Cross-modal Transfer Learning Via Multi-grained Alignment for End-to-End Spoken Language Understanding.
Interspeech 2022(2022)
摘要
End-to-end spoken language understanding (E2E-SLU) has witnessed impressive improvements through cross-modal (text-to-audio) transfer learning. However, current methods mostly focus on coarse-grained sequence-level text-to-audio knowledge transfer with simple loss, and neglecting the fine-grained temporal alignment between the two modalities. In this work, we propose a novel multi-grained cross-modal transfer learning framework for E2E-SLU. Specifically, we devise a cross attention module to align the tokens of text with the frame features of speech, encouraging the model to target at the salient acoustic features attended to each token during transferring the semantic information. We also leverage contrastive learning to facilitate cross-modal representation learning in sentence level. Finally, we explore various data augmentation methods to mitigate the deficiency of large amount of labelled data for the training of E2E-SLU. Extensive experiments are conducted on both English and Chinese SLU datasets to verify the effectiveness of our proposed approach. Experimental results and detailed analyses demonstrate the superiority and competitiveness of our model.
更多查看译文
关键词
spoken language understanding,cross-modal transfer learning,cross attention,contrastive learning
AI 理解论文
溯源树
样例
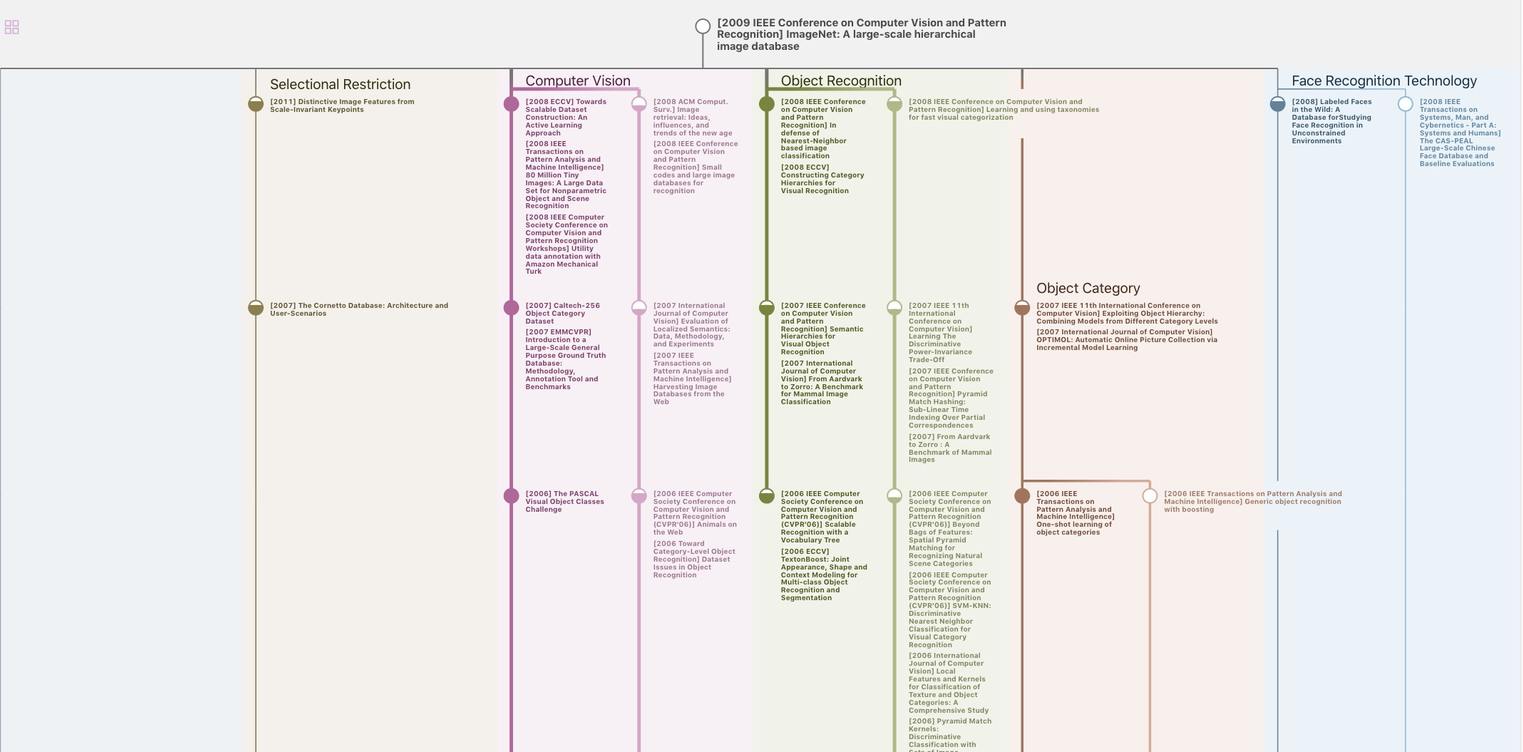
生成溯源树,研究论文发展脉络
Chat Paper
正在生成论文摘要