Hardware-aware Neural Architecture Search with Segmentation-Based Selection.
International Conference on Information and Computer Technologies (ICICT)(2022)
摘要
Hardware-aware Neural Architecture Search (HW-NAS) has been drawing increasing attention since it can auto-matically design deep neural networks optimized in a resource-constrained device. However, it requires enormous amount of computations, which is not affordable for many. Thus, we propose an efficient method for searching promising neural architectures in HW-NAS. We can significantly reduce computing cost of search using both an accuracy predictor and a latency estimator and sharing pre-trained weights of a super-network. Overall searching procedure takes under 1 minute on a single CPU, which is tremendous improvement compared to general NAS work which requires several days or weeks on a single GPU. To search neural architectures under multiple objectives, we propose segmentation-based selection in search stage. The experimental results show our approach is very competitive compared with other multi-objective optimized methods. For a target hardware, we experimented on Field Programmable Gate Array (FPGA) and compared the results with modern CPUs.
更多查看译文
关键词
neural architecture search,segmentation-based se-lection,multi-objective optimization
AI 理解论文
溯源树
样例
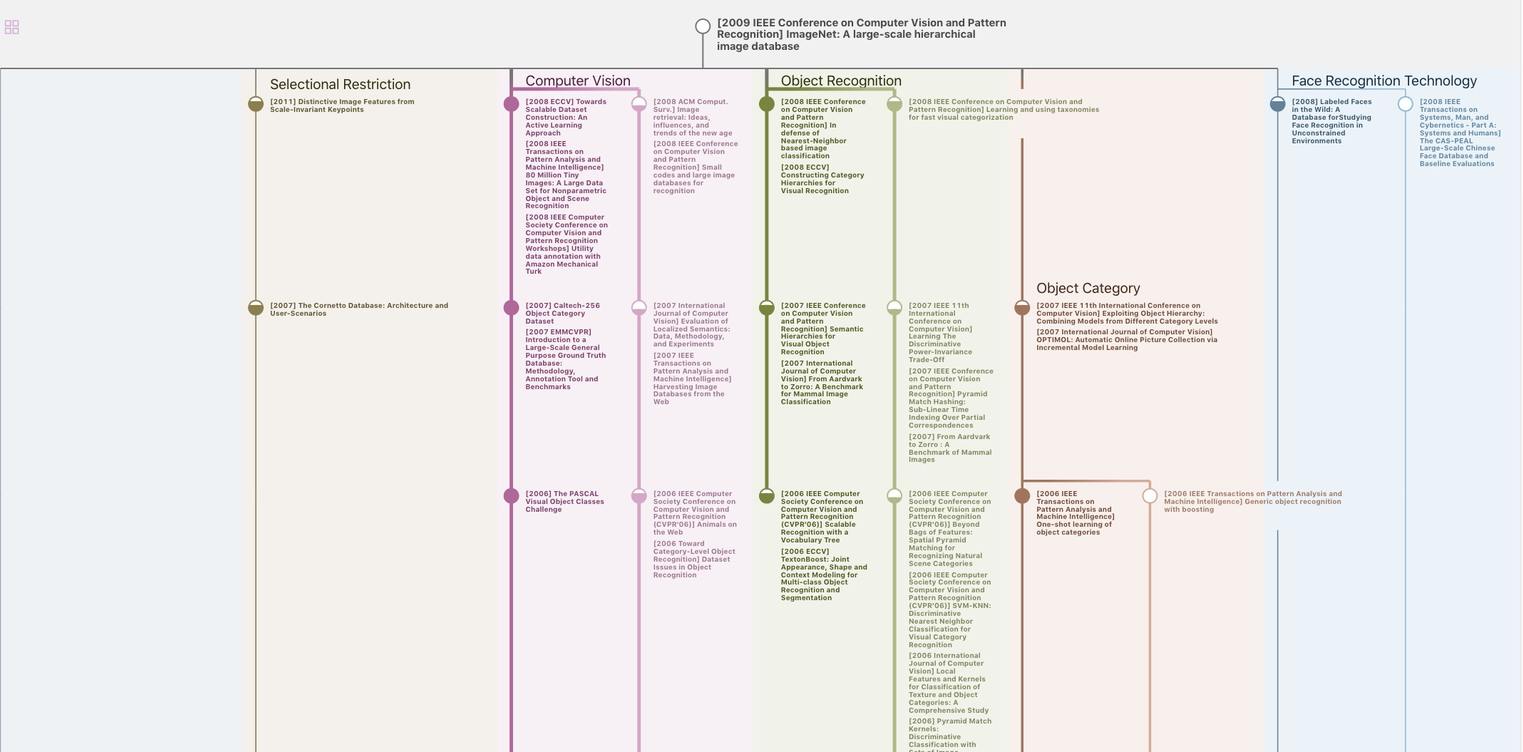
生成溯源树,研究论文发展脉络
Chat Paper
正在生成论文摘要