Digital twins of physical printing-imaging channel
2022 IEEE International Workshop on Information Forensics and Security (WIFS)(2022)
摘要
In this paper, we address the problem of modeling a printing-imaging channel built on a machine learning approach a.k.a. digital twin for anti-counterfeiting applications based on copy detection patterns (CDP). The digital twin is formulated on an information-theoretic framework called Turbo that uses variational approximations of mutual information developed for both encoder and decoder in two-directional information passage. The proposed model generalizes several state-of-the-art architectures such as adversarial autoencoder (AAE) [1], CycleGAN [2] and adversarial latent space auto-encoder (ALAE) [3]. This model can be applied to any type of printing and imaging and it only requires training data consisting of digital templates or artworks that are sent to a printing device and data acquired by an imaging device. Moreover, these data can be paired, unpaired or hybrid paired-unpaired which makes the proposed architecture very flexible and scalable to many practical setups. We demonstrate the impact of various architectural factors, metrics and discriminators on the overall system performance in the task of generation/prediction of printed CDP from their digital counterparts and vice versa. We also compare the proposed system with several state-of-the-art methods used for image-to-image translation applications. The code and extended results of the simulation are publicly available
1
.
更多查看译文
关键词
Copy detection patterns,machine learning,digital twin,information theory,variational approximation
AI 理解论文
溯源树
样例
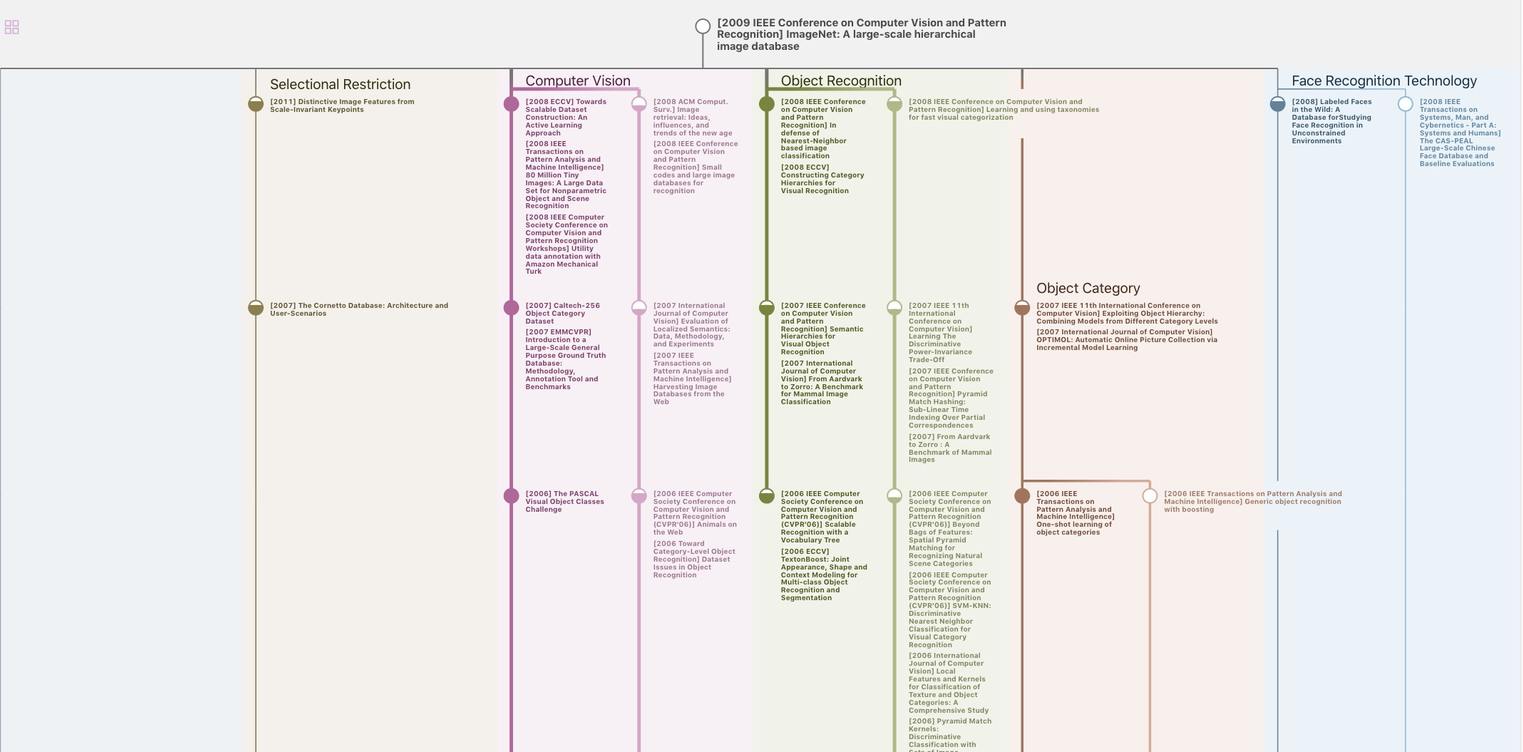
生成溯源树,研究论文发展脉络
Chat Paper
正在生成论文摘要