Unsupervised Early Detection of Physical Activity Behaviour Changes from Wearable Accelerometer Data
Sensors(2022)
摘要
Wearable accelerometers record physical activity with high resolution, potentially capturing the rich details of behaviour changes and habits. Detecting these changes as they emerge is valuable information for any strategy that promotes physical activity and teaches healthy behaviours or habits. Indeed, this offers the opportunity to provide timely feedback and to tailor programmes to each participant’s needs, thus helping to promote the adherence to and the effectiveness of the intervention. This article presents and illustrates U-BEHAVED, an unsupervised algorithm that periodically scans step data streamed from activity trackers to detect physical activity behaviour changes to assess whether they may become habitual patterns. Using rolling time windows, current behaviours are compared with recent previous ones, identifying any significant change. If sustained over time, these new behaviours are classified as potentially new habits. We validated this detection algorithm using a physical activity tracker step dataset (N = 12,798) from 79 users. The algorithm detected 80% of behaviour changes of at least 400 steps within the same hour in users with low variability in physical activity, and of 1600 steps in those with high variability. Based on a threshold cadence of approximately 100 steps per minute for standard walking pace, this number of steps would suggest approximately 4 and 16 min of physical activity at moderate-to-vigorous intensity, respectively. The detection rate for new habits was 80% with a minimum threshold of 500 or 1600 steps within the same hour in users with low or high variability, respectively.
更多查看译文
关键词
behaviour changes,physical activity,unsupervised learning,activity tracker,accelerometer
AI 理解论文
溯源树
样例
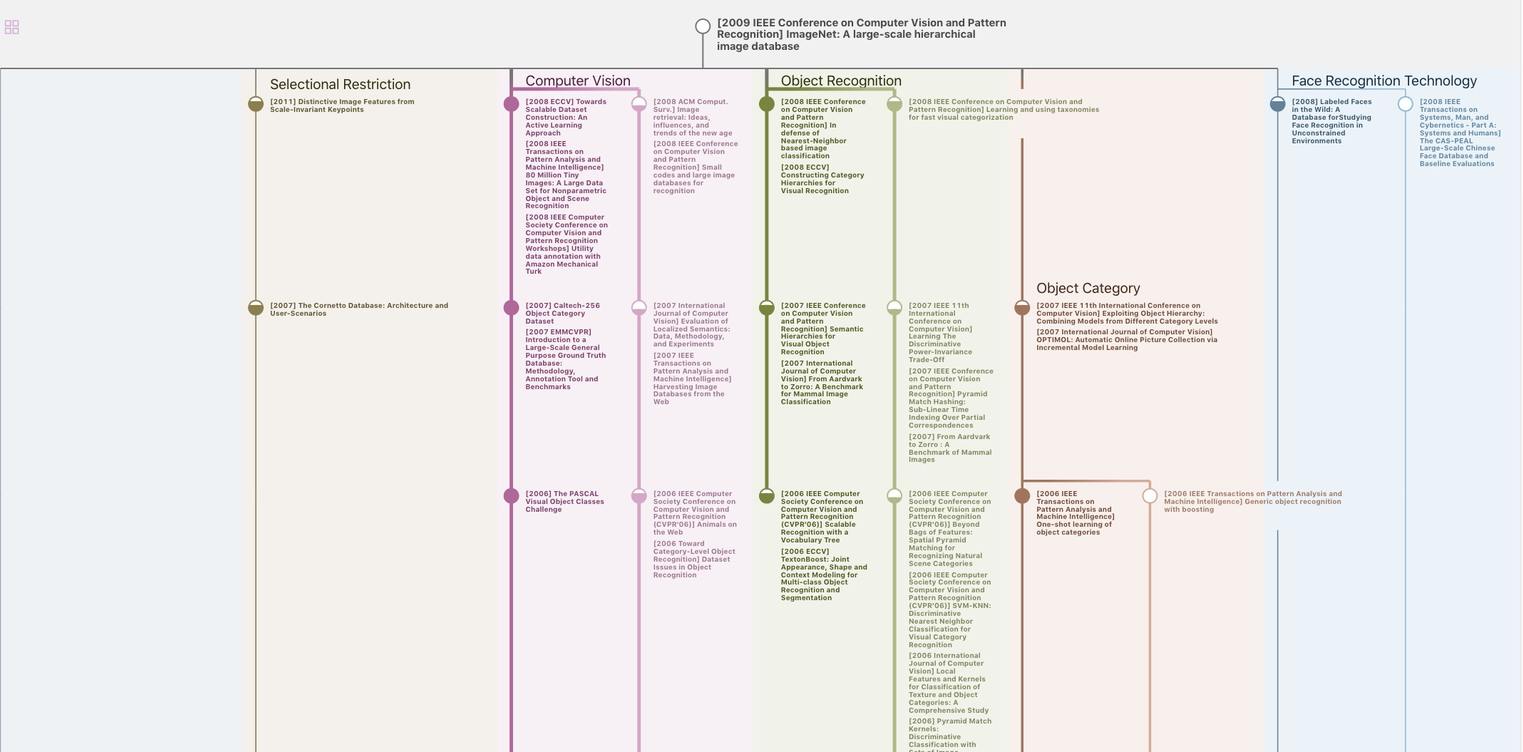
生成溯源树,研究论文发展脉络
Chat Paper
正在生成论文摘要