A Deep Learning Model for Estimating Tropical Cyclone Wind Radius from Geostationary Satellite Infrared Imagery
MONTHLY WEATHER REVIEW(2023)
Abstract
This article developed a deep learning (DL) model for estimating tropical cyclone (TC) 34-, 50-, and 64-kt (1 kt approximate to 0.51 m s(-1)) wind radii in four quadrants from infrared images in the global ocean. We collected 63 675 TC images from 2004 to 2016 and divided them into three periods (2004-12, 2013-14, and 2015-16) for model training, validation, and testing. First, four DL-based radius estimation models were developed to estimate the TC wind radius for each of the four quadrants. Then, the entire original images and the one-quarter-quadrant subimages were included in the model training for each quadrant. Last, we modified the mean absolute error (MAE) loss function in these DL-based models to reduce the side effect of an unbalanced distribution of wind radii and developed an asymmetric TC wind radius estimation model globally. The comparison of model results with the best-track data of TCs shows that the MAEs of 34-kt wind radius are 18.8, 19.5, 18.6, and 18.8 n mi (1 n mi = 1.852 km) for the northeast, southeast, southwest, and northwest quadrants, respectively. The MAEs of 50-kt wind radius are 11.3, 11.3, 11.1, and 10.8 n mi, respectively, and the MAEs of 64-kt wind radius are 8.9, 9.9, 9.2, and 8.7 n mi, respectively. These results represent a 12.1%-35.5% improvement over existing methods in the literature. In addition, the DL-based models were interpreted with two deep visualization toolboxes. The results indicate that the TC eye, cloud, and TC spiral structure are the main factors that affect the model performance.
MoreTranslated text
Key words
Tropical cyclones,Remote sensing,Deep learning
AI Read Science
Must-Reading Tree
Example
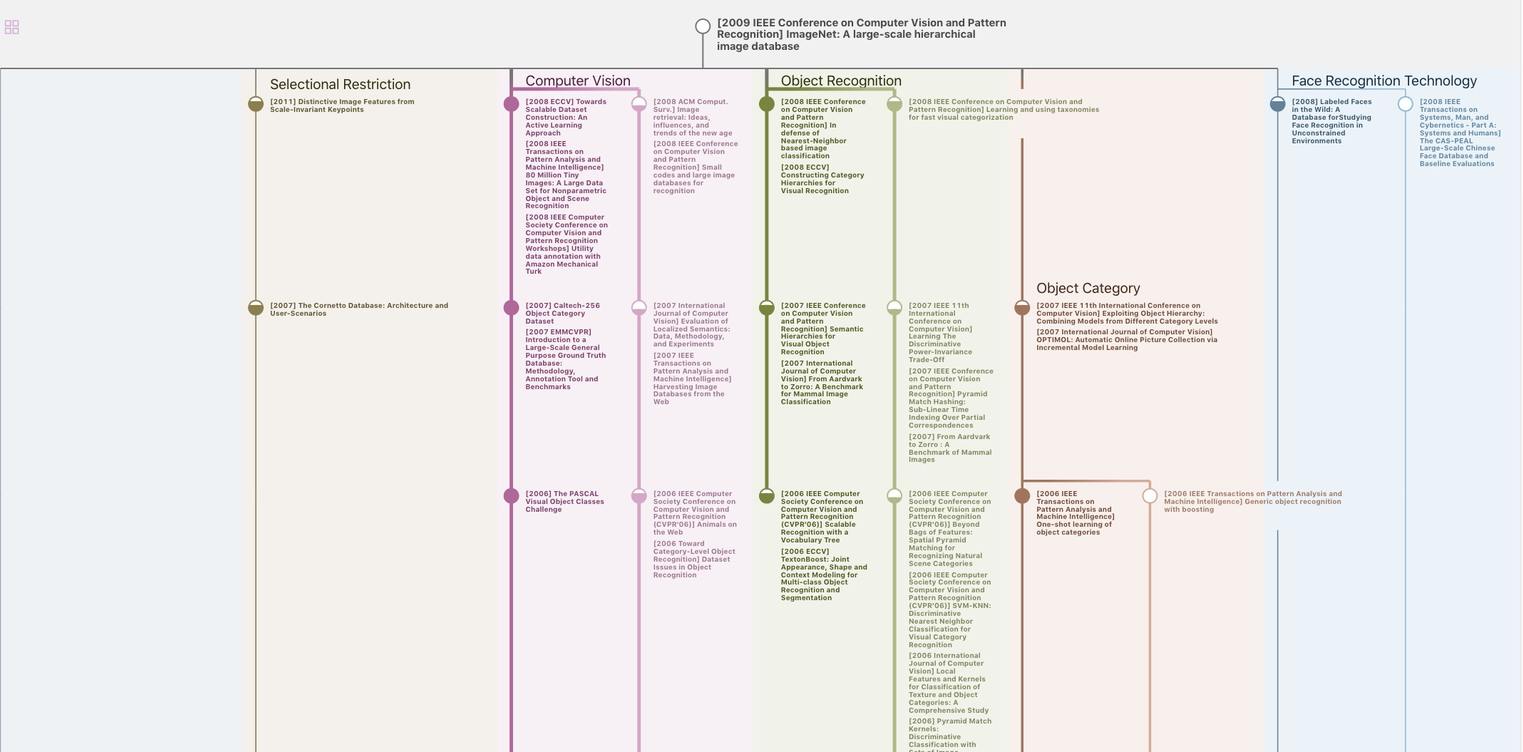
Generate MRT to find the research sequence of this paper
Chat Paper
Summary is being generated by the instructions you defined