Forecasting Undergraduate Majors: A Natural Language Approach
AERA Open(2022)
摘要
Committing to a major is a fateful step in an undergraduate education, yet the relationship between courses taken early in an academic career and ultimate major issuance remains little studied at scale. Using transcript data capturing the academic careers of 26,892 undergraduates enrolled at a private university between 2000 and 2020, we describe enrollment histories by using natural-language methods and vector embeddings to forecast terminal major on the basis of course sequences beginning at college entry. We find that (a) a student’s very first enrolled course predicts their major 30 times better than random guessing and more than one-third better than majority-class voting, (b) modeling strategies substantially influence forecasting metrics, and (c) course portfolios vary substantially within majors, such that students with the same major exhibit relatively modest overlap.
更多查看译文
关键词
colleges,decision-making,degree planning,descriptive analysis,higher education,information retrieval,institutional research,Jaccardian similarity,LASSO,network analysis,NLP,observational research,postsecondary education,predictive analytics,regression analyses,textual analysis,word embedding
AI 理解论文
溯源树
样例
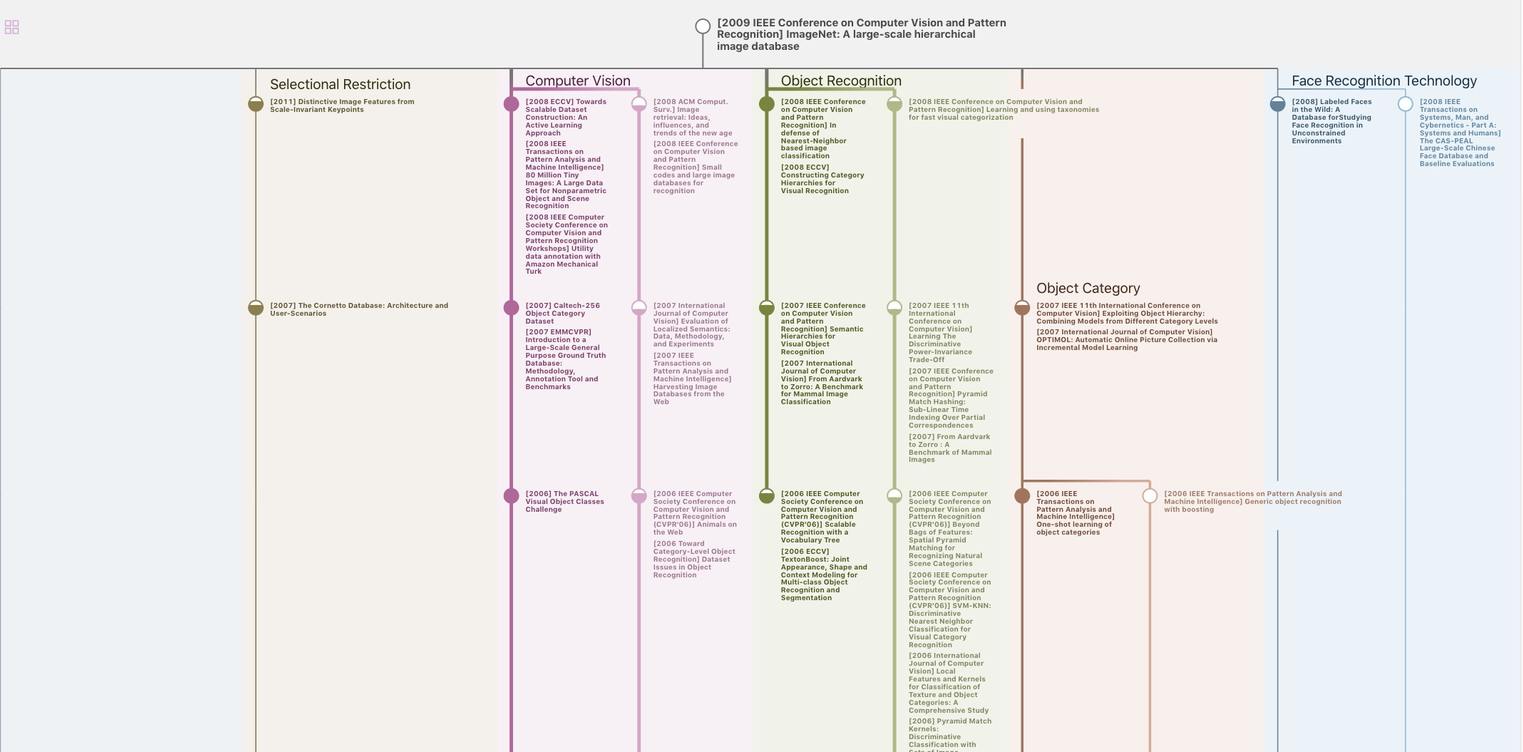
生成溯源树,研究论文发展脉络
Chat Paper
正在生成论文摘要