Video Representation Learning for Temporal Action Detection Using Global-Local Attention
Pattern Recognition(2022)
摘要
Video representation is of significant importance for temporal action detection. The two sub-tasks of temporal action detection, i.e., action classification and action localization, have different requirements for video representation. Specifically, action classification requires video representations to be highly dis-criminative, so that action features and background features are as dissimilar as possible. For action local-ization, it is crucial to obtain information about the action itself and the surrounding context for accurate prediction of action boundaries. However, the previous methods failed to extract the optimal representa-tions for the two sub-tasks, whose representations for both sub-tasks are obtained in a similar way. In this paper, a Global-Local Attention (GLA) mechanism is proposed to produce a more powerful video rep-resentation for temporal action detection without introducing additional parameters. The global attention mechanism predicts each action category by integrating features in the entire video that are similar to the action while suppressing other features, thus enhancing the discriminability of video representation during the training process. The local attention mechanism uses a Gaussian weighting function to inte-grate each action and its surrounding contextual information, thereby enabling precise localization of the action. The effectiveness of GLA is demonstrated on THUMOS'14 and ActivityNet-1.3 with a simple one -stage action detection network, achieving state-of-the-art performance among the methods using only RGB images as input. The inference speed of the proposed model reaches 1373 FPS on a single Nvidia Titan Xp GPU. The generalizability of GLA to other detection architectures is verified using R-C3D and Decouple-SSAD, both of which achieve consistent improvements. The experimental results demonstrate that designing representations with different properties for the two sub-tasks leads to better performance for temporal action detection compared to the representations obtained in a similar way.(c) 2022 Elsevier Ltd. All rights reserved.
更多查看译文
关键词
Temporal action detection,Video representation,Untrimmed video analysis
AI 理解论文
溯源树
样例
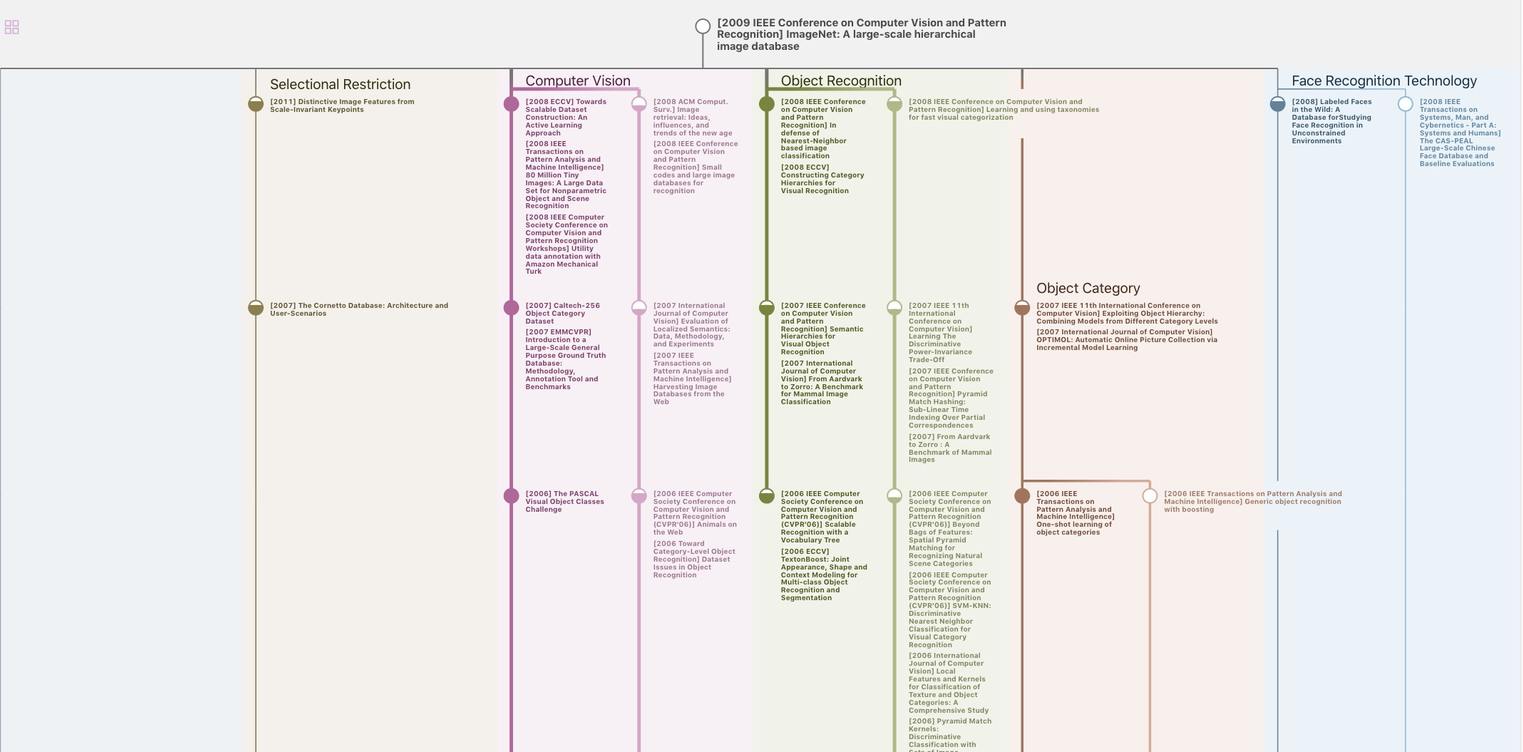
生成溯源树,研究论文发展脉络
Chat Paper
正在生成论文摘要