Dual-Domain Low-Rank Fusion Deep Metric Learning for Off-the-Person ECG Biometrics.
IEEE International Conference on Acoustics, Speech, and Signal Processing (ICASSP)(2022)
摘要
Electrocardiogram (ECG) biometrics has been an emerging field, and off-the-person ECG biometrics capturing the ECG from fingertips is one of the new trends in this field. However, dynamic morphological variability in the same person and low signal-to-noise ratios pose great challenges for off-the-person ECG biometrics. To reduce the dynamic morphological variability, this paper introduces deep metric learning into ECG biometrics to learn intra-individual compact features. To enforce the robust of proposed method, dual-domain features extracted from both 1D signals and 2D spectrograms are integrated by low-rank fusion. Furthermore, this method dispenses with the need for noise removal and outliers discarding completely. Experiments on two off-the-person ECG benchmark databases demonstrate that the proposed method significantly outperforms the state-of-the-art methods. Additionally, ablation experiments show the effectiveness of every part of our framework.
更多查看译文
关键词
ECG biometrics,off-the-person,deep metric learning,dual-domain,low-rank fusion
AI 理解论文
溯源树
样例
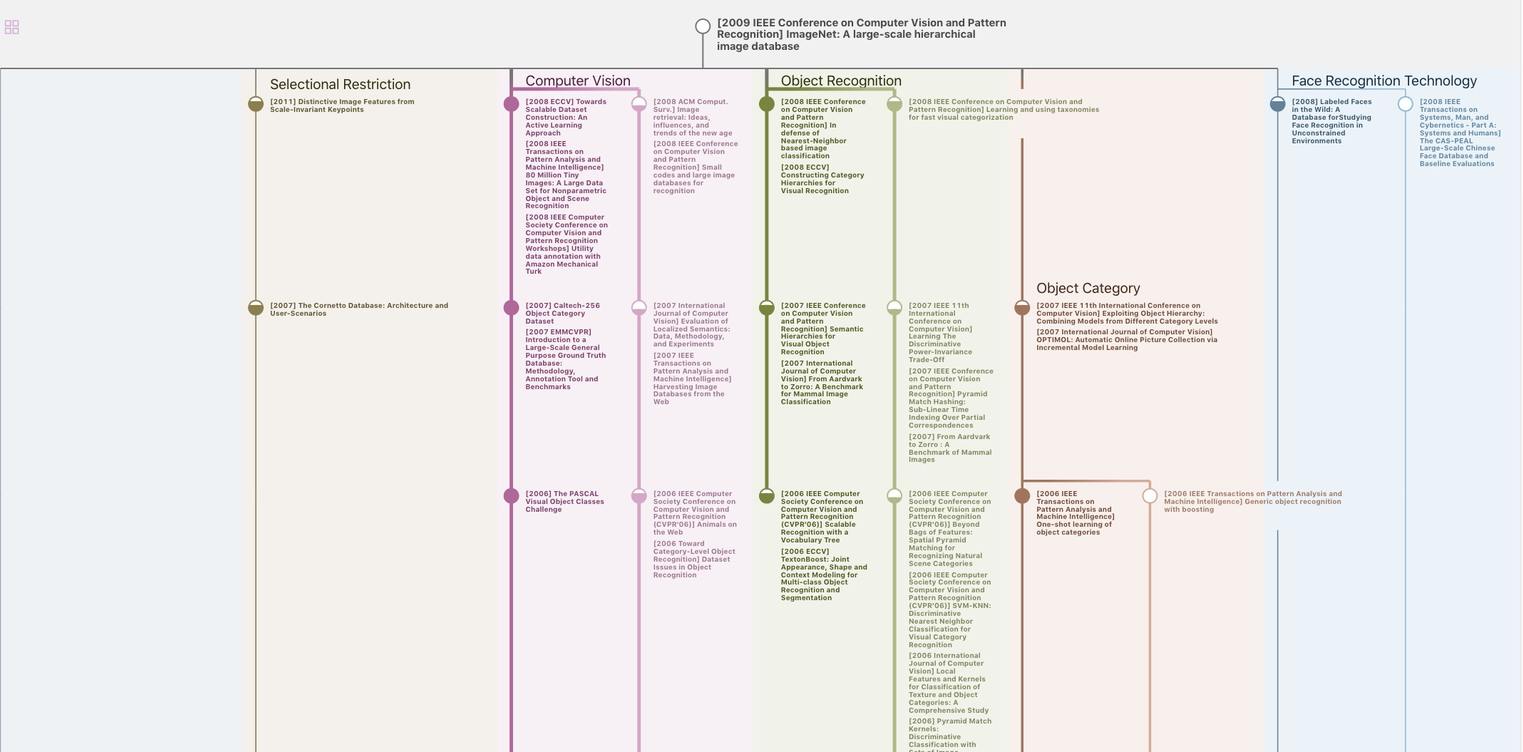
生成溯源树,研究论文发展脉络
Chat Paper
正在生成论文摘要