Detection and Prevention Against Poisoning Attacks in Federated Learning
arxiv(2022)
摘要
This paper proposes and investigates a new approach for detecting and preventing several different types of poisoning attacks from affecting a centralized Federated Learning model via average accuracy deviation detection (AADD). By comparing each client's accuracy to all clients' average accuracy, AADD detect clients with an accuracy deviation. The implementation is further able to blacklist clients that are considered poisoned, securing the global model from being affected by the poisoned nodes. The proposed implementation shows promising results in detecting poisoned clients and preventing the global model's accuracy from deteriorating.
更多查看译文
关键词
federated learning,poisoning attacks,prevention,detection
AI 理解论文
溯源树
样例
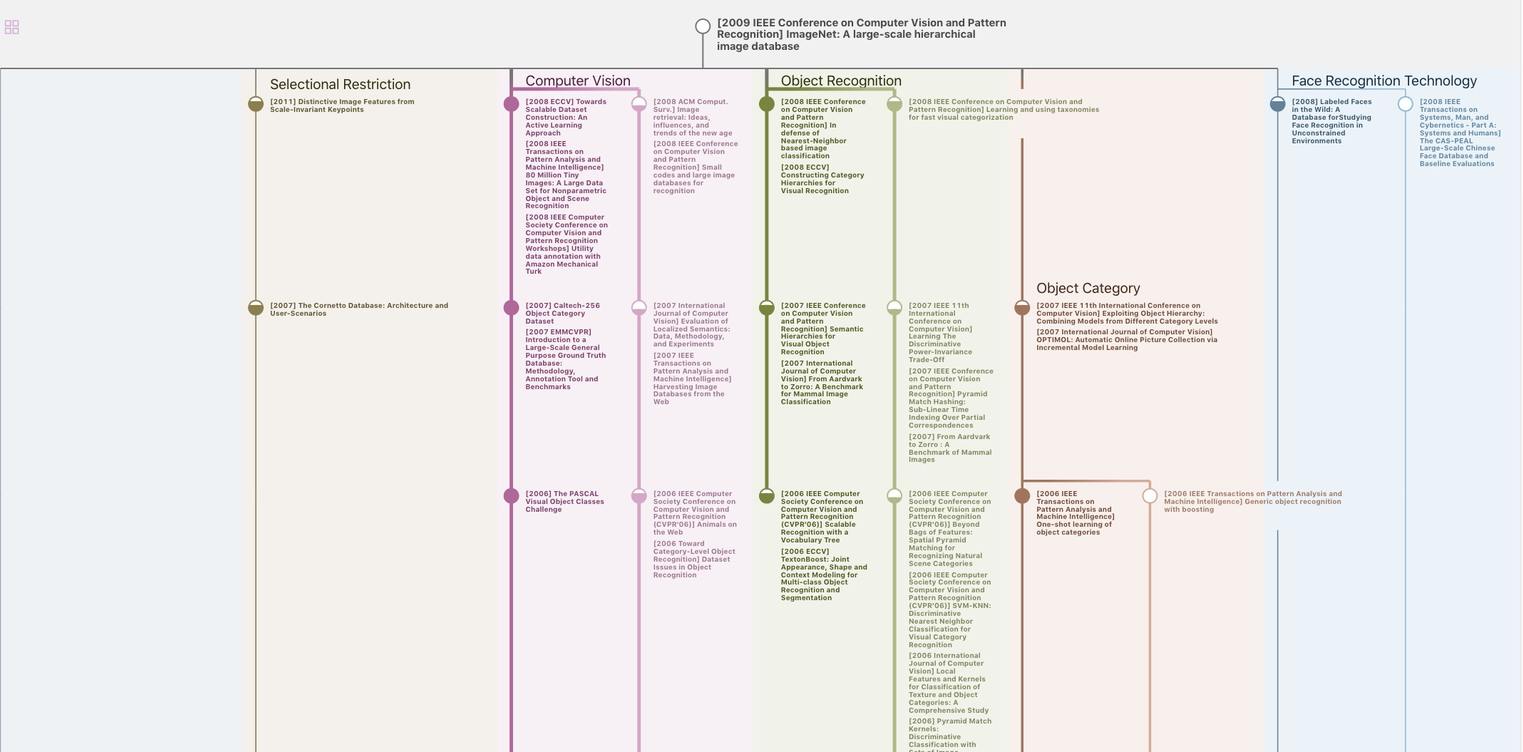
生成溯源树,研究论文发展脉络
Chat Paper
正在生成论文摘要