Can Transformer Attention Spread Give Insights Into Uncertainty of Detected and Tracked Objects?
arxiv(2022)
摘要
Transformers have recently been utilized to perform object detection and tracking in the context of autonomous driving. One unique characteristic of these models is that attention weights are computed in each forward pass, giving insights into the model's interior, in particular, which part of the input data it deemed interesting for the given task. Such an attention matrix with the input grid is available for each detected (or tracked) object in every transformer decoder layer. In this work, we investigate the distribution of these attention weights: How do they change through the decoder layers and through the lifetime of a track? Can they be used to infer additional information about an object, such as a detection uncertainty? Especially in unstructured environments, or environments that were not common during training, a reliable measure of detection uncertainty is crucial to decide whether the system can still be trusted or not.
更多查看译文
关键词
transformer attention spread,tracked objects,uncertainty
AI 理解论文
溯源树
样例
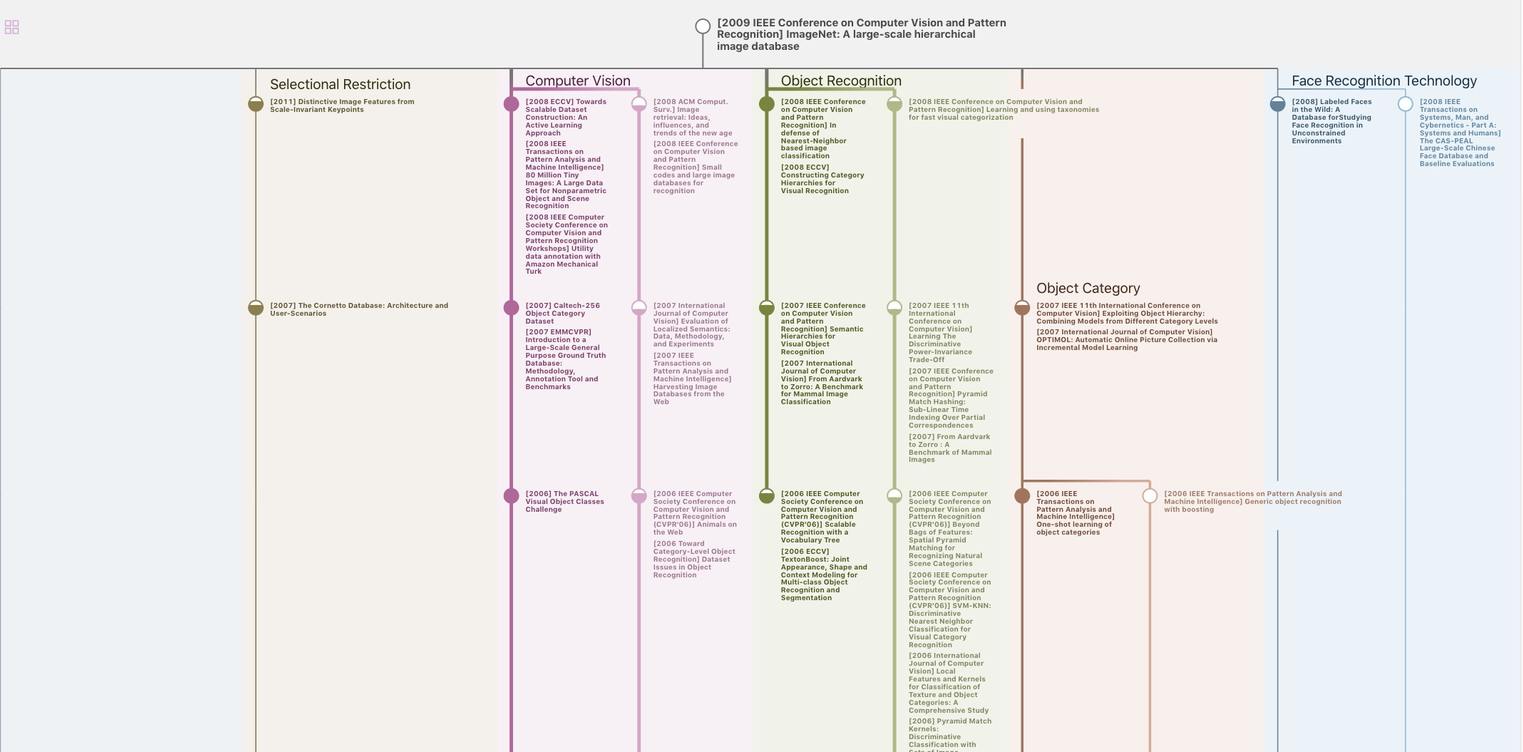
生成溯源树,研究论文发展脉络
Chat Paper
正在生成论文摘要