Improving Generalization by Learning Geometry-Dependent and Physics-Based Reconstruction of Image Sequences
IEEE Transactions on Medical Imaging(2023)
摘要
Deep neural networks have shown promise in image reconstruction tasks, although often on the premise of large amounts of training data. In this paper, we present a new approach to exploit the geometry and physics underlying electrocardiographic imaging (ECGI) to learn efficiently with a relatively small dataset. We first introduce a non-Euclidean encoding-decoding network that allows us to describe the unknown and measurement variables over their respective geometrical domains. We then explicitly model the geometry-dependent physics in between the two domains via a bipartite graph over their graphical embeddings. We applied the resulting network to reconstruct electrical activity on the heart surface from body-surface potentials. In a series of generalization tasks with increasing difficulty, we demonstrated the improved ability of the network to generalize across geometrical changes underlying the data using less than 10% of training data and fewer variations of training geometry in comparison to its Euclidean alternatives. In both simulation and real-data experiments, we further demonstrated its ability to be quickly fine-tuned to new geometry using a modest amount of data.
更多查看译文
关键词
Geometric deep learning,inverse problems,physics-based deep learning
AI 理解论文
溯源树
样例
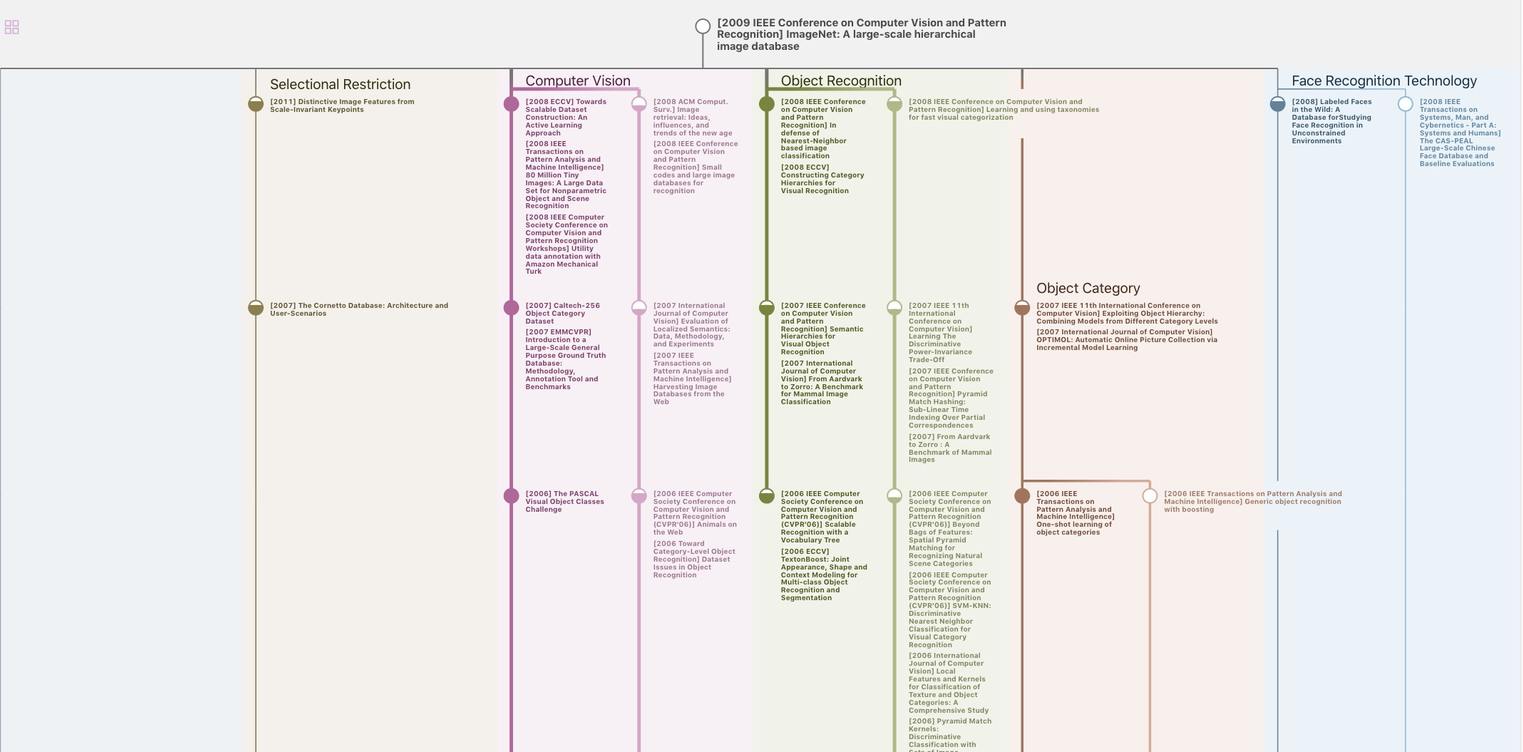
生成溯源树,研究论文发展脉络
Chat Paper
正在生成论文摘要