Power control and resource allocation for return on investment maximization in cross-modal communications
Transactions on emerging telecommunications technologies(2023)
摘要
Multi-modal services will become the mainstream in future applications, including video, audio, and haptic flows. In multi-modal services, video and audio primarily require high-rate transmission, while haptic flow requires ultra-high reliability and ultra-low latency transmission, which imposes unprecedented challenges on the network. To improve the transmission efficiency of multi-modal services, this article proposes a cross-modal communication architecture in the cloud radio access network based on network slicing technology. First, we take the data rate and transmission latency of video, audio, and haptic flows contents as the quality of service (QoS) parameters, and send different modes of flows into varying slices for transmission. Second, to transmit the cross-modal services efficiently while saving communication resources as many as possible, we define our optimization goal as the form of return on investment (ROI), that is, we aim to maximize the return of QoS parameters under the limited investment in communication resources. Third, we propose a two-stage framework to solve this ROI maximization problem. In the first stage, we propose the power control gradient assisted binary search algorithm to solve the power control problem, which can achieve the optimization of data rate and transmission latency and obtain the suitable QoS. Then, in the second stage, we introduce the network slicing ant colony optimization algorithm to solve the slice resource allocation problem, and get the largest possible ROI. The simulation results verify the effectiveness of the proposed cross-modal communication architecture, and prove the optimum of the proposed algorithm in terms of ROI compared to some existing algorithms, such as the reverse labeling Dijkstra algorithm, the delay acceptance algorithm, and the random searching algorithm.
更多查看译文
AI 理解论文
溯源树
样例
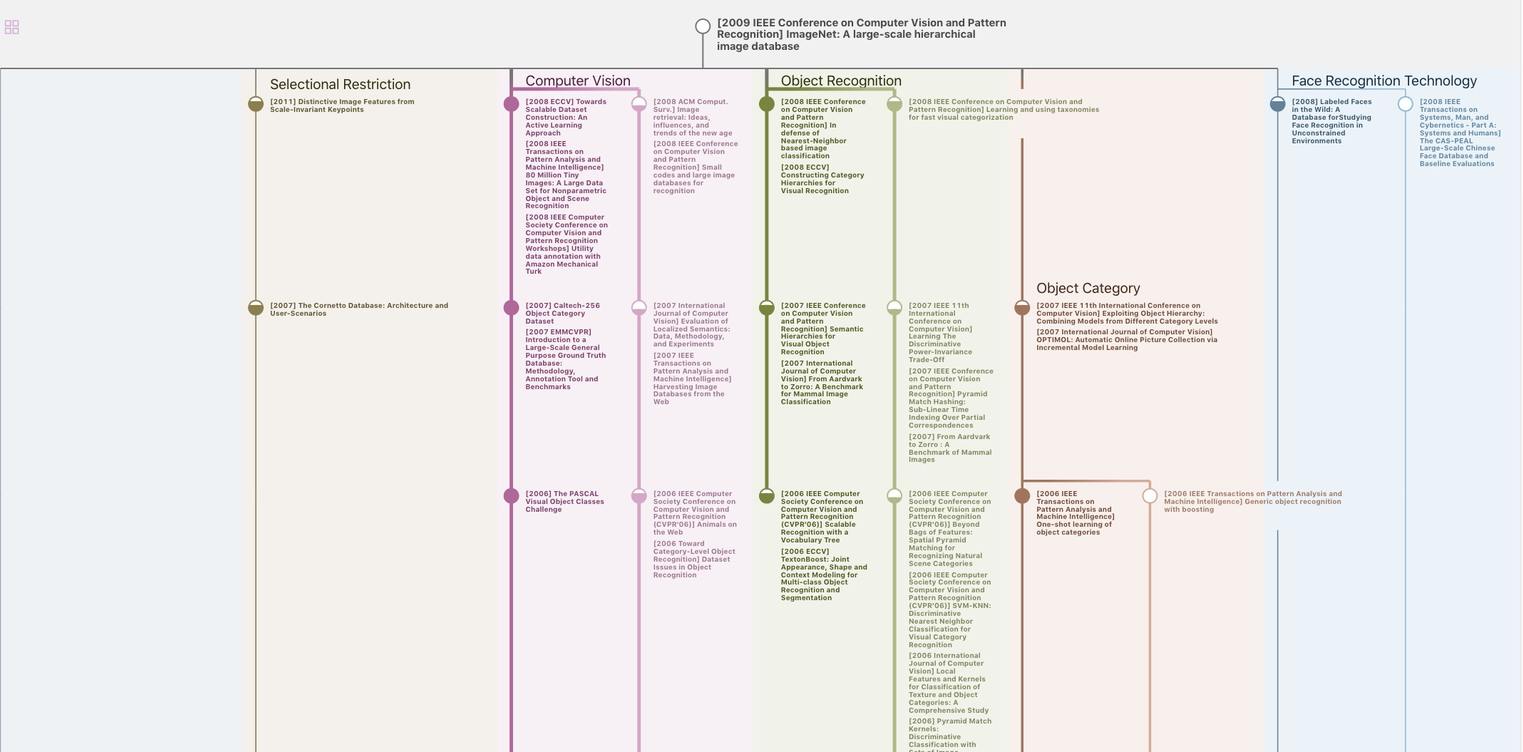
生成溯源树,研究论文发展脉络
Chat Paper
正在生成论文摘要