A General Framework of Rotational Sparse Approximation in Uncertainty Quantification.
SIAM/ASA J. Uncertain. Quantification(2022)
摘要
This paper proposes a general framework for estimating coefficients of generalized polynomial chaos (gPC) used in uncertainty quantification (UQ) via rotational sparse approximation. In particular, we aim to identify a rotation matrix such that the gPC expansion of a set of random variables after the rotation has a sparser representation. However, this rotational approach alters the underlying linear system to be solved, which makes finding the sparse coefficients more difficult than in the case without rotation. To solve this problem, we examine several popular nonconvex regulariza-tions in compressive sensing (CS) that perform better than the classic 1 pound approach empirically. All these regularizations can be minimized by the alternating direction method of multipliers (ADMM). Numerical examples show superior performance of the proposed combination of rotation and non-convex sparsity-promoting regularizations over those with and without rotation but using the convex 1 pound approach.
更多查看译文
关键词
generalized polynomial chaos,uncertainty quantification,iterative rotations,compressive sensing,alternating direction,nonconvex regularization
AI 理解论文
溯源树
样例
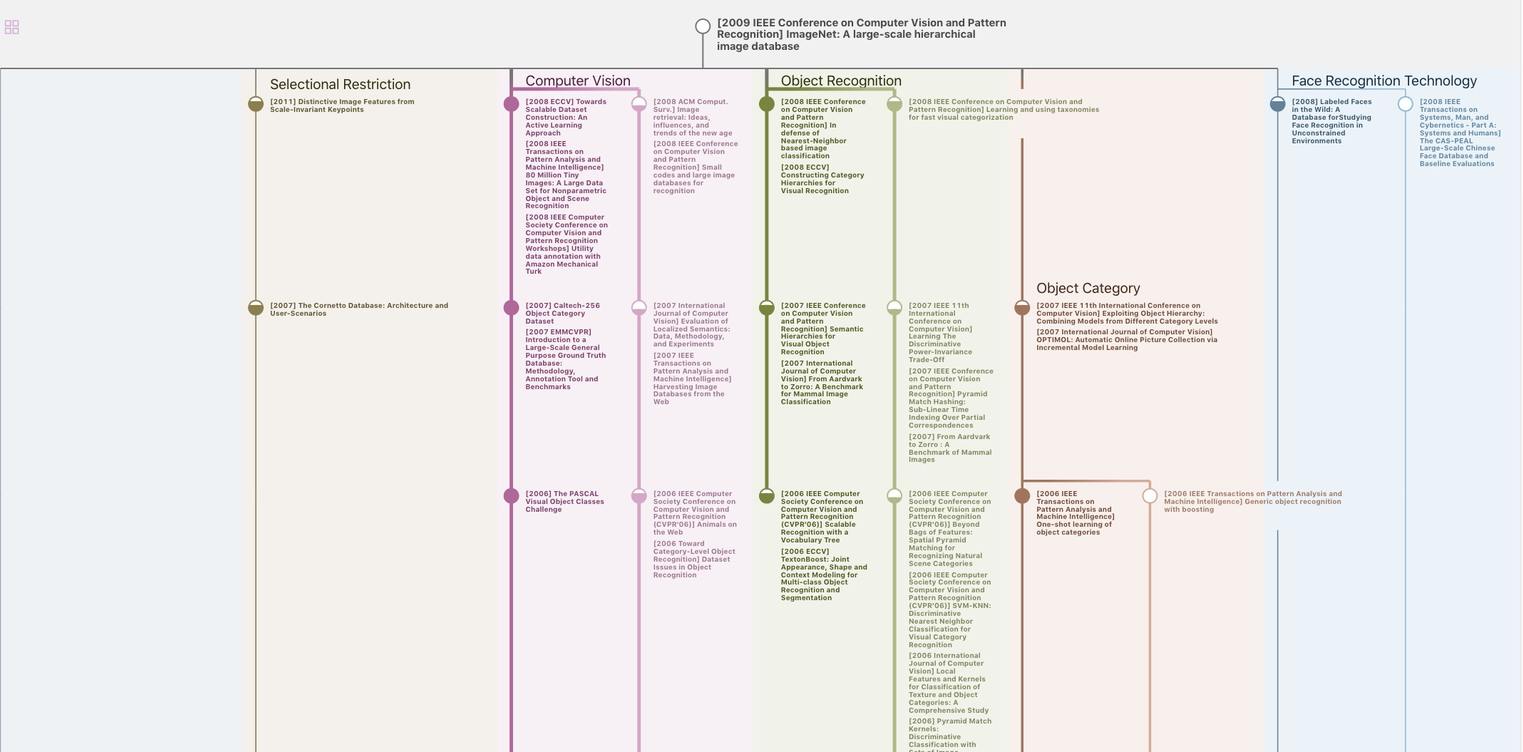
生成溯源树,研究论文发展脉络
Chat Paper
正在生成论文摘要