PD-BertEDL: an Ensemble Deep Learning Method Using BERT and Multivariate Representation to Predict Peptide Detectability
International Journal of Molecular Sciences(2022)
关键词
peptide detectability,BERT,multivariate representation,ensemble deep learning
AI 理解论文
溯源树
样例
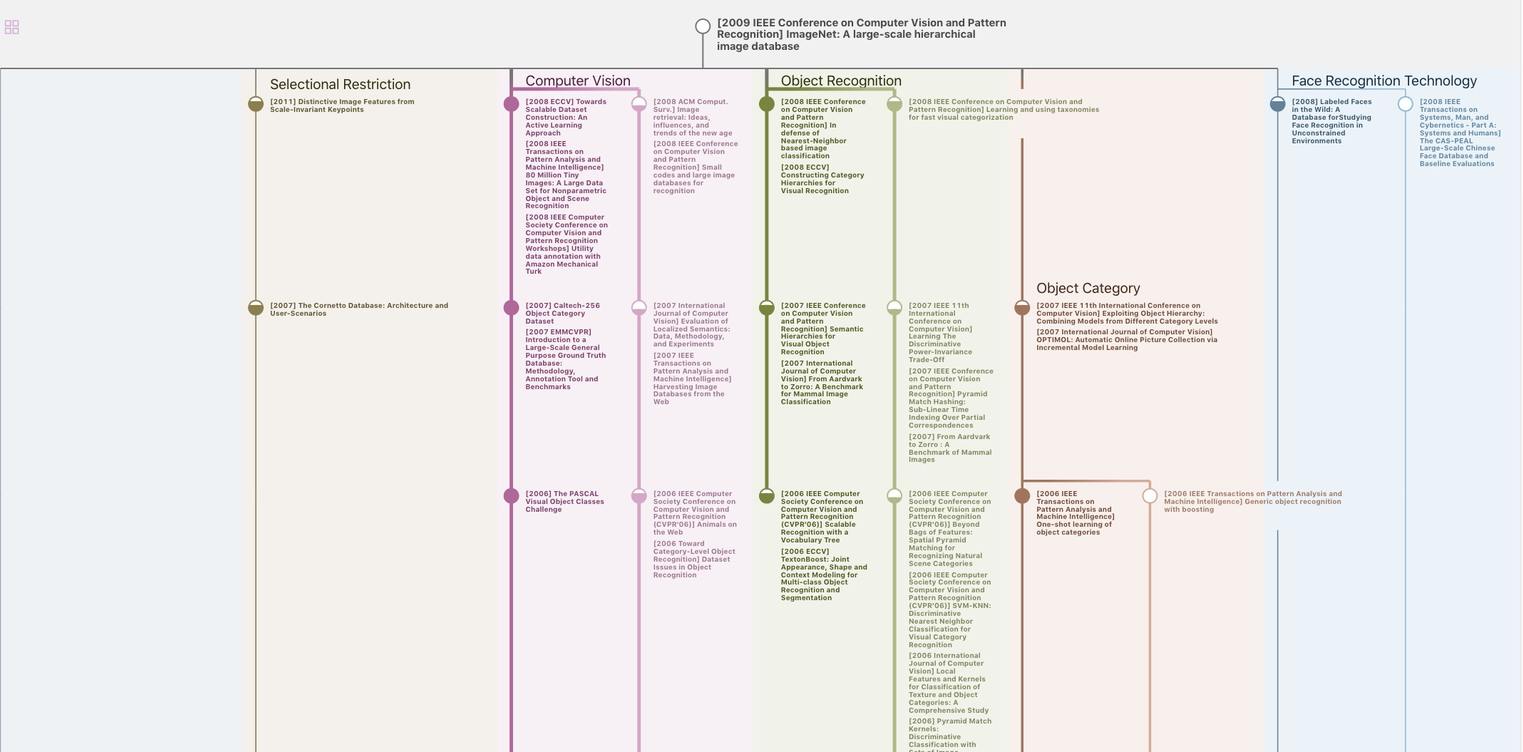
生成溯源树,研究论文发展脉络
Chat Paper
正在生成论文摘要