Robust and fuzzy ensemble framework via spectral learning for random projection-based fuzzy-c-means clustering
Engineering Applications of Artificial Intelligence(2023)
摘要
The ensembles of random projection-based fuzzy-c-means (RP-FCM) can handle high-dimensional data ef-ficiently. However, the performance of these ensemble frameworks is still hindered by some issues, such as misaligned membership matrices, information loss of co-similar matrices, large storage space, unstable ensemble results due to the additional re-clustering, etc. To address these issues, we propose a robust and fuzzy ensemble framework via spectral learning for RP-FCM clustering. After using random projection to generate different dimensional datasets and obtaining the membership matrices via fuzzy-c-means, we first convert these membership matrices into regularized graphs and approximates the affinity matrices of these graphs by spectral matrices. This step not only avoids the alignment problems of membership matrices but also excludes the storage of large-scale graphs. The spectral matrices of the same size are used as the features of membership matrices for the ensemble, avoiding the possible information loss by applying co-similar matrix transformations. More importantly, an optimization model is designed in our framework to learn the fusion of spectral features. In this model, the proportion of each base clustering is adjusted adaptively through a fuzzification exponent, and the effect of outliers is also suppressed by a robust norm. Finally, the Laplacian rank constraint in the model guarantees the ensemble can achieve the exact final partition. An efficient algorithm for this model is derived, and its time complexity and convergence are also analyzed. Competitive experimental results on benchmark data demonstrate the effectiveness of the proposed ensemble framework in comparison to state-of-the-art methods.
更多查看译文
关键词
Fuzzy-c-means,Random projection,Ensemble,Spectral learning,Robust
AI 理解论文
溯源树
样例
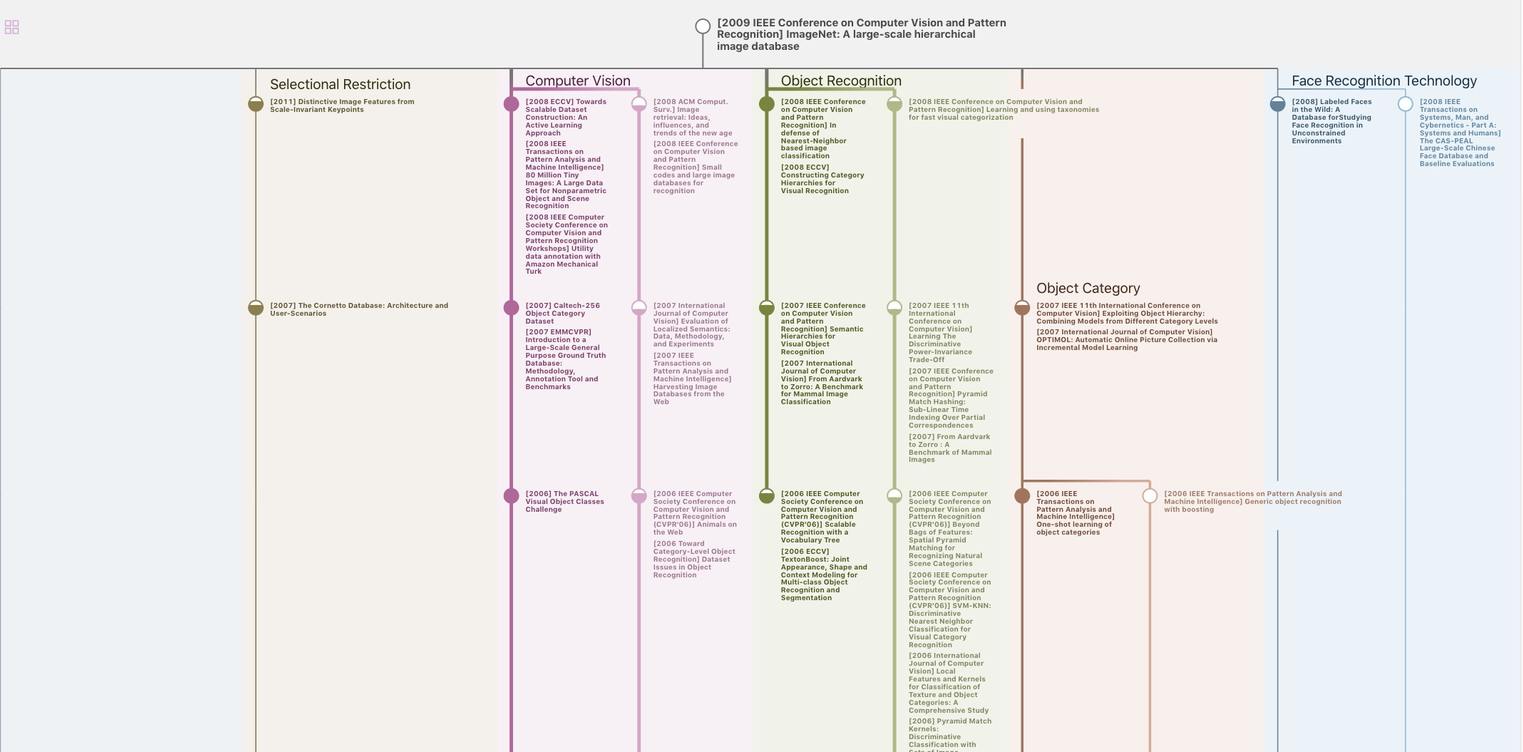
生成溯源树,研究论文发展脉络
Chat Paper
正在生成论文摘要