A Gaussian Mixture Model for Dialogue Generation with Dynamic Parameter Sharing Strategy.
IEEE International Conference on Acoustics, Speech, and Signal Processing (ICASSP)(2022)
摘要
Existing dialog models are trained with data in an encoder-decoder framework with the same parameters, ignoring the multinomial distribution nature in the dataset. In fact, model improvement and development commonly requires fine-grained modeling on individual data subsets. However, collecting a labeled fine-grained dialogue dataset often requires expert-level domain knowledge and therefore is difficult to scale in the real world. As we focus on better modeling multinomial data for dialog generation, we study an approach that combines the unsupervised clustering and generative model together with a GMM (Gaussian Mixture Model) based encoder-decoder framework. Specifically, our model samples from the prior and recognition distributions over the latent variables by a Gaussian mixture network and the latent layer with the capability to form multiple clusters. We also introduce knowledge distillation to guide and improve the clustering results. Finally, we use a dynamic parameter sharing strategy conditioned on different labels to train different decoders. Experimental results on a widely used dialogue dataset verify the effectiveness of the proposed method.
更多查看译文
关键词
dialogue generation,cluster,GMM,knowledge distill,dynamic parameter sharing strategy
AI 理解论文
溯源树
样例
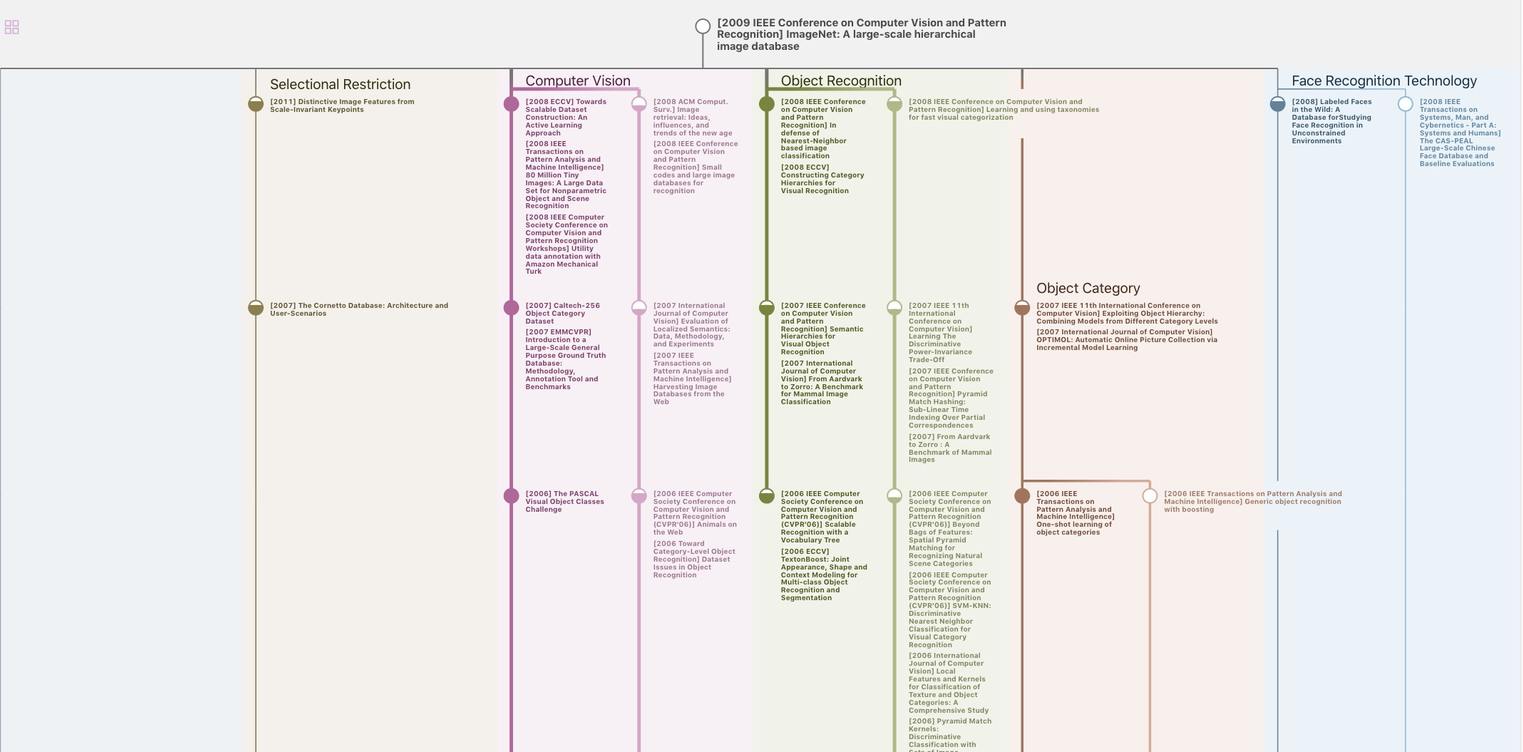
生成溯源树,研究论文发展脉络
Chat Paper
正在生成论文摘要