ReLMole: Molecular Representation Learning Based on Two-Level Graph Similarities
J. Chem. Inf. Model.(2022)
摘要
Molecular representation is a critical part of various prediction tasks for physicochemical properties of molecules and drug design. As graph notations are common in expressing the structural information of chemical compounds, graph neural networks (GNNs) have become the mainstream backbone model for learning molecular representation. However, the scarcity of task-specific labels in the biomedical domain limits the power of GNNs. Recently, self-supervised pretraining for GNNs has been leveraged to deal with this issue, while the existing pretraining methods are mainly designed for graph data in general domains without considering the specific data properties of molecules. In this paper, we propose a representation learning method for molecular graphs, called ReLMole, which is featured by a hierarchical graph modeling of molecules and a contrastive learning scheme based on two-level graph similarities. We assess the performance of ReLMole on two types of downstream tasks, namely, the prediction of molecular properties (MPs) and drug-drug interaction (DDIs). ReLMole achieves promising results for all the tasks. It outperforms the baseline models by over 2.6% on ROC-AUC averaged across six MP prediction tasks, and it improves the F1 value by 7-18% in DDI prediction for unseen drugs compared with other self-supervised models.
更多查看译文
关键词
molecular representation learning,graph,similarities,two-level
AI 理解论文
溯源树
样例
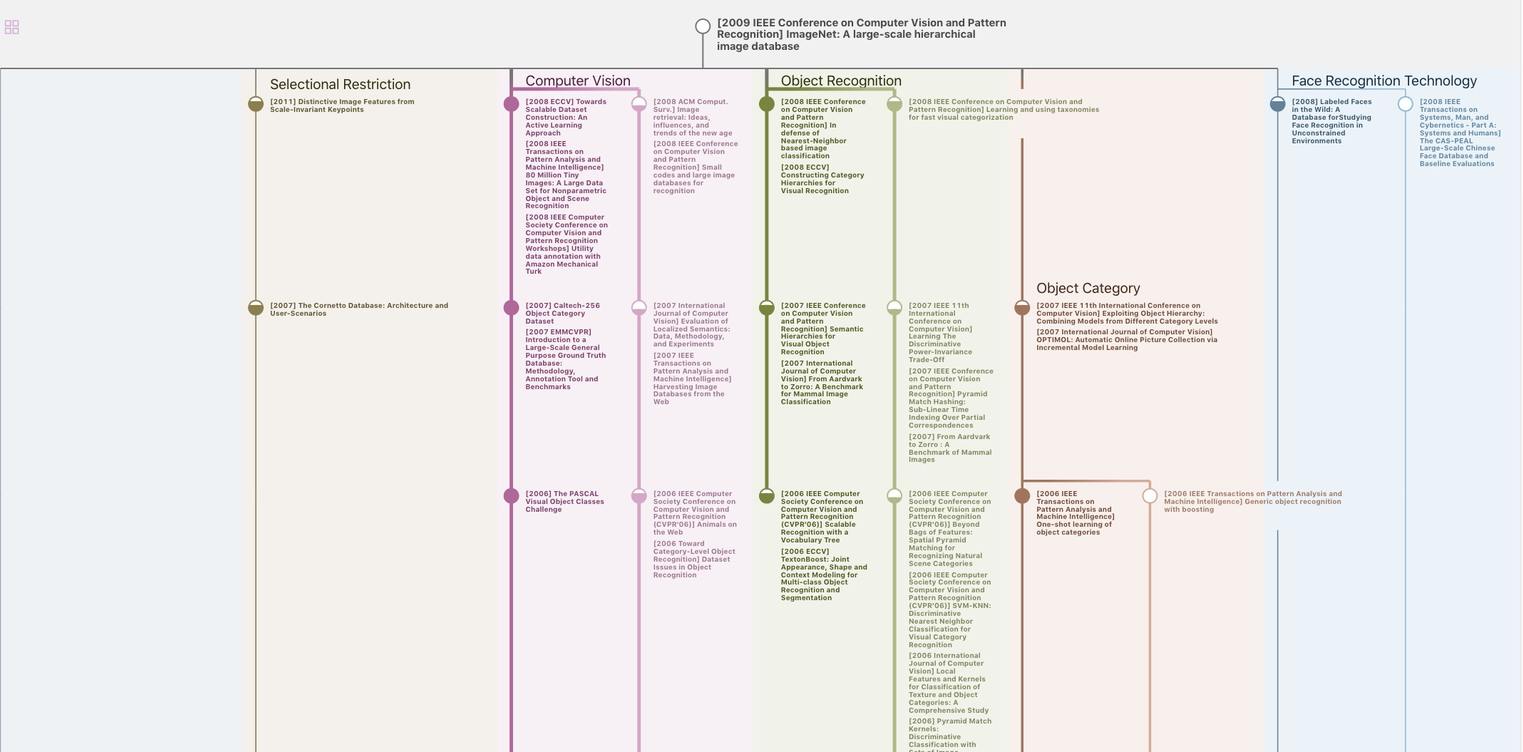
生成溯源树,研究论文发展脉络
Chat Paper
正在生成论文摘要