SPILL—Security Properties and Machine-Learning Assisted Pre-Silicon Laser Fault Injection Assessment
International Symposium for Testing and Failure Analysis ISTFA 2022 Conference Proceedings from the 48th International Symposium for Testing and Failure Analysis(2022)
Abstract
Abstract Laser-based fault injection (LFI) attacks are powerful physical attacks with high precision and controllability. Therefore, attempts have been in the literature to model and simulate the laser effect in pre-silicon digital designs. However, these efforts can only model the laser effect on small SPICE or TCAD circuits of individual standard cells. This paper proposes security properties and a machine-learning assisted layout signoff framework in verifying the full-chip layout's resiliency against LFI. In the framework, we leveraged the commercial SoC power integrity sign-off tool to inject the Gaussian laser current to any spot in the layout, by considering different layout features such as power distribution network, decoupling capacitor placement, metal geometry, instance switching power, etc. To avoid exhaustive analysis of all layout spots regardless of LFI criticality, we use security properties to drive the assessment and identify critical areas. We then use SPICE simulations and machine learning to develop cell-level laser fault models under different laser-induced transient current intensities. This laser cell library is used during full-chip LFI feasibility analysis for the cells inside laser illumination, enabling precise layout -level design fix for critical cells failing the fault injection threshold. Finally, we show the effectiveness of the proposed framework by analyzing the fully implemented AES design layout.
MoreTranslated text
AI Read Science
Must-Reading Tree
Example
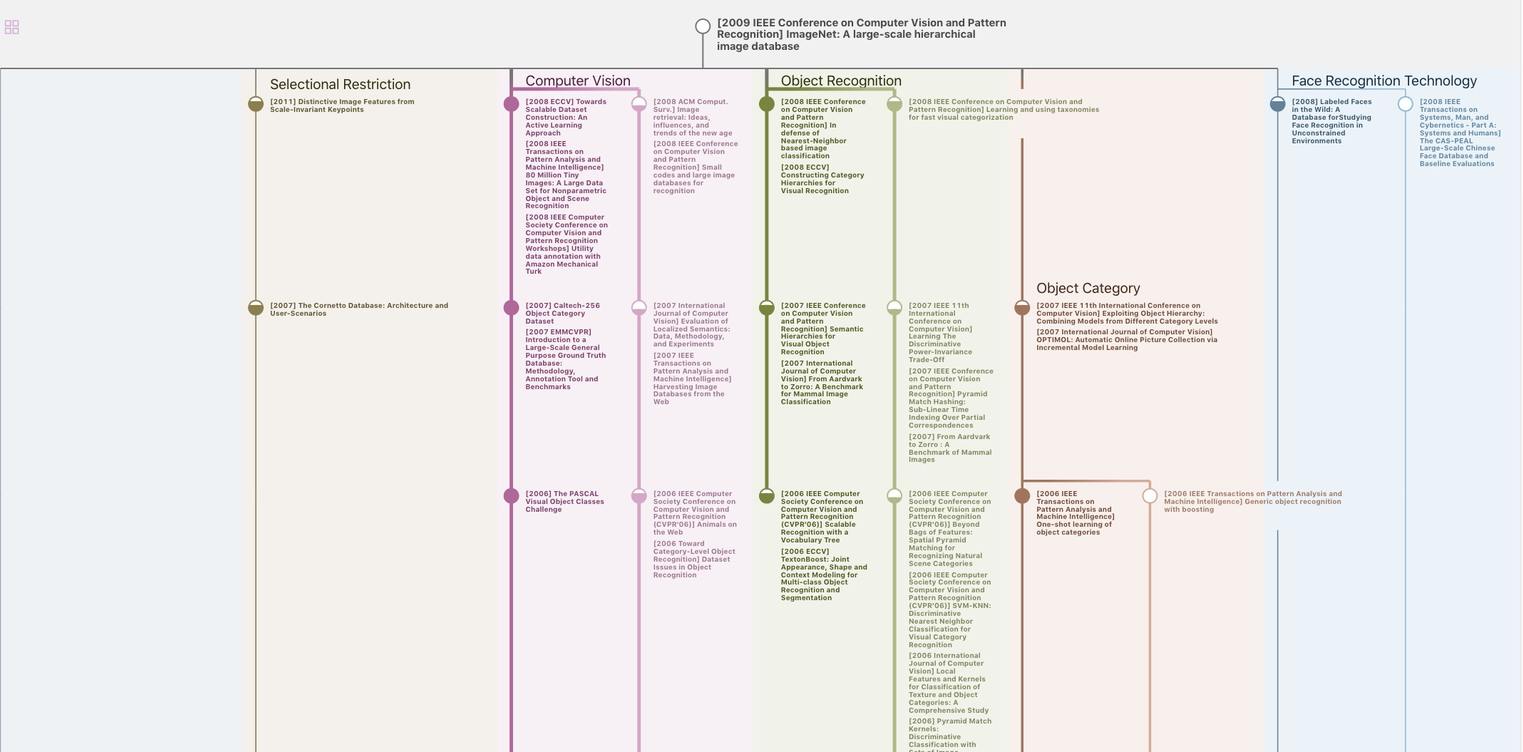
Generate MRT to find the research sequence of this paper
Chat Paper
Summary is being generated by the instructions you defined