A Simple, interpretable method to identify surprising topic shifts in scientific fields.
Frontiers in research metrics and analytics(2022)
摘要
This paper proposes a text-mining framework to systematically identify vanishing or newly formed topics in highly interdisciplinary and diverse fields like cognitive science. We apply topic modeling via non-negative matrix factorization to cognitive science publications before and after 2012; this allows us to study how the field has changed since the revival of neural networks in the neighboring field of AI/ML. Our proposed method represents the two distinct sets of topics in an interpretable, common vector space, and uses an entropy-based measure to quantify topical shifts. Case studies on vanishing (e.g., connectionist/symbolic AI debate) and newly emerged (e.g., art and technology) topics are presented. Our framework can be applied to any field or any historical event considered to mark a major shift in thought. Such findings can help lead to more efficient and impactful scientific discoveries.
更多查看译文
关键词
cognitive science,entropy,matrix factorization,science of science,topic matching,topic modeling
AI 理解论文
溯源树
样例
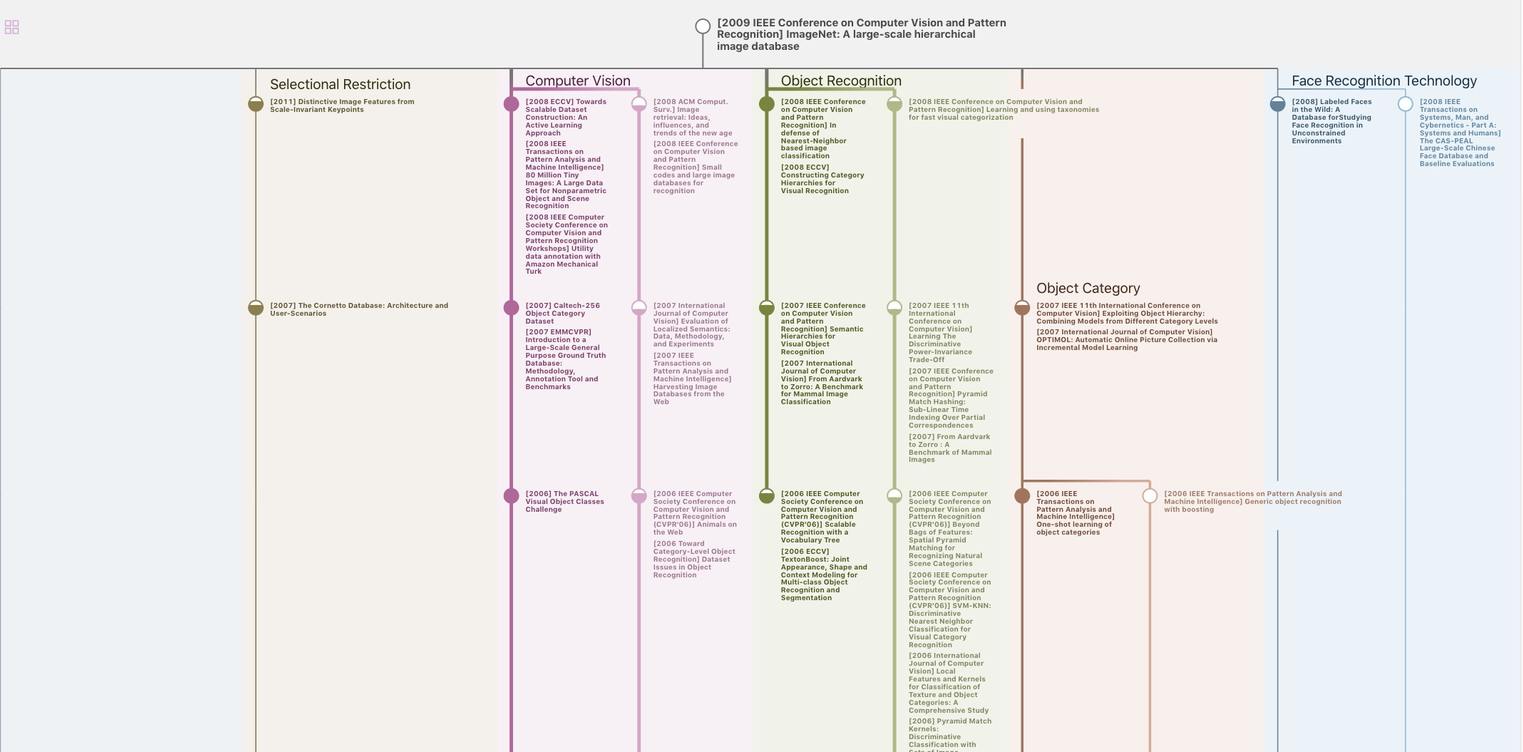
生成溯源树,研究论文发展脉络
Chat Paper
正在生成论文摘要