Hybrid HMM Decoder for Convolutional Codes by Joint Trellis-Like Structure and Channel Prior
IEEE Transactions on Cognitive Communications and Networking(2023)
摘要
The anti-interference capability of wireless links is a physical layer problem for edge computing. Although convolutional codes have inherent error correction potential due to the redundancy introduced in the data, the performance of the convolutional code is drastically degraded due to multipath effects on the channel. In this paper, we propose the use of a Hidden Markov Model (HMM) for the reconstruction of convolutional codes and decoding by the Viterbi algorithm. Furthermore, to implement soft-decision decoding, the observation of HMM is replaced by Gaussian mixture models (GMM). Our method provides superior error correction potential than the standard method because the model parameters contain channel state information (CSI). We evaluated the performance of the method compared to standard Viterbi decoding by numerical simulation. In the multipath channel, the hybrid HMM decoder can achieve a performance gain of 4.7 dB and 2 dB when using hard-decision and soft-decision decoding, respectively. The HMM decoder also achieves significant performance gains for the RSC code, suggesting that the method could be extended to turbo codes. Code can be available at
https://github.com/HaoyyLi/HMM-decoder
.
更多查看译文
关键词
Edge computing,convolutional codes,GMM,hybrid HMM,multipath channels
AI 理解论文
溯源树
样例
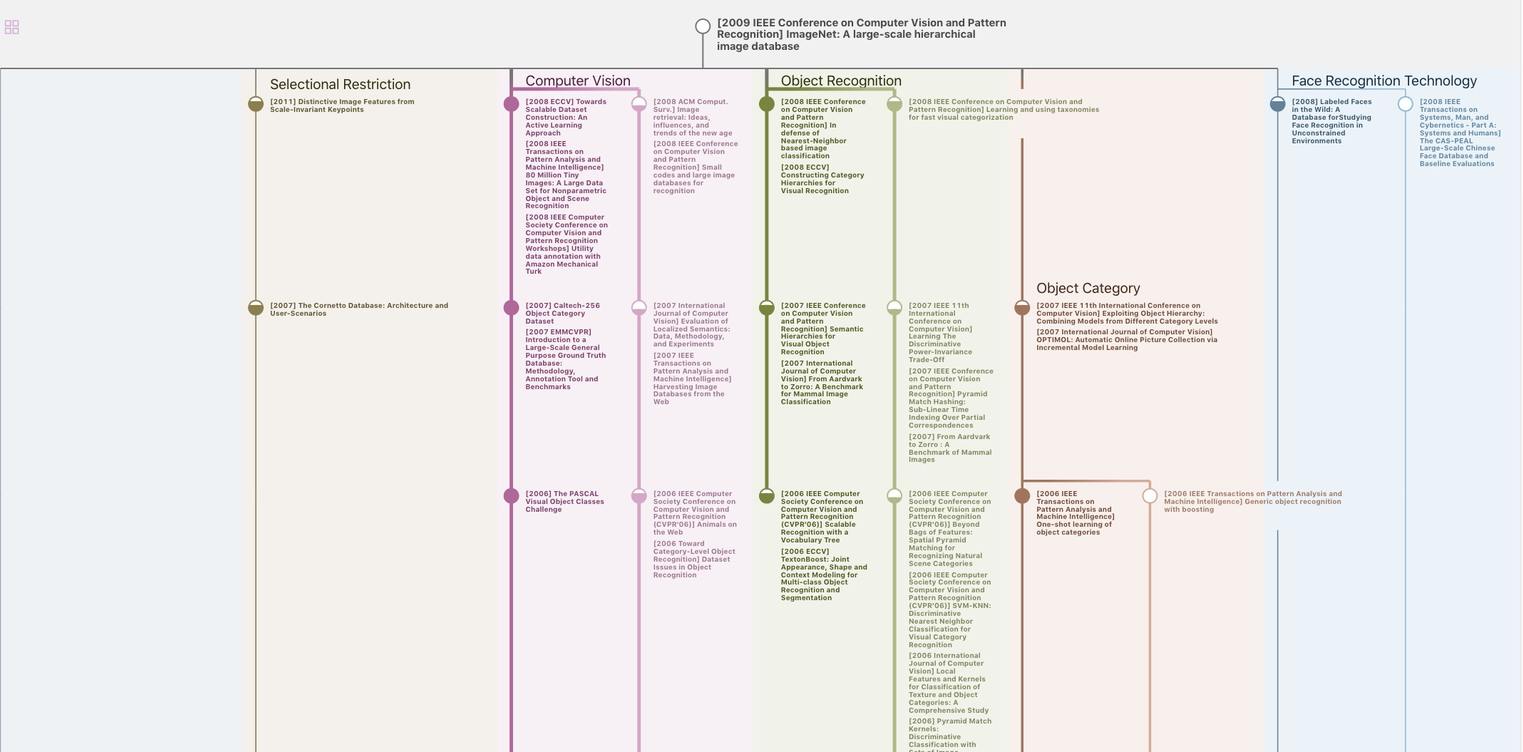
生成溯源树,研究论文发展脉络
Chat Paper
正在生成论文摘要