Understanding the Determinants for Predicting Citizens’ Travel Mode Change from Private Cars to Public Transport in China
Frontiers in psychology(2022)
摘要
Rapid urbanization and motorization have generated increasing social and environmental challenges to the urban transport sector in China, such as traffic congestion, car accidents, air pollution, and global warming. Prioritizing the development of urban public transport system has been adopted as a primary strategy by Chinese government. However, the problems caused by large numbers of private cars are still far from being solved, and the ridership of public transport in China is relatively low. Therefore, the current study proposes a new comprehensive framework by enhancing the norm activation model (NAM) and theory of planned behavior (TPB) with the perceived accessibility to public transport, to better understand the determinants for predicting citizens’ choice of public transport as a sustainable travel mode. An online survey concerning travel mode change was conducted among citizens in Hangzhou, China. Based on 341 valid samples, partial least squares structural equation modeling (PLS-SEM) was employed to verify the proposed framework. The analytical results confirm that awareness of consequences and subjective norms are the two key constructs for connecting the two theories as a whole. Further, the examination of intention-behavior relationship shows that perceived accessibility to public transport could strengthen the relationship between behavioral intention and actual behavior in using public transport. The findings not only contribute to the development of pro-environmental theories, but also have meaningful implications for governments to develop relevant policies to encourage citizens to use public transport as a sustainable travel mode.
更多查看译文
关键词
travel mode change,private cars,public transport,norm activation model,theory of planned behavior,perceived accessibility
AI 理解论文
溯源树
样例
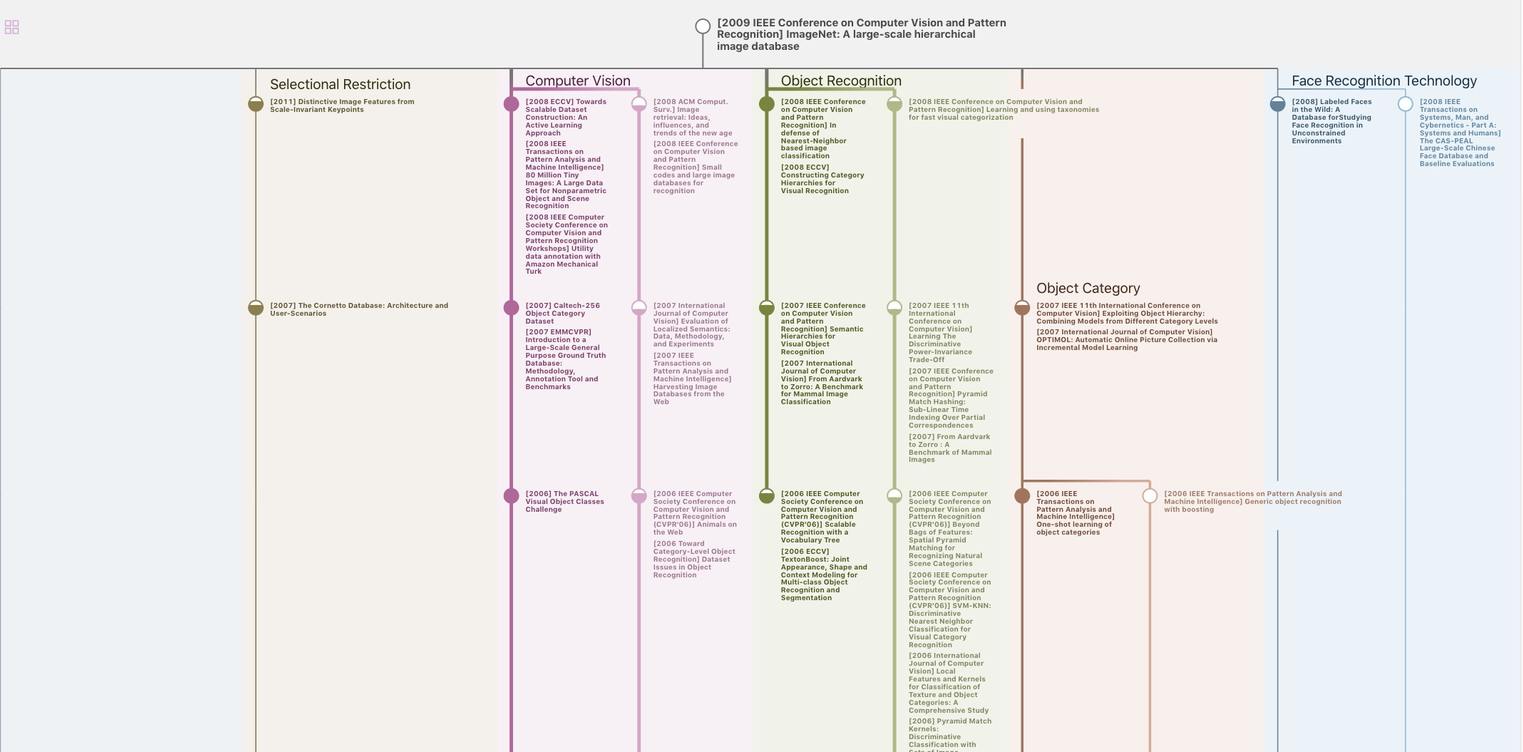
生成溯源树,研究论文发展脉络
Chat Paper
正在生成论文摘要