Dysfluencies Seldom Come Alone -- Detection as a Multi-Label Problem
arxiv(2022)
摘要
Specially adapted speech recognition models are necessary to handle stuttered speech. For these to be used in a targeted manner, stuttered speech must be reliably detected. Recent works have treated stuttering as a multi-class classification problem or viewed detecting each dysfluency type as an isolated task; that does not capture the nature of stuttering, where one dysfluency seldom comes alone, i.e., co-occurs with others. This work explores an approach based on a modified wav2vec 2.0 system for end-to-end stuttering detection and classification as a multi-label problem. The method is evaluated on combinations of three datasets containing English and German stuttered speech, yielding state-of-the-art results for stuttering detection on the SEP-28k-Extended dataset. Experimental results provide evidence for the transferability of features and the generalizability of the method across datasets and languages.
更多查看译文
关键词
detection,multi-label
AI 理解论文
溯源树
样例
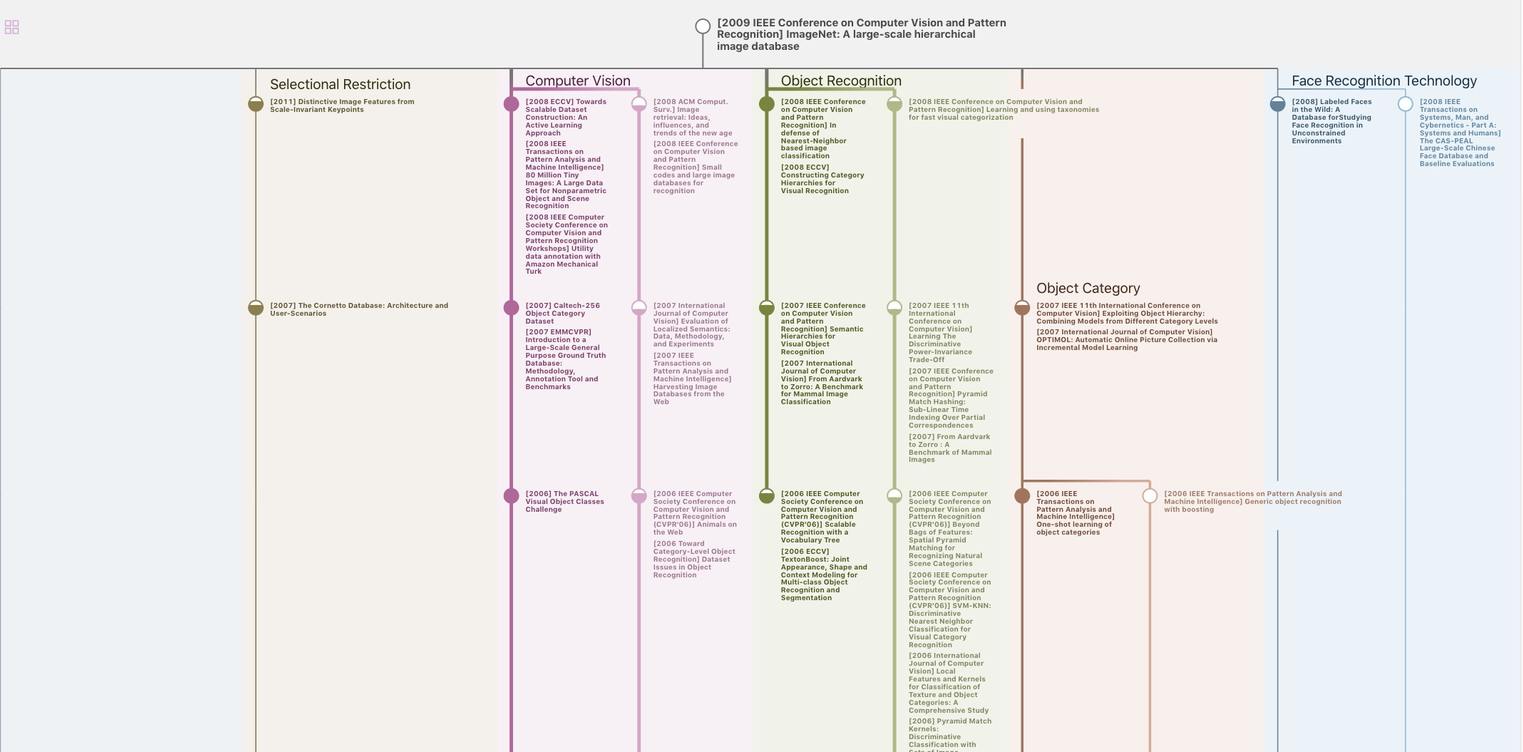
生成溯源树,研究论文发展脉络
Chat Paper
正在生成论文摘要