Speaker Representation Learning via Contrastive Loss with Maximal Speaker Separability
PROCEEDINGS OF 2022 ASIA-PACIFIC SIGNAL AND INFORMATION PROCESSING ASSOCIATION ANNUAL SUMMIT AND CONFERENCE (APSIPA ASC)(2022)
摘要
A great challenge in speaker representation learning using deep models is to design learning objectives that can enhance the discrimination of unseen speakers under unseen domains. This work proposes a supervised contrastive learning objective to learn a speaker embedding space by effectively leveraging the label information in the training data. In such a space, utterance pairs spoken by the same or similar speakers will stay close, while utterance pairs spoken by different speakers will be far apart. For each training speaker, we perform random data augmentation on their utterances to form positive pairs, and utterances from different speakers form negative pairs. To maximize speaker separability in the embedding space, we incorporate the additive angular-margin loss into the contrastive learning objective. Experimental results on CN-Celeb show that this new learning objective can cause ECAPA-TDNN to find an embedding space that exhibits great speaker discrimination. The contrastive learning objective is easy to implement, and we provide PyTorch code at https://github.com/shanmon110/AAMSupCon.
更多查看译文
关键词
additive angular-margin loss,CN-Celeb,contrastive learning objective,contrastive loss,deep models,ECAPA-TDNN,https://github.com/shanmon110/AAMSupCon,label information,maximal speaker separability,PyTorch code,random data augmentation,speaker discrimination,speaker embedding space,speaker representation learning,supervised contrastive learning,training speaker,unseen speakers,utterance pairs
AI 理解论文
溯源树
样例
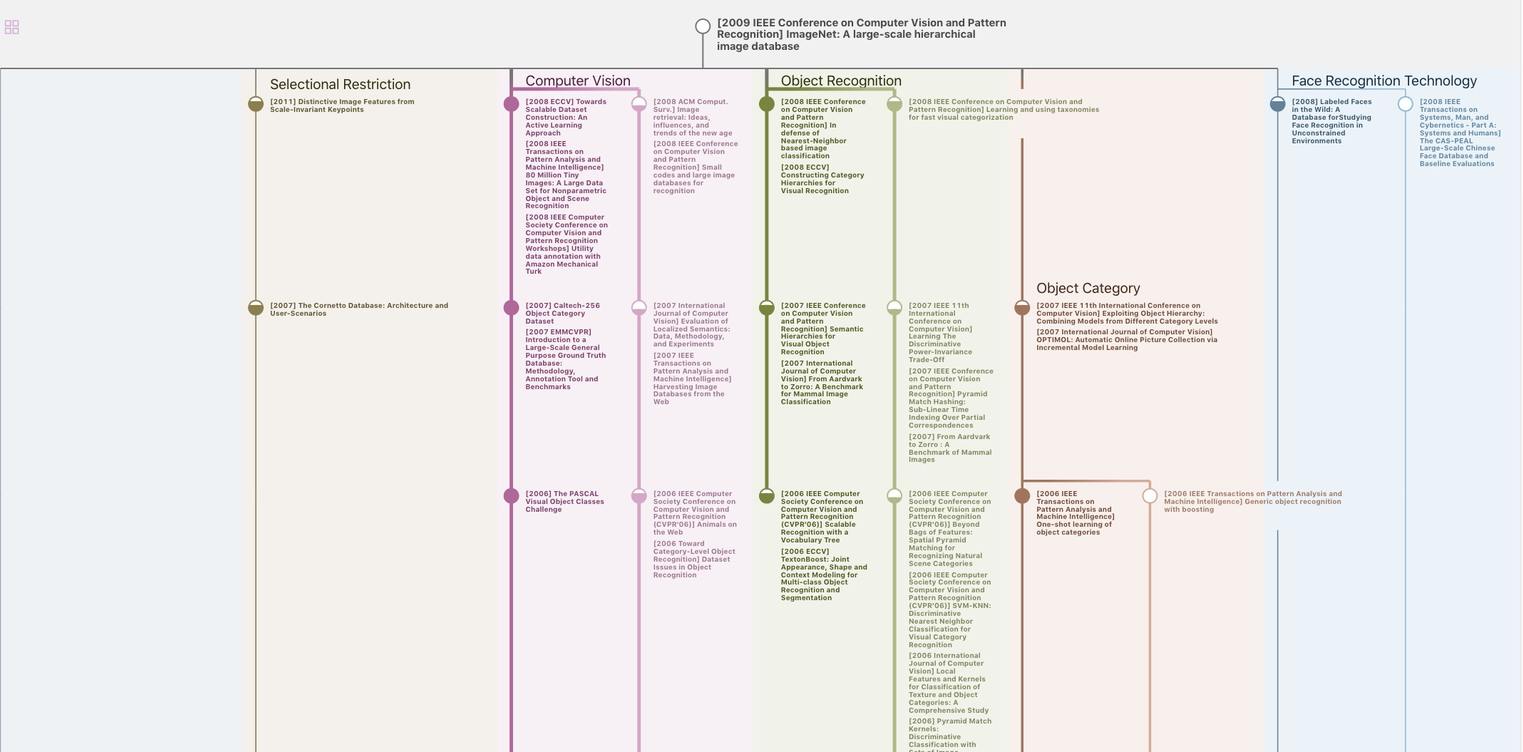
生成溯源树,研究论文发展脉络
Chat Paper
正在生成论文摘要