TRScore: A Novel GPT-based Readability Scorer for ASR Segmentation and Punctuation model evaluation and selection
arXiv (Cornell University)(2022)
摘要
Punctuation and Segmentation are key to readability in Automatic Speech Recognition (ASR), often evaluated using F1 scores that require high-quality human transcripts and do not reflect readability well. Human evaluation is expensive, time-consuming, and suffers from large inter-observer variability, especially in conversational speech devoid of strict grammatical structures. Large pre-trained models capture a notion of grammatical structure. We present TRScore, a novel readability measure using the GPT model to evaluate different segmentation and punctuation systems. We validate our approach with human experts. Additionally, our approach enables quantitative assessment of text post-processing techniques such as capitalization, inverse text normalization (ITN), and disfluency on overall readability, which traditional word error rate (WER) and slot error rate (SER) metrics fail to capture. TRScore is strongly correlated to traditional F1 and human readability scores, with Pearson's correlation coefficients of 0.67 and 0.98, respectively. It also eliminates the need for human transcriptions for model selection.
更多查看译文
关键词
asr segmentation,punctuation model evaluation,readability scorer,gpt-based
AI 理解论文
溯源树
样例
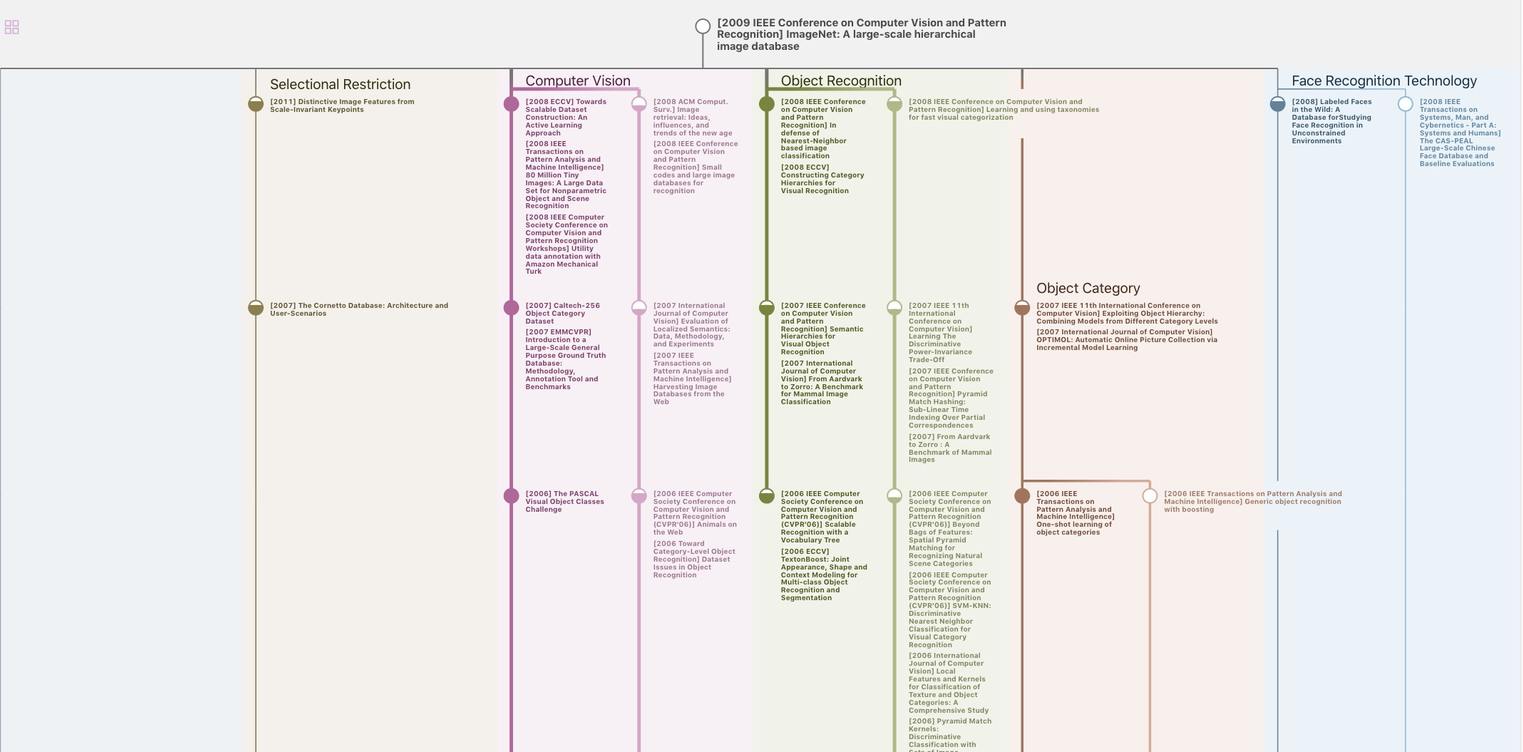
生成溯源树,研究论文发展脉络
Chat Paper
正在生成论文摘要