Simulation-and-mining: towards accurate source-free unsupervised domain adaptive object detection
IEEE International Conference on Acoustics, Speech, and Signal Processing (ICASSP)(2022)
摘要
Vanilla unsupervised domain adaptive (UDA) object detection typically requires the labeled source data for joint-training with the unlabeled target data, which is usually unavailable in real-world scenarios due to data privacy, leading to source data-free UDA object detection. Herein, we first analyze the phenomenon of cross-domain detection degradation varying from easy to hard samples (e.g. the objects with different scales or occlusion degrees), termed as domain generalization differentiation. In detail, the ability to detect easy samples is well transferred while the one to detect hard samples is dramatically degraded. To this end, we then revisit the existing self-training method, which is of great challenge to deal with the abundant false negatives (hard samples). Assumed that true positives (easy samples) labeled by the source model can be exploited as supervision cues. UDA is finally modeled into an unsupervised false negatives mining problem. Thus, we propose a Simulation-and-Mining (S&M) framework, which simulates false negatives by augmenting true positives and mines back false negatives alternatively and iteratively. Experimental results show the effectiveness.
更多查看译文
关键词
Domain Adaptation,Self-Training,Object Detection,Domain Generalization Differentiation
AI 理解论文
溯源树
样例
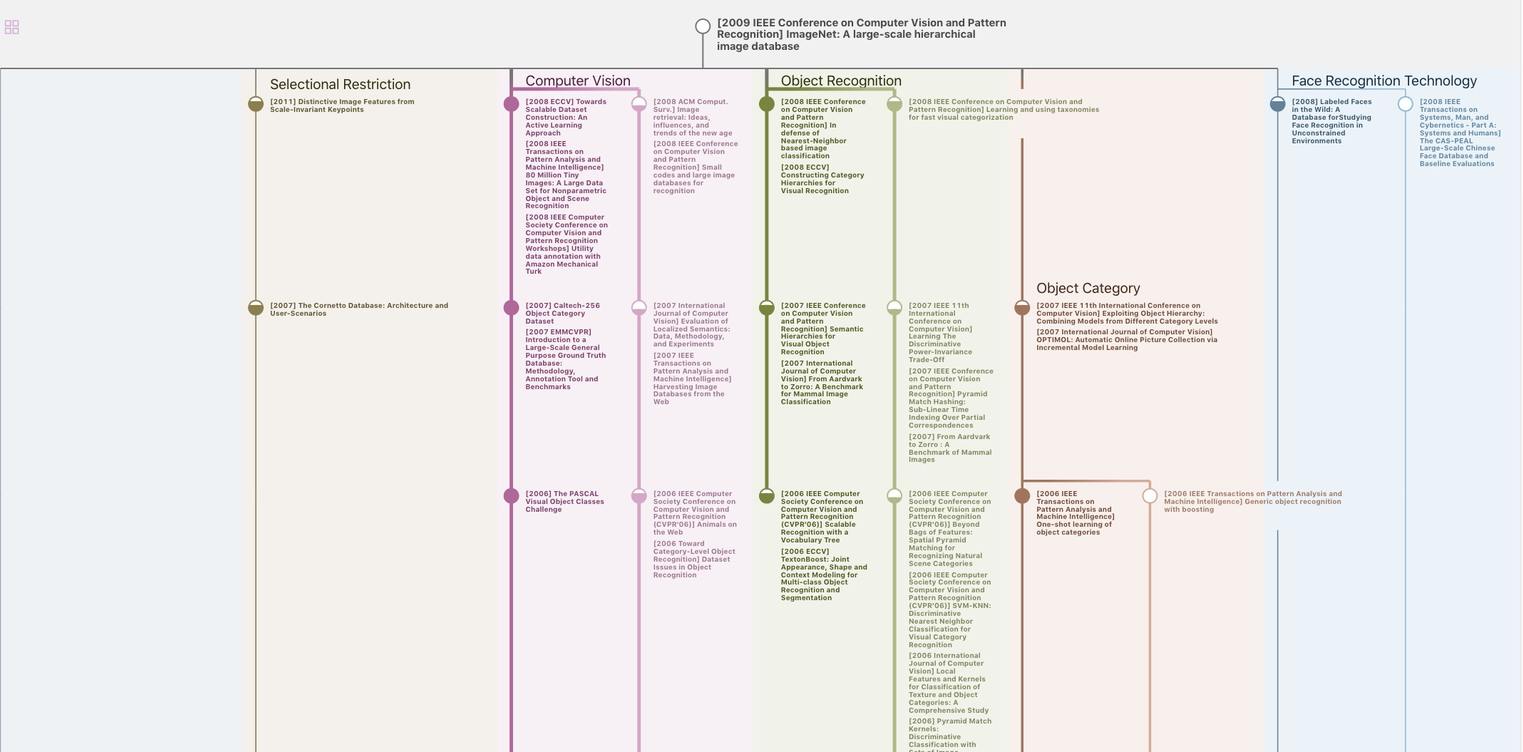
生成溯源树,研究论文发展脉络
Chat Paper
正在生成论文摘要