Adaptive Deep Density Approximation for Fractional Fokker–Planck Equations
arxiv(2023)
摘要
In this work, we propose adaptive deep learning approaches based on normalizing flows for solving fractional Fokker–Planck equations (FPEs). The solution of a FPE is a probability density function (PDF). Traditional mesh-based methods are ineffective because of a unbounded computation domain, a large number of dimensions and a nonlocal fractional operator. To this end, we represent the solution with an explicit PDF model induced by a flow-based deep generative model, which constructs a transport map from a simple distribution to the target distribution. We consider two methods to approximate the fractional Laplacian. One method is the Monte Carlo approximation. The other method is to construct an auxiliary model with Gaussian radial basis functions (GRBFs) to approximate the solution such that we may take advantage of the fact that the fractional Laplacian of a Gaussian is known analytically. Based on these two different ways for the approximation of the fractional Laplacian, we propose two models to approximate stationary FPEs and one model to approximate time-dependent FPEs. To further improve the accuracy, we refine the training set and the approximate solution alternately. A variety of numerical examples is presented to demonstrate the effectiveness of our adaptive deep density approaches.
更多查看译文
关键词
Fractional Fokker–Planck equation,Normalizing flow,Adaptive density approximation,Monte Carlo sampling,Gaussian radial basis functions,65M75,65C30,68T07
AI 理解论文
溯源树
样例
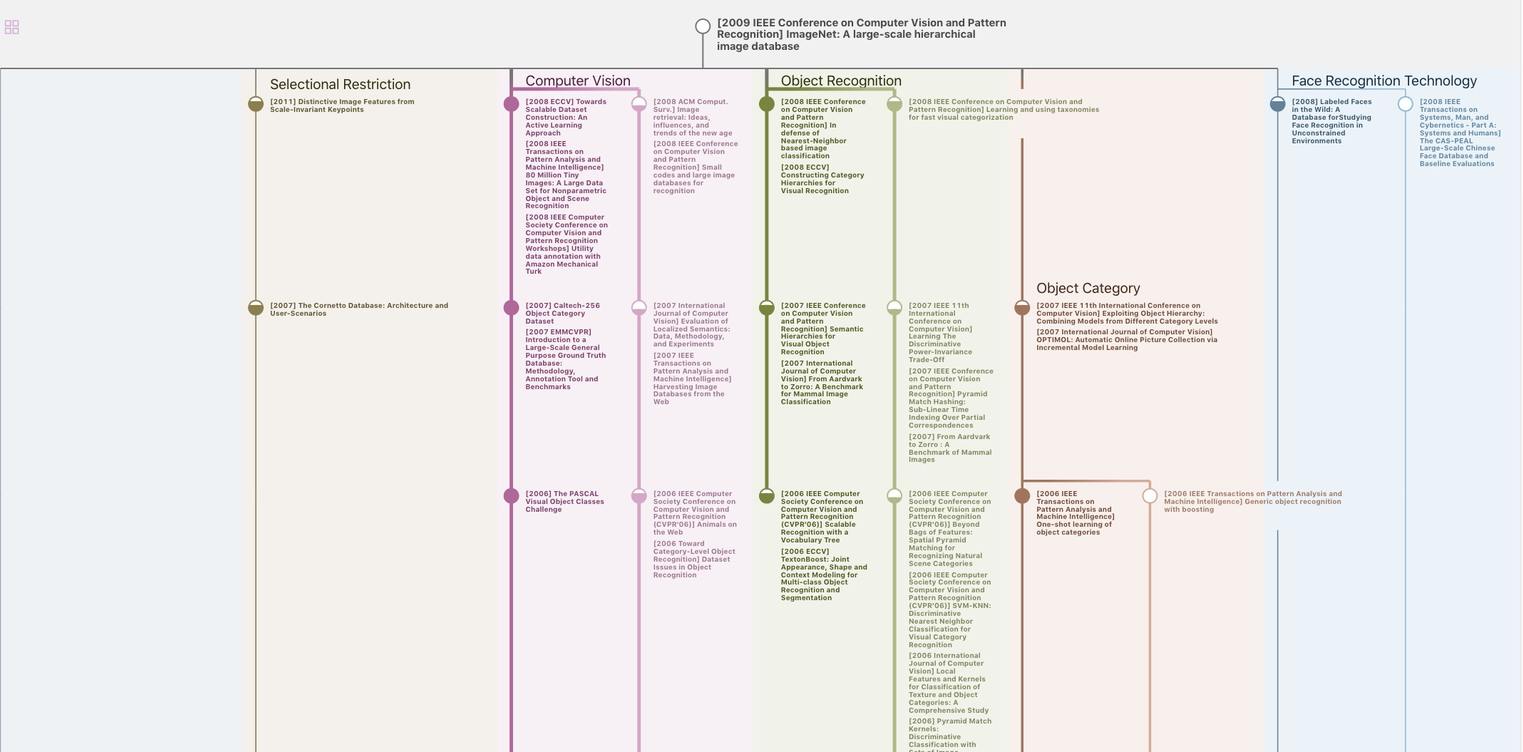
生成溯源树,研究论文发展脉络
Chat Paper
正在生成论文摘要