Robust Inference and Modeling of Mean and Dispersion for Generalized Linear Models
Journal of the American Statistical Association(2023)
摘要
Generalized Linear Models (GLMs) are a popular class of regression models when the responses follow a distribution in the exponential family. In real data the variability often deviates from the relation imposed by the exponential family distribution, which results in over- or underdispersion. Dispersion effects may even vary in the data. Such datasets do not follow the traditional GLM distributional assumptions, leading to unreliable inference. Therefore, the family of double exponential distributions has been proposed, which models both the mean and the dispersion as a function of covariates in the GLM framework. Since standard maximum likelihood inference is highly susceptible to the possible presence of outliers, we propose the robust double exponential (RDE) estimator. Asymptotic properties and robustness of the RDE estimator are discussed. A generalized robust quasi-deviance measure is introduced which constitutes the basis for a stable robust test. Simulations for binomial and Poisson models show the excellent performance of the RDE estimator and corresponding robust tests. Penalized versions of the RDE estimator are developed for sparse estimation with high-dimensional data and for flexible estimation via generalized additive models (GAMs). Real data applications illustrate the relevance of robust inference for dispersion effects in GLMs and GAMs. for this article are available online.
更多查看译文
关键词
Double exponential family,Influence function,Likelihood ratio test,M-estimator,Penalization
AI 理解论文
溯源树
样例
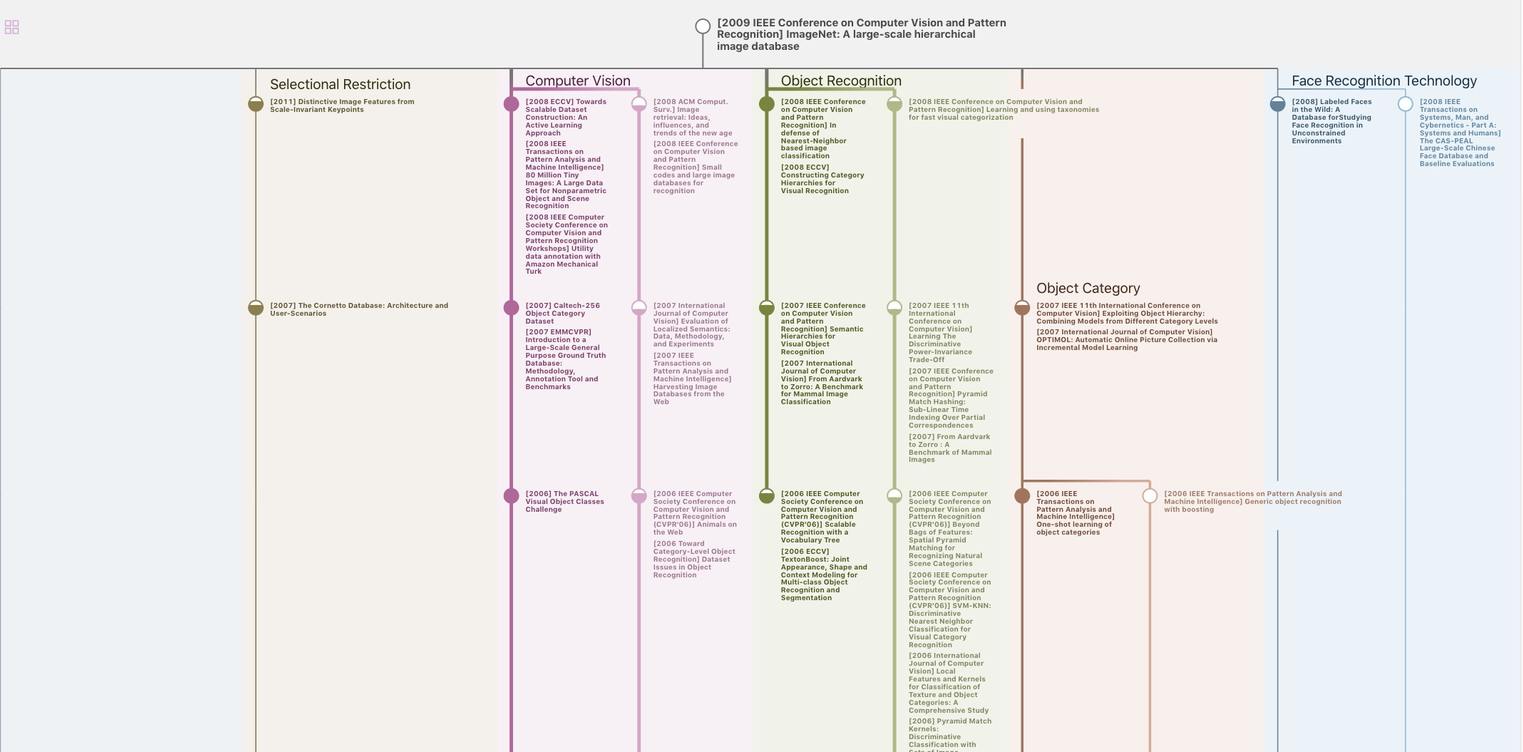
生成溯源树,研究论文发展脉络
Chat Paper
正在生成论文摘要