Quantum Fisher kernel for mitigating the vanishing similarity issue
arxiv(2022)
摘要
Quantum kernel method is a machine learning model exploiting quantum computers to calculate the quantum kernels (QKs) that measure the similarity between data. Despite the potential quantum advantage of the method, the commonly used fidelity-based QK suffers from a detrimental issue, which we call the vanishing similarity issue; detecting the difference between data becomes hard with the increase of the number of qubits, due to the exponential decrease of the expectation and the variance of the QK. This implies the need to design QKs alternative to the fidelity-based one. In this work, we propose a new class of QKs called the quantum Fisher kernels (QFKs) that take into account the geometric structure of the data source. We analytically and numerically demonstrate that the QFK based on the anti-symmetric logarithmic derivatives (ALDQFK) can avoid the issue when the alternating layered ansatzs (ALAs) are used, while the fidelity-based QK cannot even with the ALAs. Moreover, the Fourier analysis numerically elucidates that the ALDQFK can have expressivity comparable to that of the fidelity-based QK. These results indicate that the QFK paves the way for practical applications of quantum machine learning with possible quantum advantages.
更多查看译文
AI 理解论文
溯源树
样例
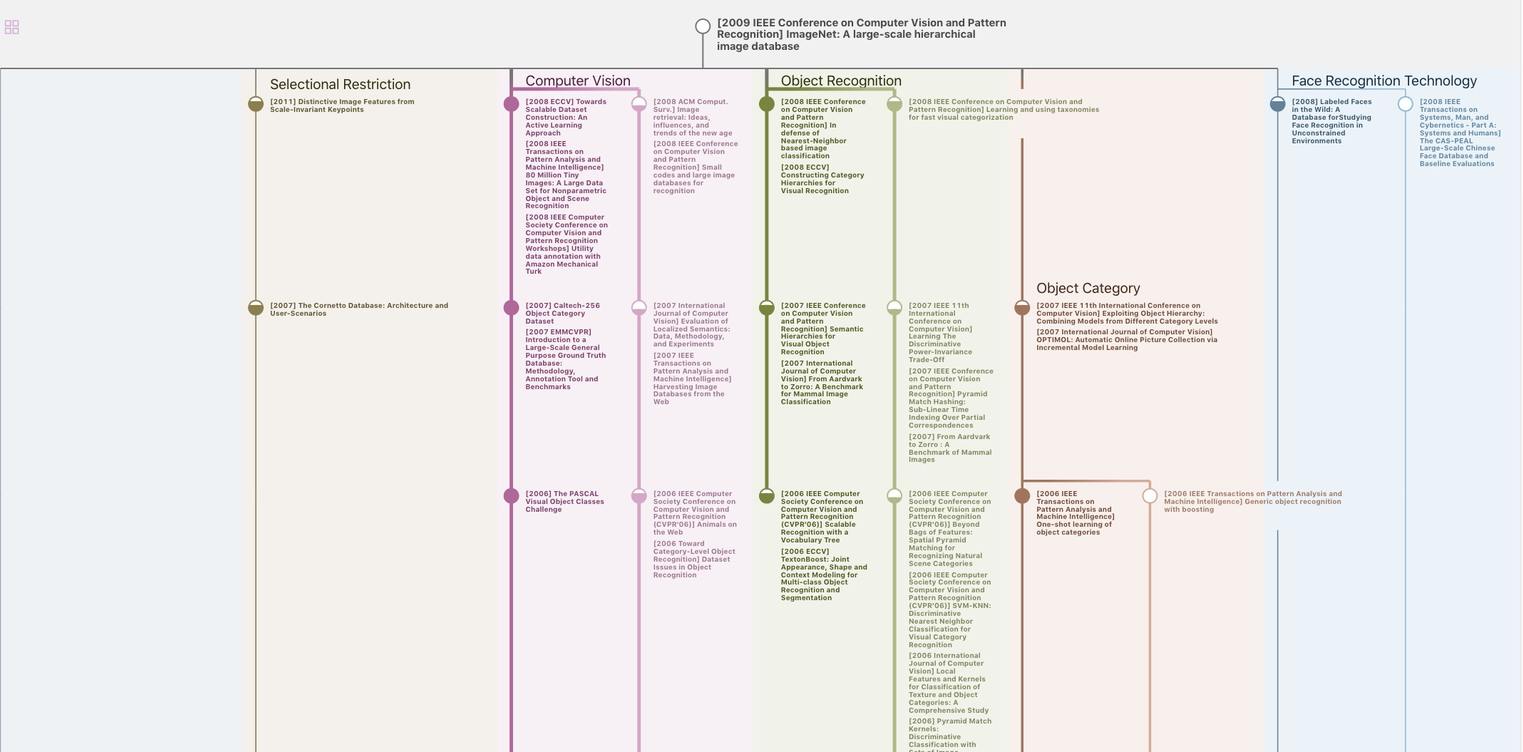
生成溯源树,研究论文发展脉络
Chat Paper
正在生成论文摘要