Resting-State Functional MRI Adaptation with Attention Graph Convolution Network for Brain Disorder Identification
BRAIN SCIENCES(2022)
摘要
Multi-site resting-state functional magnetic resonance imaging (rs-fMRI) data can facilitate learning-based approaches to train reliable models on more data. However, significant data heterogeneity between imaging sites, caused by different scanners or protocols, can negatively impact the generalization ability of learned models. In addition, previous studies have shown that graph convolution neural networks (GCNs) are effective in mining fMRI biomarkers. However, they generally ignore the potentially different contributions of brain regions- of-interest (ROIs) to automated disease diagnosis/prognosis. In this work, we propose a multi-site rs-fMRI adaptation framework with attention GCN (A(2)GCN) for brain disorder identification. Specifically, the proposed A(2)GCN consists of three major components: (1) a node representation learning module based on GCN to extract rs-fMRI features from functional connectivity networks, (2) a node attention mechanism module to capture the contributions of ROIs, and (3) a domain adaptation module to alleviate the differences in data distribution between sites through the constraint of mean absolute error and covariance. The A(2)GCN not only reduces data heterogeneity across sites, but also improves the interpretability of the learning algorithm by exploring important ROIs. Experimental results on the public ABIDE database demonstrate that our method achieves remarkable performance in fMRI-based recognition of autism spectrum disorders.
更多查看译文
关键词
domain adaptation, multi-site data, graph convolutional networks, autism, resting-state functional MRI
AI 理解论文
溯源树
样例
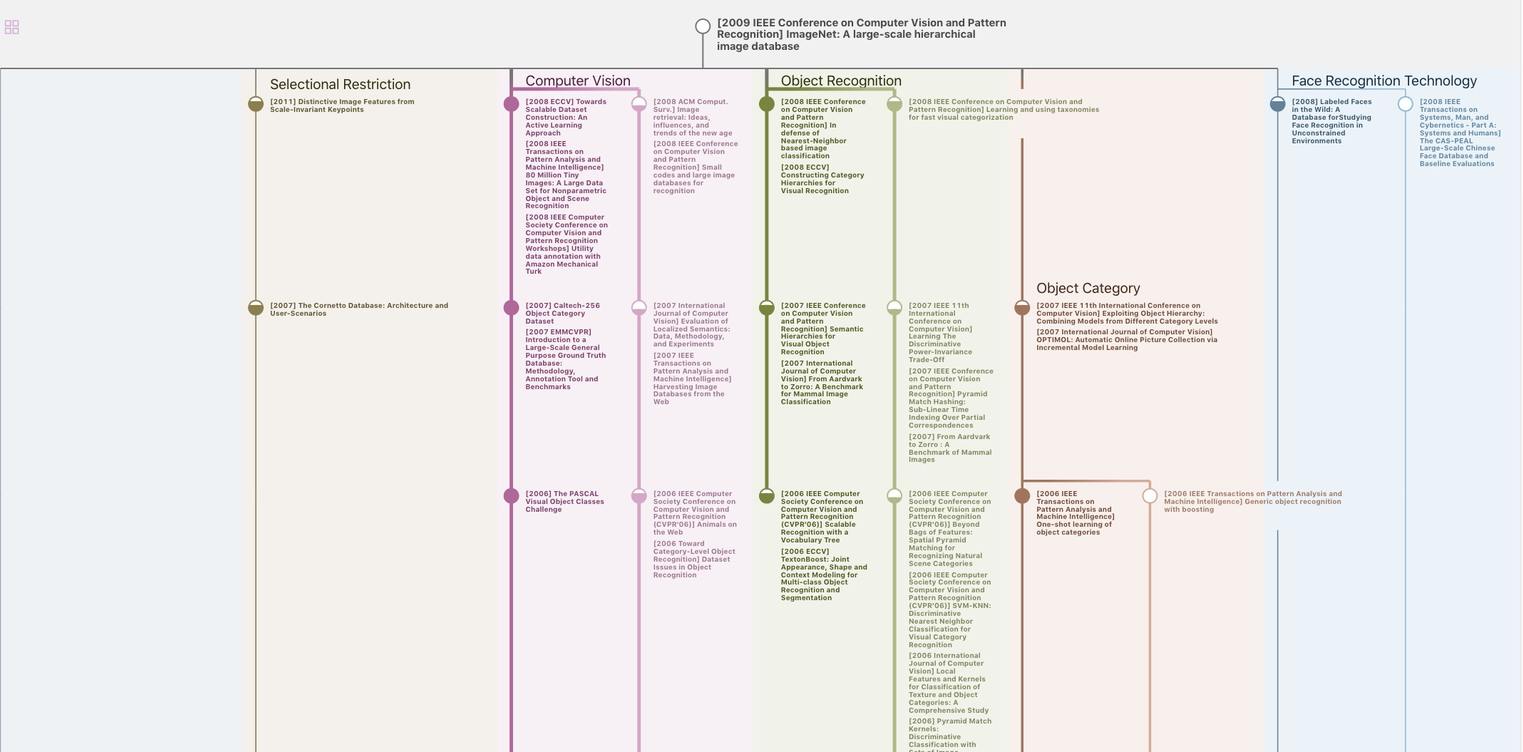
生成溯源树,研究论文发展脉络
Chat Paper
正在生成论文摘要