Development and implementation of centralised, cloud-based, employee health contact tracing database and predictive modelling framework in the COVID-19 pandemic.
The Lancet. Digital health(2022)
摘要
At the onset of the COVID-19 pandemic in early March, 2020, it became apparent that non-electronic exposure investigations would not meet the demands required by the mounting number of employees with COVID-19 in the Mount Sinai Health System in New York City (NY, USA), a multicentre, academic medical institution and hospital system. New York City was an epicentre early in the pandemic, resulting in a peak of more than 6000 cases daily and more than 1000 deaths per day.1NYC HealthCOVID-19: data. City of New York.https://www1.nyc.gov/site/doh/covid/covid-19-data-totals.pageDate: 2021Date accessed: February 1, 2021Google Scholar Agile exposure investigation and contact tracing were crucial to containing the spread of COVID-19 among hospital staff.2US Centers for Disease Control and PreventionCase investigation and contact tracing: part of a multipronged approach to fight the COVID-19 pandemic.https://www.cdc.gov/coronavirus/2019-ncov/php/principles-contact-tracing.htmlDate: 2021Date accessed: February 1, 2021Google Scholar Unlike patient-facing electronic health records with continuously improving platforms, employee health data management methods have not developed uniformly in the USA and often rely on paper-based or non-integrated systems of record keeping.3Bronsoler A Doyle J Van Reenen J The impact of new technology on the healthcare workforce.https://workofthefuture.mit.edu/wp-content/uploads/2020/10/2020-Research-Brief-Bronsoler-Doyle-VanReenen.pdfDate: 2020Date accessed: February 1, 2021Google Scholar Even when electronic health records are incorporated into employee health service departments, this infrastructure lacks the required flexibility to meet the demand from changing guidance about the disease.4Breeher L Boon A Hainy C Murad MH Wittich C Swift M A framework for sustainable contact tracing and exposure investigation for large health systems.Mayo Clinic Proc. 2020; 95: 1432-1444Summary Full Text Full Text PDF PubMed Scopus (15) Google Scholar At our institution, the Mount Sinai Health System, this need was met with the creation of the Employee Health COVID-19 REDCap Registry (EHCRR) using the well known Research Electronic Data Capture (REDCap) platform.5Harris PA Taylor R Thielke R Payne J Gonzalez N Conde JG Research electronic data capture (REDCap)—a metadata-driven methodology and workflow process for providing translational research informatics support.J Biomed Inform. 2009; 42: 377-381Crossref PubMed Scopus (22280) Google Scholar The EHCRR is a user-friendly, cloud-based, reproducible electronic system for tracking employee exposures to and illness due to COVID-19. We outline the development and implementation of the EHCRR at our institution. Although the EHCRR was created to support COVID-19 clinical work, it captures rich qualitative data relevant for current and future studies. Additionally, this modifiable tool can be used for data sharing and information management in resource-limited settings without compromising data integrity. As a Health Insurance Portability and Accountability Act (HIPPA)-compliant, cloud-hosted solution, this system can be used by institutions across the USA as well as internationally. The EHCRR system is available for download in a link, which will generate a request from the corresponding author. The design of the EHCRR enables the collection of both structured and unstructured datasets.6Boulton D Hammersley M Analysis of unstructured data.Data Collect Anal. 1996; 11: 282-297Google Scholar Through REDCap's database, we created three interfaces with five areas of impact. The EHCRR provides employee-facing health data collection using secure, online, user-friendly forms; employee health service contact-tracing investigations for employee monitoring and workflow capture; multidisciplinary review and qualitative analysis of employee interviews; analysis and genomic sequencing integration; and the potential for machine learning in exposure investigations by using supervised machine-learning principles in the development of the contact-tracing data collection interfaces. The EHCRR is not designed for clinical research; rather, it establishes a platform for acute clinical care and standardised decision making in the context of the pandemic. So far, there have been more than 500 revisions of the framework, with more than 50 000 employee interviews and 1775 pieces of structured data collected per interview. The iteratively designed platform provides flexibility, which pairs well with evolving knowledge around transmission dynamics and changes in national recommendations regarding COVID-19. With the EHCRR, employee health services reduced case follow-up time from days to hours. For example, in the beginning of November, 2020, an employee reported to work symptomatic at 0600 h. With the EHCRR, the employee health service was able to follow-up with the employee's manager, who reported the employee's symptoms, conduct the initial contact tracing interview, and send the employee home for isolation by 0900 h. Follow-up contact tracing of this case led to the detection of an emerging cluster within the department. In total, 19 exposed employees, five of whom tested positive, were identified in this cluster. Finally, directionality of transmission was determined by incorporating genomic sequencing data provided by the institution's genomics laboratory (figure). The EHCRR and its cloud-based presence with remote access allows for integration at all Mount Sinai Health System hospitals and clinics via internet-capable devices. The EHCRR's accessibility removes historical silos between departments and provides a user-friendly data repository with the ability to create modifiable intake forms. The agility demonstrated in the example above is due to the employee-facing interfaces the employee health service was able to create and disseminate to all staff through web forms that could be shared. One form was created for mandatory daily symptom attestation for employees before reporting for work, and a second for employees to report either testing positive for COVID-19 or having a high-risk exposure. The web forms allow for prompt follow-up by staff because they can be completed on personal devices and submitted to the employee health service instantaneously. This workflow provides an educational opportunity for employees to speak directly with employee health service clinical staff to learn about the disease and its transmission, hospital policies, and protected COVID-19 sick leave. By connecting employees directly with the employee health service, barriers to reporting due to fear of retaliation by supervisors or the loss of pay due to time off for a positive test or a high-risk exposure are mitigated. The pre-built EHCRR registry forms have automated follow-up times for obtaining test results, checking symptom improvement, and providing return-to-work information to assist the employee health service in case management and tracking. The employee health service contact-tracing interface captures information regarding employee demographics, quarantine length, personal protective equipment used, and COVID-19 testing (ie, structured data), and is collected alongside free-text notes (ie, unstructured data) from conversations with the employee. The interviews provide narrative context for the employee's infection and potential risk of spread (eg, whether the employee carpooled with colleagues or whether their spouse is also an employee). The form enables the application of risk stratification definitions based on the level of exposure and the subsequent need for quarantine. Cases due to exposure to colleagues are linked, and information is communicated with infection prevention teams to aid in follow-up investigations. In designing the employee health service contact-tracing interface, the team created an exposure matrix to assess the risk of reported exposure (low, medium, or high). The matrix outlines risk scores based on the type of exposure, and employee health service staff code the exposure according to this scale. We hope to use this scoring method in creating a classification model for supervised machine learning to predict exposure outcomes.7Tostmann A Bradley J Bousema T et al.Strong associations and moderate predictive value of early symptoms for SARS-CoV-2 test positivity among healthcare workers, the Netherlands, March 2020.Euro Surveill. 2020; 252000508Crossref Scopus (145) Google Scholar Additionally, this overall collection of structured and unstructured data provides us with a unique opportunity to build deep-learning models for SARS-CoV-2 transmission in the future.8Zhang D Yin C Zeng J Yuan X Zhang P Combining structured and unstructured data for predictive models: a deep learning approach.BMC Med Inform Decis Mak. 2020; 20: 280Crossref Scopus (36) Google Scholar Last, the EHCRR database is intentionally built with an event-based design in mind, in which every exposure—ie, event—is recorded uniquely without linking it to previous events for the same person, department, and so on. With this structure, we can associate certain events and identify burgeoning clusters of infections to focus follow-up investigations. This design choice will pay dividends in future analyses, as we are seeing substantial changes in disease transmission with the spread of delta and omicron variants of the virus. As the pandemic progressed, we incorporated the tool in research activities. Researchers enrol and are granted access to the standardised data repository created by the EHCRR, with restrictions built in for employee privacy. The EHCRR also provides the infrastructure for employees to enrol in COVID-19 research conducted by the institution; for example, employees elected to enrol to donate immunogenic plasma during the early phase of the pandemic. Additionally, the EHCRR allows for analysis of subgroups of employees to determine risk factors (occupational and non-occupational) associated with contracting COVID-19.9Pawloski KR Kolod B Khan RF et al.Factors associated with SARS-CoV-2 infection in physician trainees in New York City during the first COVID-19 wave.Int J Environ Res Public Health. 2021; 185274Crossref Scopus (2) Google Scholar To conclude, we developed the EHCRR into an agile and robust tool for employee contact tracing with comprehensive oversight of our employees’ footprints, within the changing landscape of the COVID-19 pandemic. We declare no competing interests. Study data were collected and managed using REDCap electronic data capture tools hosted at the Mount Sinai Health System. REDCap is a secure, web-based software platform designed to support data capture for research studies, providing an intuitive interface for validated data capture; audit trails for tracking data manipulation and export procedures; automated export procedures for seamless data downloads to common statistical packages; and procedures for data integration and interoperability with external sources. This work is supported by grant UL1TR001433 from the US National Center for Advancing Translational Sciences, National Institutes of Health. We thank the Infection prevention department at Mount Sinai Beth Israel Hospital, and the employee health teams who supported the work, which made this project possible. This project did not meet the criteria for human participant research so institutional review board review or approval was not needed.
更多查看译文
AI 理解论文
溯源树
样例
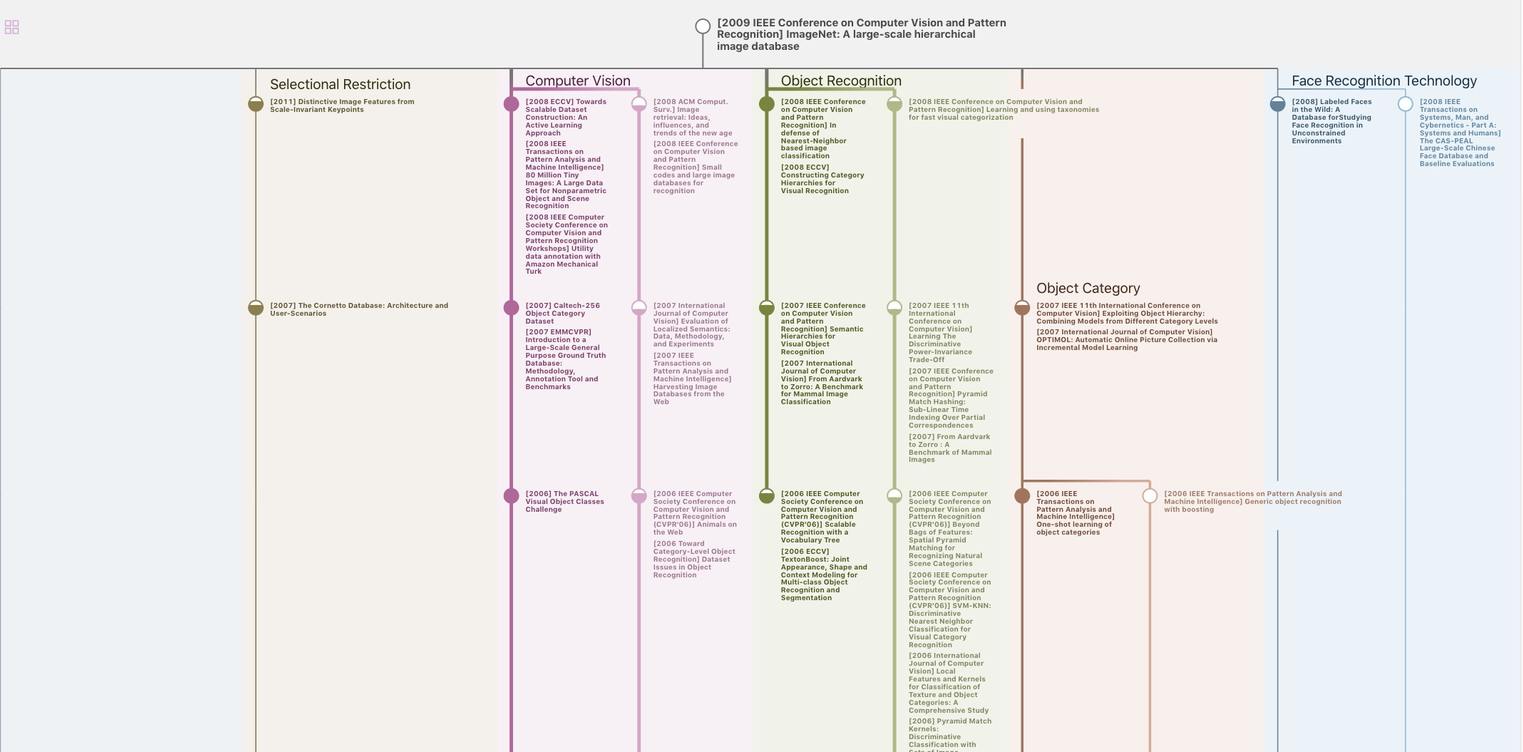
生成溯源树,研究论文发展脉络
Chat Paper
正在生成论文摘要