Contrastive Heartbeats: Contrastive Learning for Self-Supervised ECG Representation and Phenotyping.
IEEE International Conference on Acoustics, Speech, and Signal Processing (ICASSP)(2022)
摘要
The non-invasive and easily accessible characteristics of electrocardiogram (ECG) attract many studies targeting AI-enabled cardiovascular-related disease screening tools based on ECG. However, the high cost of manual labels makes high-performance deep learning models challenging to obtain. Hence, we propose a new self-supervised representation learning framework, contrastive heartbeats (CT-HB), which learns general and robust electrocardiogram representations for efficient training on various downstream tasks. We employ a novel heartbeat sampling method to define positive and negative pairs of heartbeats for contrastive learning by utilizing the periodic and meaningful patterns of electrocardiogram signals. Using the CT-HB framework, the self-supervised learning model learns personalized heartbeat representations representing the specific cardiology context of a patient. Evaluations on public benchmark datasets and a private large-scale real-world dataset with multiple tasks demonstrate that the learned semantic representations result in better performance on downstream tasks and retain high performance while supervised learning suffers performance degradation with fewer supervised labels in downstream tasks.
更多查看译文
关键词
Electrocardiogram,Self-supervised learning,Representation learning,Contrastive learning
AI 理解论文
溯源树
样例
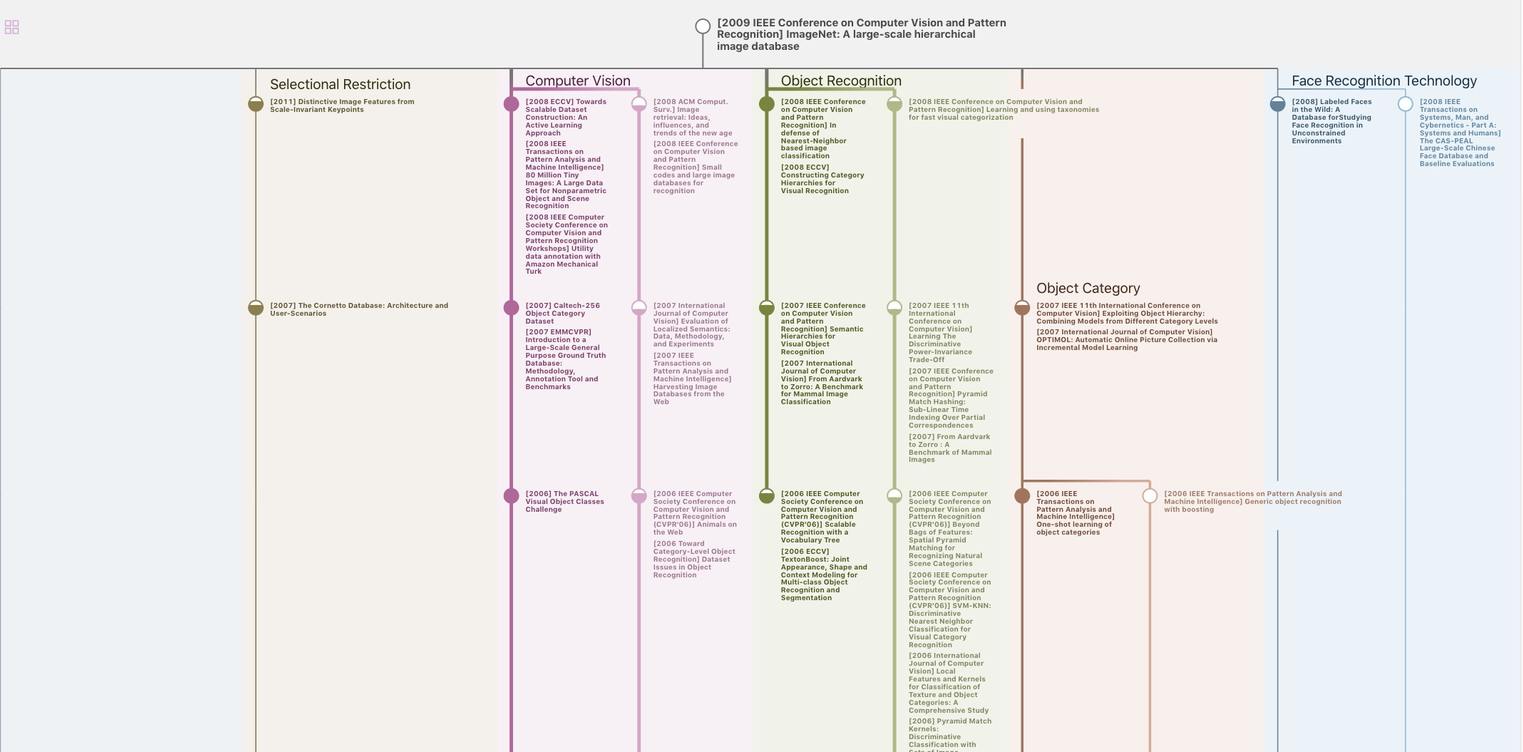
生成溯源树,研究论文发展脉络
Chat Paper
正在生成论文摘要