Deep Network Series for Large-Scale High-Dynamic Range Imaging
arXiv (Cornell University)(2022)
摘要
We propose a new approach for large-scale high-dynamic range computational imaging. Deep Neural Networks (DNNs) trained end-to-end can solve linear inverse imaging problems almost instantaneously. While unfolded architectures provide robustness to measurement setting variations, embedding large-scale measurement operators in DNN architectures is impractical. Alternative Plug-and-Play (PnP) approaches, where the denoising DNNs are blind to the measurement setting, have proven effective to address scalability and high-dynamic range challenges, but rely on highly iterative algorithms. We propose a residual DNN series approach, also interpretable as a learned version of matching pursuit, where the reconstructed image is a sum of residual images progressively increasing the dynamic range, and estimated iteratively by DNNs taking the back-projected data residual of the previous iteration as input. We demonstrate on radio-astronomical imaging simulations that a series of only few terms provides a reconstruction quality competitive with PnP, at a fraction of the cost.
更多查看译文
关键词
computational imaging,deep neural networks,unfolded architectures,plug-and-play,astronomical imaging
AI 理解论文
溯源树
样例
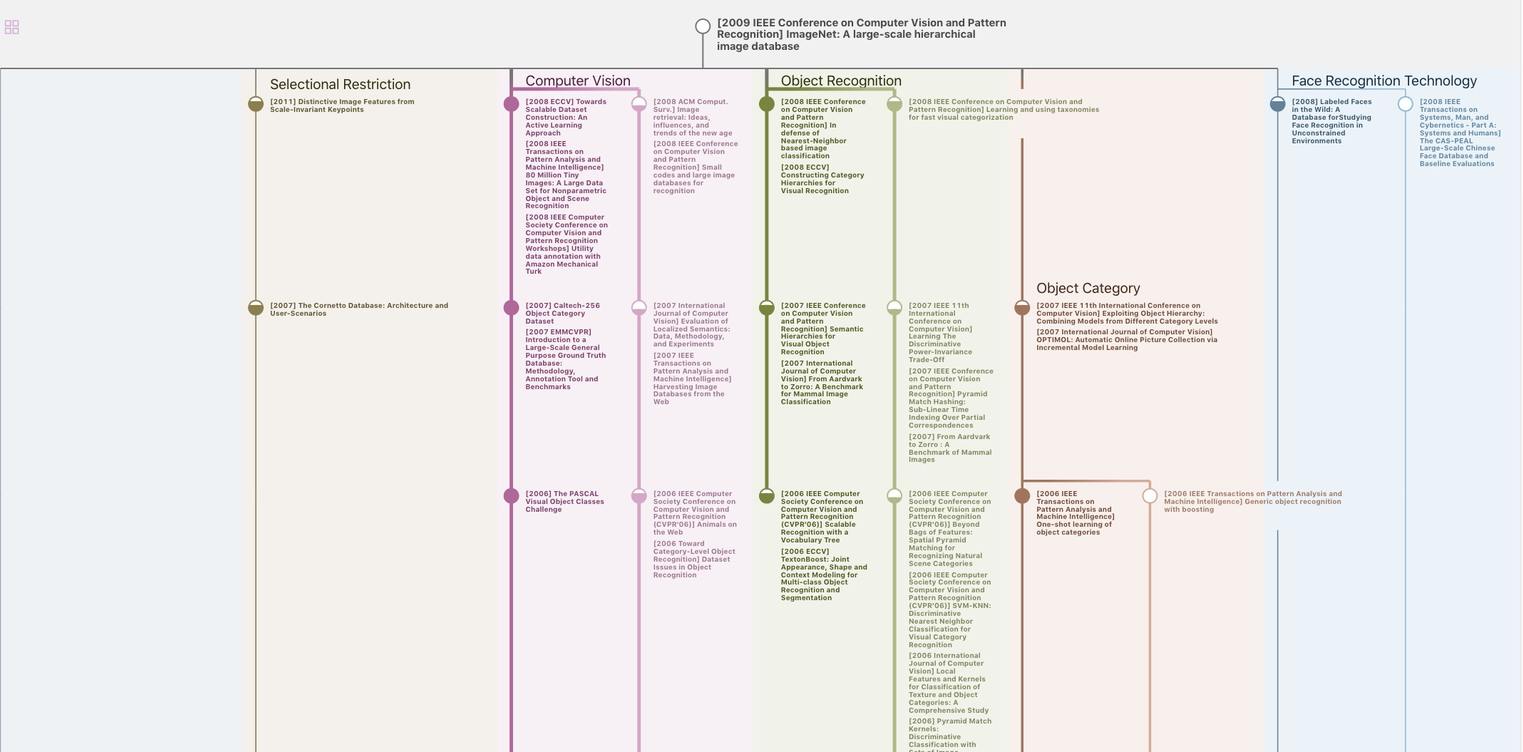
生成溯源树,研究论文发展脉络
Chat Paper
正在生成论文摘要