Embedding Space Augmentation for Weakly Supervised Learning in Whole-Slide Images
arxiv(2023)
摘要
Multiple Instance Learning (MIL) is a widely employed framework for learning on gigapixel whole-slide images (WSIs) from WSI-level annotations. In most MIL-based analytical pipelines for WSI-level analysis, the WSIs are divided into patches, and deep features for patches (i.e., patch embeddings) are extracted prior to training to reduce the overall computational cost and cope with the GPUs’ limited RAM. Because of this bottleneck, incorporating patch-level data augmentations during training adds an extra computational burden. To overcome this limitation, we present EmbAugmenter, a data augmentation generative adversarial network (DA-GAN) that can synthesize data augmentations in the embedding space rather than in the pixel space, thereby significantly reducing the computational requirements. Experiments on the SICAPv2 dataset show that our approach outperforms MIL without augmentation and is on par with traditional patch-level augmentation for MIL training while being substantially faster.
更多查看译文
关键词
weakly supervised learning,space augmentation,whole-slide
AI 理解论文
溯源树
样例
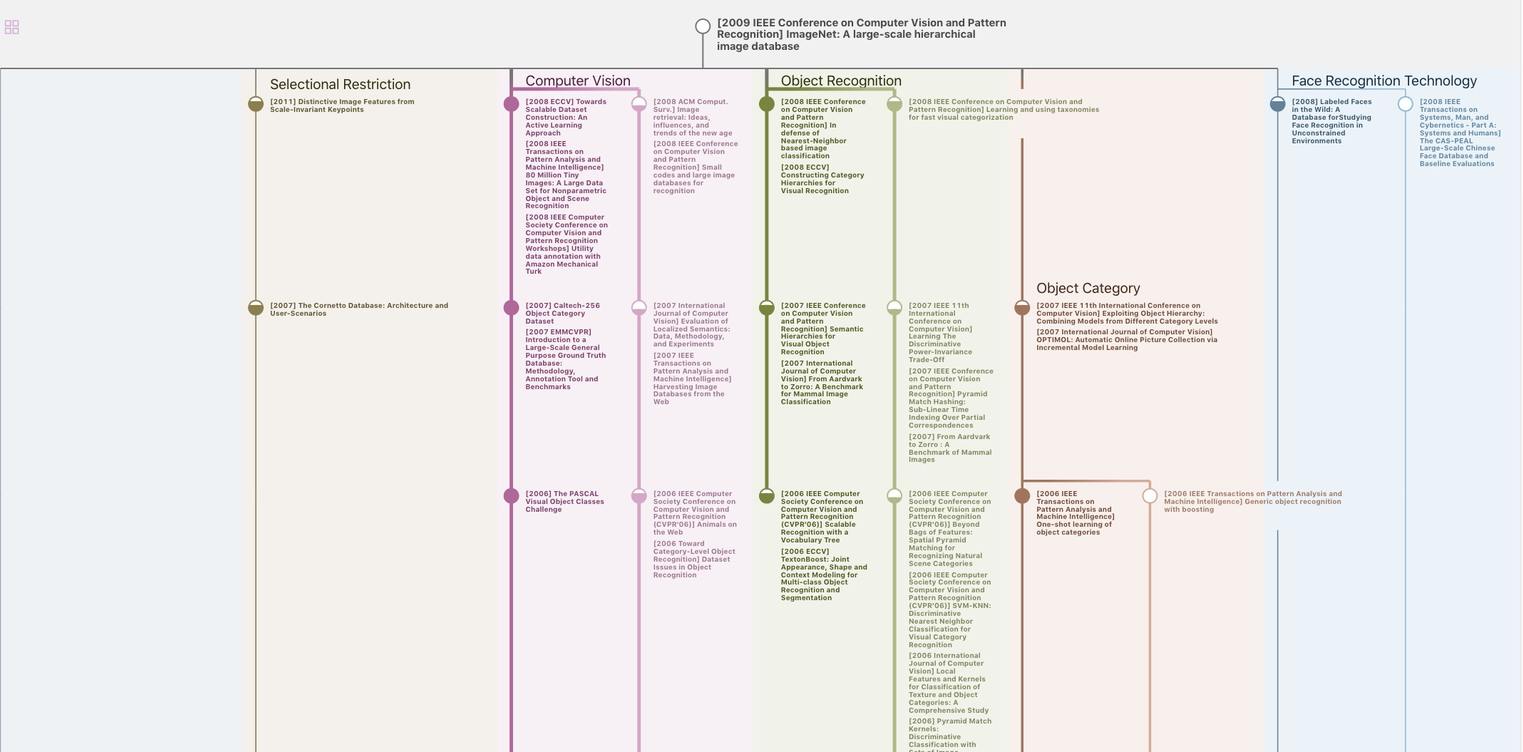
生成溯源树,研究论文发展脉络
Chat Paper
正在生成论文摘要