Deciphering tumor ecosystems at super resolution from spatial transcriptomics with TESLA
CELL SYSTEMS(2023)
摘要
Cell populations in the tumor microenvironment (TME), including their abundance, composition, and spatial location, are critical determinants of patient response to therapy. Recent advances in spatial transcriptomics (ST) have enabled the comprehensive characterization of gene expression in the TME. However, popular ST platforms, such as Visium, only measure expression in low-resolution spots and have large tissue areas that are not covered by any spots, which limits their usefulness in studying the detailed structure of TME. Here, we present TESLA, a machine learning framework for tissue annotation with pixel-level resolution in ST. TESLA integrates histological information with gene expression to annotate heterogeneous immune and tumor cells directly on the histology image. TESLA further detects unique TME features such as tertiary lymphoid struc-tures, which represents a promising avenue for understanding the spatial architecture of the TME. Although we mainly illustrated the applications in cancer, TESLA can also be applied to other diseases.
更多查看译文
关键词
spatial transcriptomics,tumor ecosystems
AI 理解论文
溯源树
样例
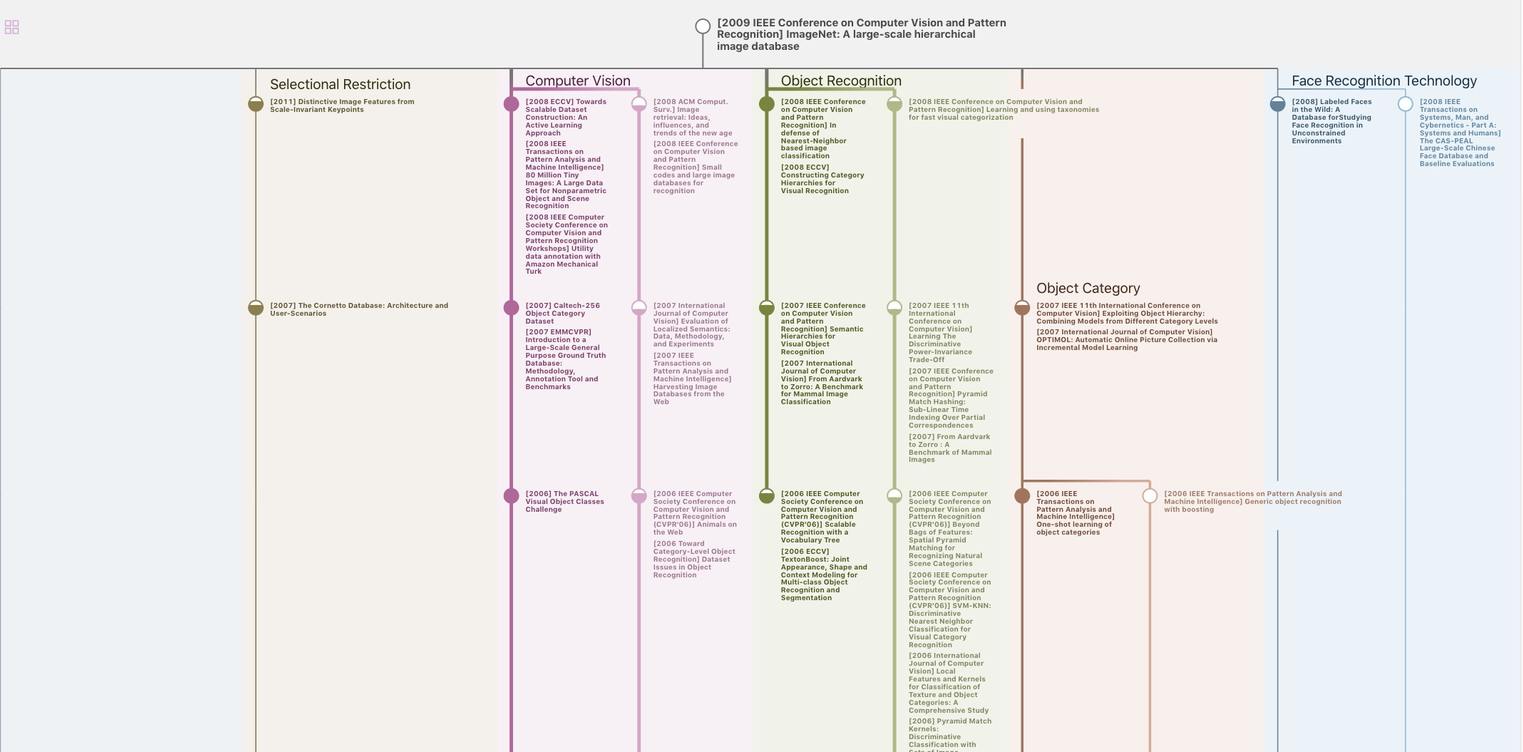
生成溯源树,研究论文发展脉络
Chat Paper
正在生成论文摘要