Predictive modeling of antibiotic eradication therapy success for new-onset Pseudomonas aeruginosa pulmonary infections in children with cystic fibrosis
biorxiv(2022)
摘要
Chronic Pseudomonas aeruginosa (Pa) lung infections are the leading cause of mortality among cystic fibrosis (CF) patients; therefore, the eradication of new-onset Pa lung infections is an important therapeutic goal that can have long-term health benefits. The use of early antibiotic eradication therapy (AET) has been shown to eradicate the majority of new-onset Pa infections, and it is hoped that identifying the underlying basis for AET failure will further improve treatment outcomes. Here we generated random forest machine learning models to predict AET outcomes based on pathogen genomic data. We used a nested cross-validation design, population structure control, and recursive feature selection to improve model performance and showed that incorporating population structure control was crucial for improving model interpretation and generalizability. Our best model, controlling for population structure and using only 30 recursively selected features, had an area under the curve of 0.87 for a holdout test dataset. The top-ranked features were generally associated with motility, adhesion, and biofilm formation.
### Competing Interest Statement
The authors have declared no competing interest.
更多查看译文
关键词
antibiotic eradication therapy success,cystic fibrosis,pulmonary infections,new-onset
AI 理解论文
溯源树
样例
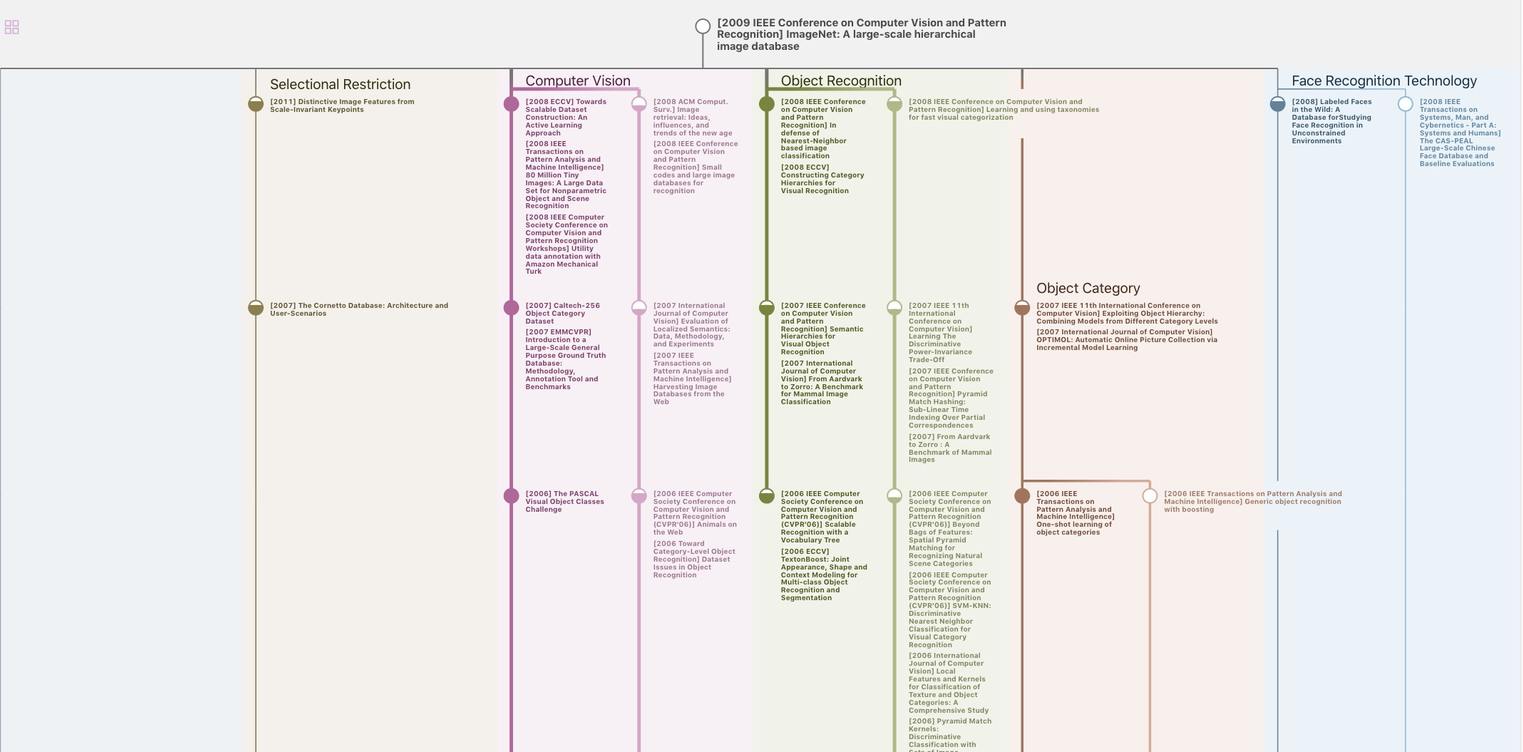
生成溯源树,研究论文发展脉络
Chat Paper
正在生成论文摘要