Graph augmented sequence-to-sequence model for neural question generation
Applied Intelligence(2022)
摘要
Neural question generation (NQG) aims to generate a question from a given passage with neural networks. NQG has attracted more attention in recent years, due to its wide applications in reading comprehension, question answering, and dialogue systems. Existing works on NQG mainly use the sequence-to-sequence (Seq2Seq) or graph-to-sequence (Graph2Seq) framework. The former ignores rich structure information of the passage, while the latter is insufficient in modeling semantic information. Moreover, the target answer plays an important role in the task, because without the answer the generated question has great randomness. To effectively utilize answer information and capture both structure and semantic information of the passage, we propose a graph augmented sequence-to-sequence (GA-Seq2Seq) model. Firstly, we design an answer-aware passage representation module to integrate the answer information into the passage. Then, to discover both the structure and semantic information of the passage, we present a graph augmented passage encoder which consists of a graph encoder and a sequence encoder. Finally, we leverage an attention-based long short-term memory decoder to generate the question. Experimental results on the SQuAD and MS MARCO datasets show that our proposed model outperforms the existing state-of-the-art baselines in terms of automatic and human evaluations. The implementation is available at https://github.com/butterfliesss/GA-Seq2Seq .
更多查看译文
关键词
Question generation,Sequence-to-sequence,Graph neural network,Recurrent neural network,Answer information
AI 理解论文
溯源树
样例
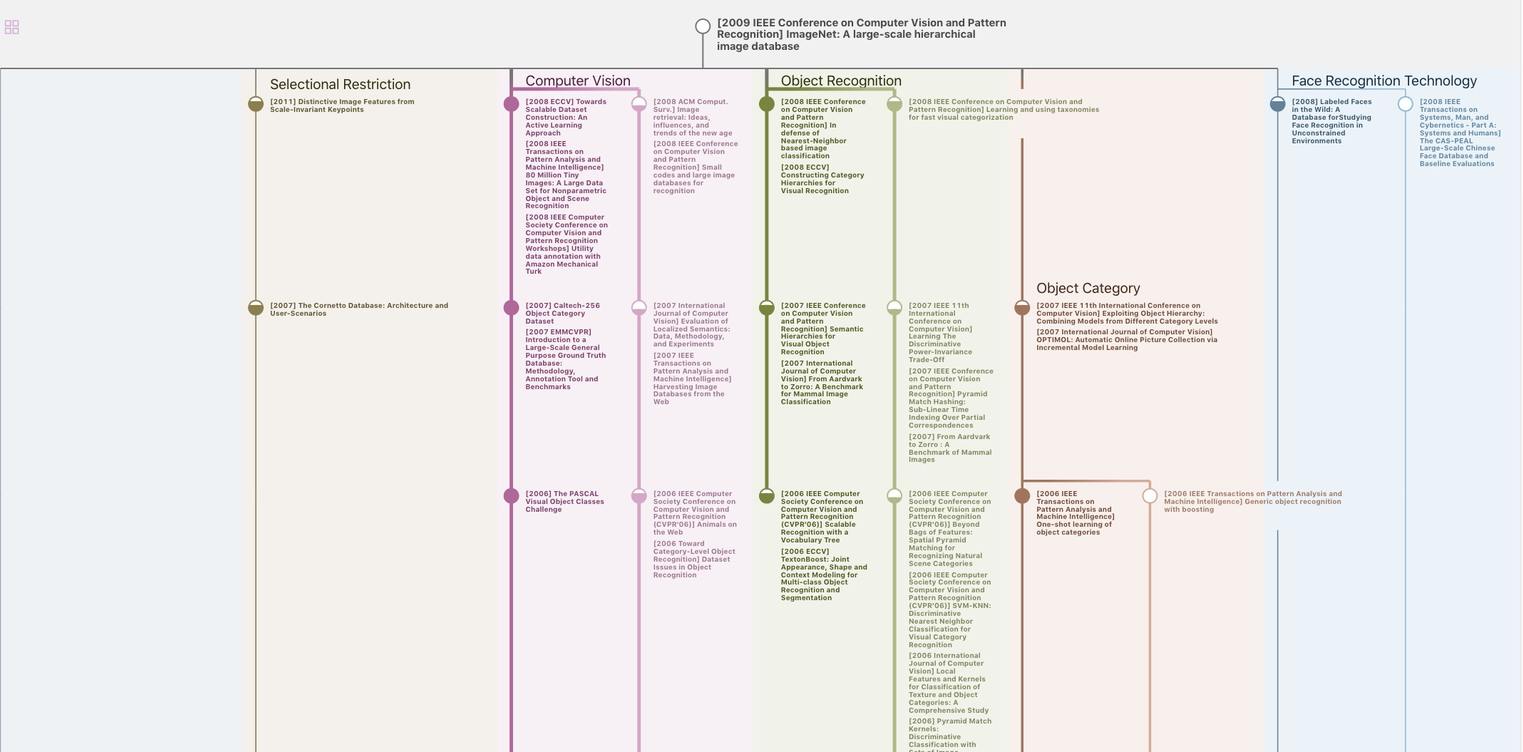
生成溯源树,研究论文发展脉络
Chat Paper
正在生成论文摘要