In-situ Data Curation: A Key To Actionable AI at the Edge
ACM International Conference on Mobile Computing and Networking(2022)
摘要
Machine learning (ML) algorithms have shown great potential in edge-computing environments, however, the literature mainly focuses on model inference only. We investigate how ML can be operationalized and how in-situ curation can improve the quality of edge applications, in the context of ML-assisted environmental surveys. We show that camera-enabled ML systems deployed on edge devices can enable scientists to perform real-time monitoring of species of interest or characterization of natural habitats. However, the benefit of this new technology is only as good as the quality and accuracy of the edge ML model inferences. In this demonstration, we show that with small additional time investment, domain scientists can manually curate ML model outputs and thus obtain highly reliable scientific insights, leading to more effective and scalable environmental surveys.
更多查看译文
关键词
Edge compute, Deep Neural networks, Interactive Learning
AI 理解论文
溯源树
样例
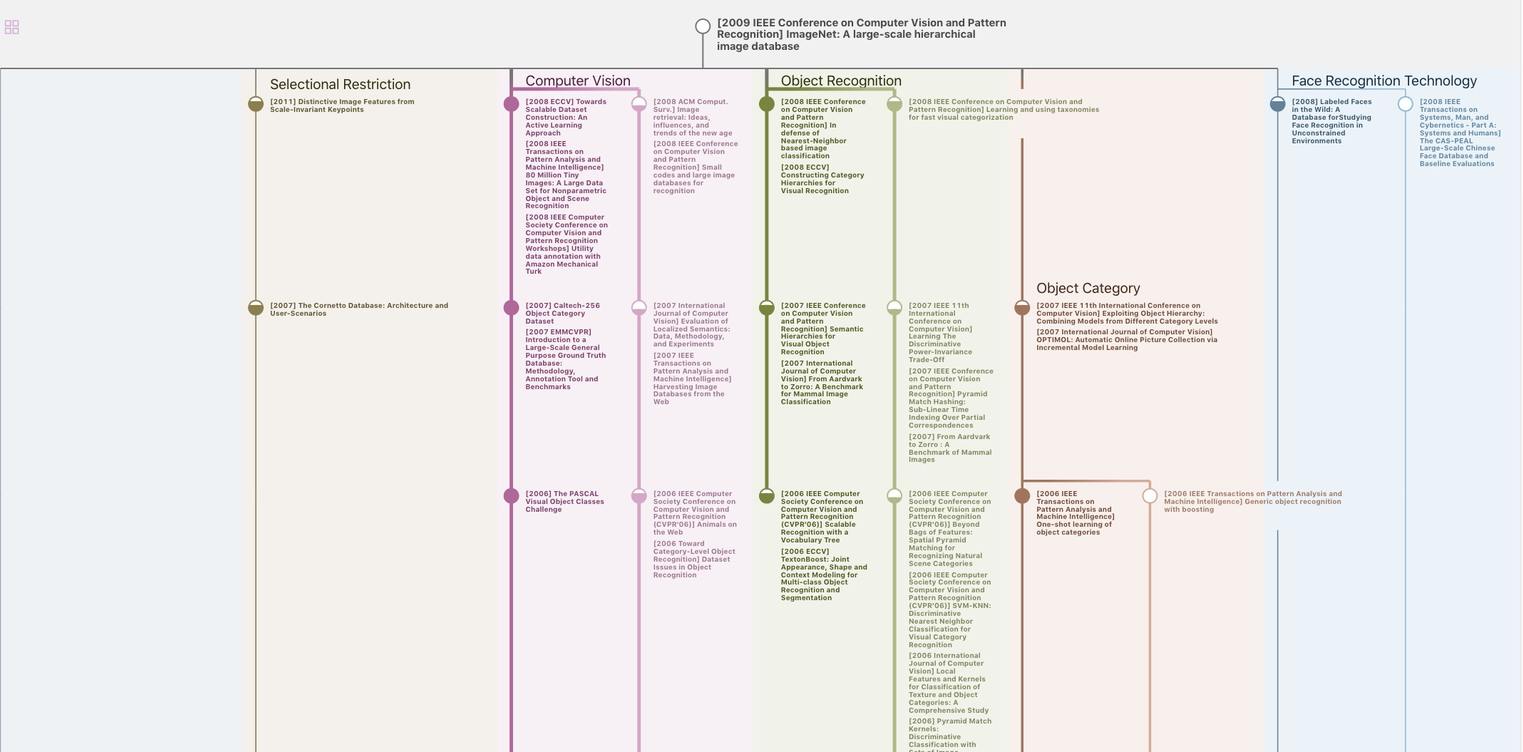
生成溯源树,研究论文发展脉络
Chat Paper
正在生成论文摘要