Then, the MES method is implemented by the localized mean and standard deviation. After this analysis, a damage detection model based on the slow feature analysis is established. A real bridge case verifies the method's validity in an environment-tolerant capacity, Gaussification of data distribution, and modal variable linearization, which outperforms global data standardization for damage detection.
更多查看译文
关键词
early damage detection,damage detection,modal equivalent standardization,bridge,data-driven
AI 理解论文
溯源树
样例
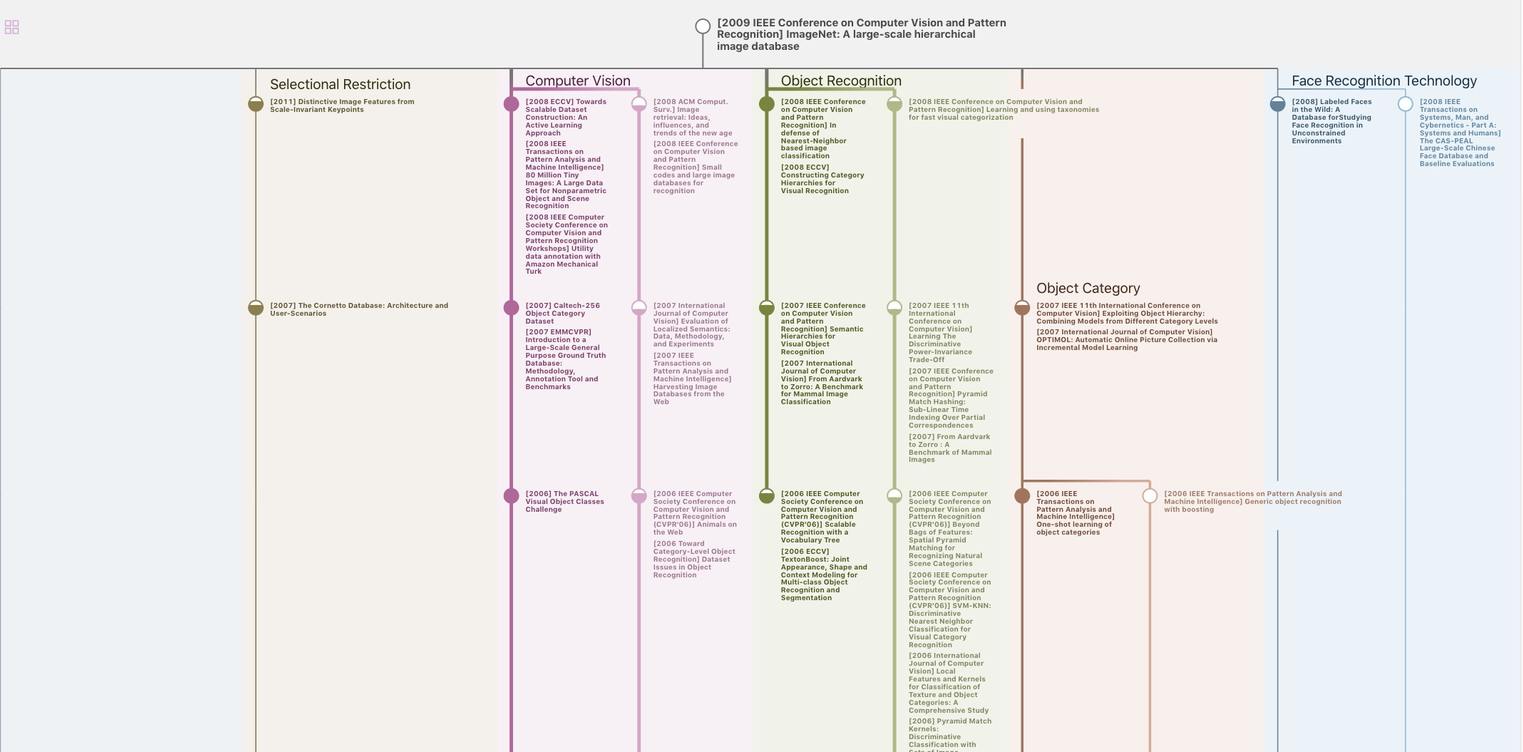
生成溯源树,研究论文发展脉络
Chat Paper
正在生成论文摘要