Accelerating Carbon Capture and Storage Modeling using Fourier Neural Operators
arxiv(2022)
摘要
Carbon capture and storage (CCS) is an important strategy for reducing carbon dioxide emissions and mitigating climate change. We consider the storage aspect of CCS, which involves injecting carbon dioxide into underground reservoirs. This requires accurate and high-resolution predictions of carbon dioxide plume migration and reservoir pressure buildup. However, such modeling is challenging at scale due to the high computational costs of existing numerical methods. We introduce a novel machine learning approach for four-dimensional spatial-temporal modeling, which speeds up predictions nearly 700,000 times compared to existing methods. It provides highly accurate predictions under diverse reservoir conditions, geological heterogeneity, and injection schemes. Our framework, Nested Fourier Neural Operator (FNO), learns the solution operator for the family of partial differential equations governing the carbon dioxide-water multiphase flow. It uses a hierarchy of FNO models to produce outputs at different refinement levels. Thus, our approach enables unprecedented real-time high-resolution modeling for carbon dioxide storage.
更多查看译文
关键词
carbon capture,fourier neural
AI 理解论文
溯源树
样例
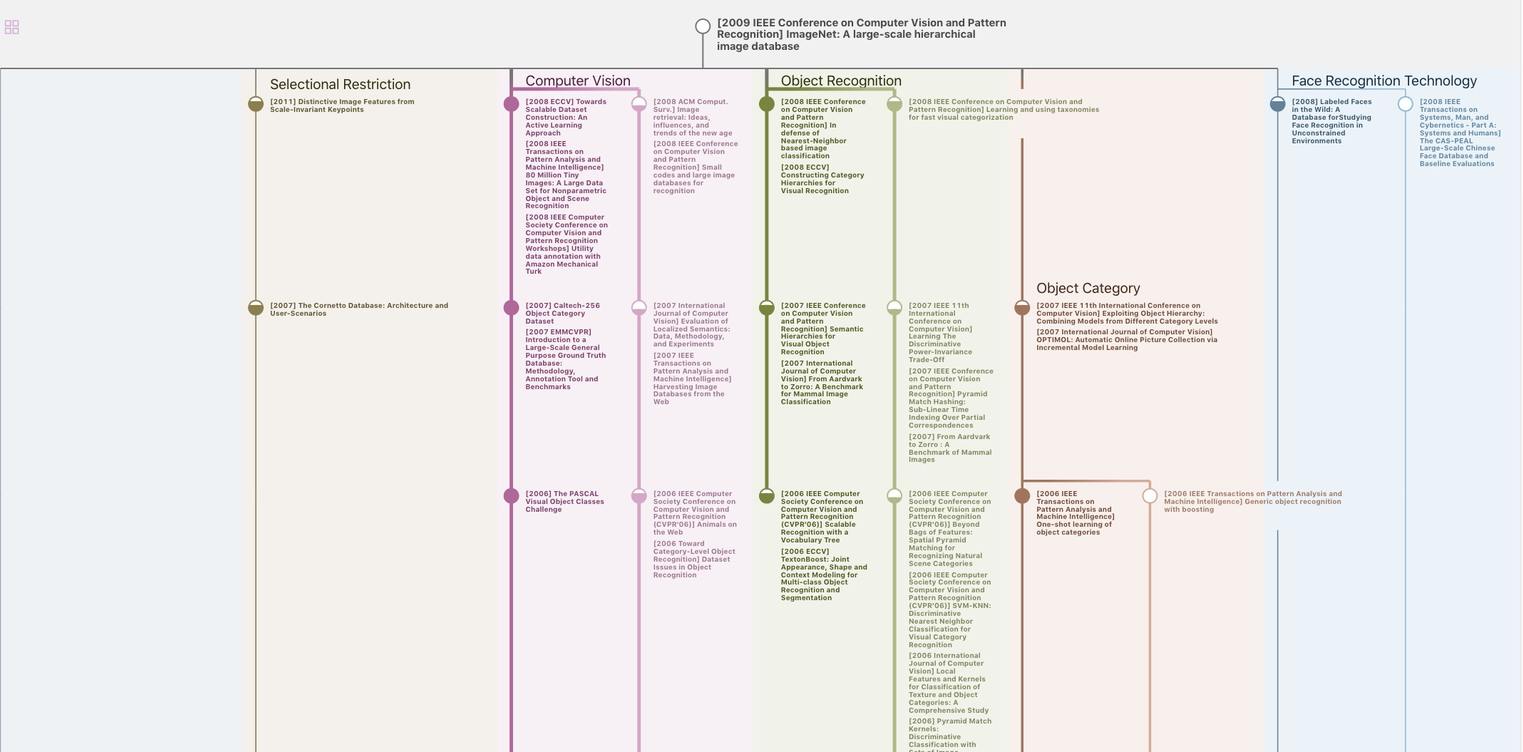
生成溯源树,研究论文发展脉络
Chat Paper
正在生成论文摘要