Enforcing Dirichlet boundary conditions in physics-informed neural networks and variational physics-informed neural networks
HELIYON(2023)
摘要
In this paper, we present and compare four methods to enforce Dirichlet boundary conditions in Physics-Informed Neural Networks (PINNs) and Variational Physics-Informed Neural Networks (VPINNs). Such conditions are usually imposed by adding penalization terms in the loss function and properly choosing the corresponding scaling coefficients; however, in practice, this requires an expensive tuning phase. We show through several numerical tests that modifying the output of the neural network to exactly match the prescribed values leads to more efficient and accurate solvers. The best results are achieved by exactly enforcing the Dirichlet boundary conditions by means of an approximate distance function. We also show that variationally imposing the Dirichlet boundary conditions via Nitsche's method leads to suboptimal solvers.
更多查看译文
关键词
Dirichlet boundary conditions,PINN,VPINN,Deep neural networks,Approximate distance function
AI 理解论文
溯源树
样例
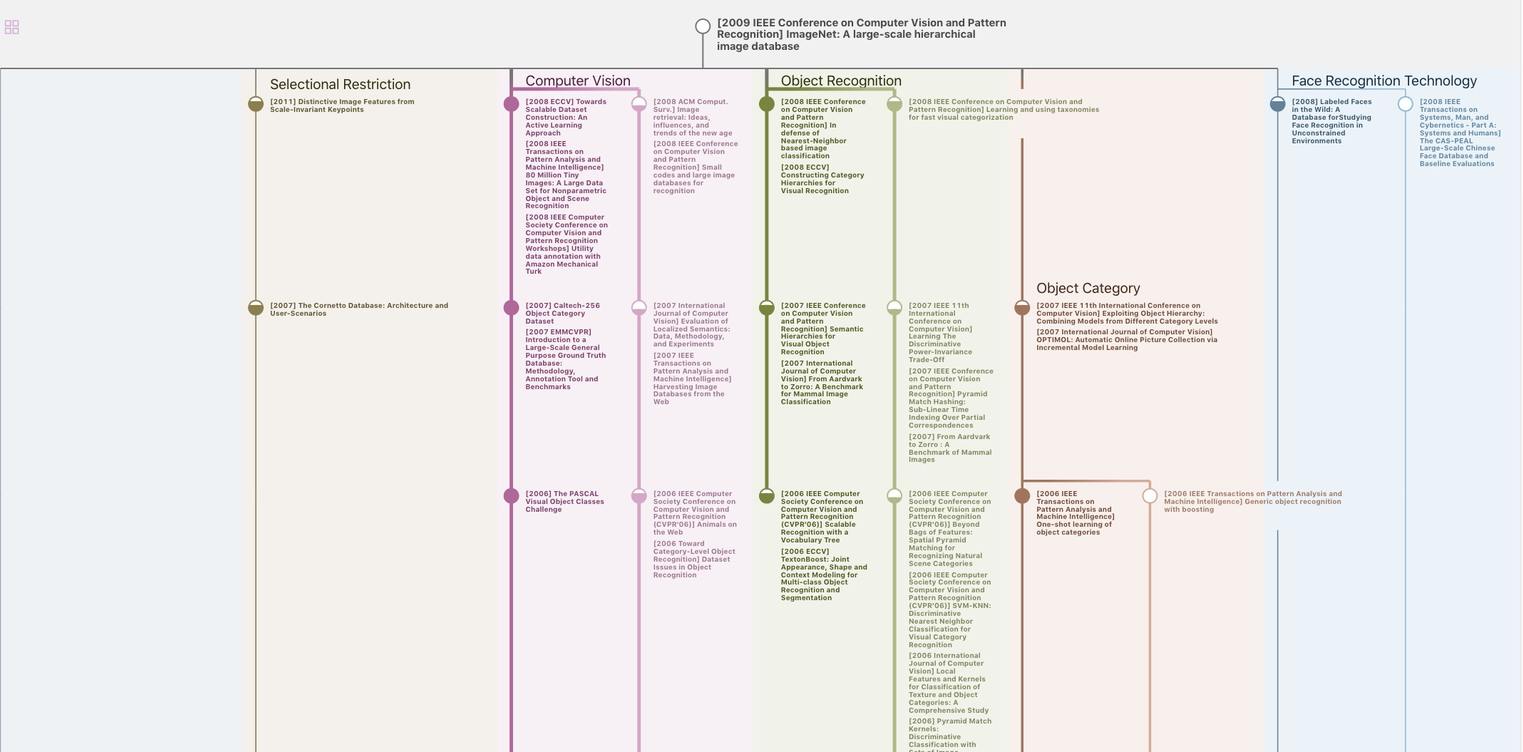
生成溯源树,研究论文发展脉络
Chat Paper
正在生成论文摘要