Effects of Information Masking in the Task-Specific Finetuning of a Transformers-Based Clinical Question-Answering Framework
2022 IEEE 10th International Conference on Healthcare Informatics (ICHI)(2022)
摘要
Transformers-based language models have achieved impressive performance in biomedical question-answering (QA). Our previous work led to surmise that such models could leverage frequent literal question-answer pairs to get the correct answers, casting doubt on true intelligence and transferability. Therefore, we conducted experiments by masking the anchor concept in the question and context documents during the fine-tuning stage of BERT for a reading comprehension QA task on clinical notes. The perturbation involved randomly replacing 0%, 10%, 20%, 30%, and 100% of the concept occurrences into a dummy string. We found the 100% masking harshly penalized the overall accuracy by about 0.10 versus 0% masking. However, the accuracy improved about 0.01 to 0.02 at 20% masking - and the benefit was able to transfer when tested on a different corpus. We also found the masking preferably enhanced the accuracy for question-answer pairs of the top 20%-40% frequent in the train set. The results suggested that transformers-based QA systems may benefit from moderate masking during fine-tuning, likely by forcing the model to learn abstract context patterns rather than relying on specific surface terms or relations. The beneficial effect skewed toward a specific non-top frequency tier could reflect a more general phenomenon in machine learning where such enhancement techniques are most effective for cases that sit around the make-or-fail border.
更多查看译文
关键词
Natural Language Processing,Deep Learning,Question Answering,Electronic Health Records,Supervised Machine Learning
AI 理解论文
溯源树
样例
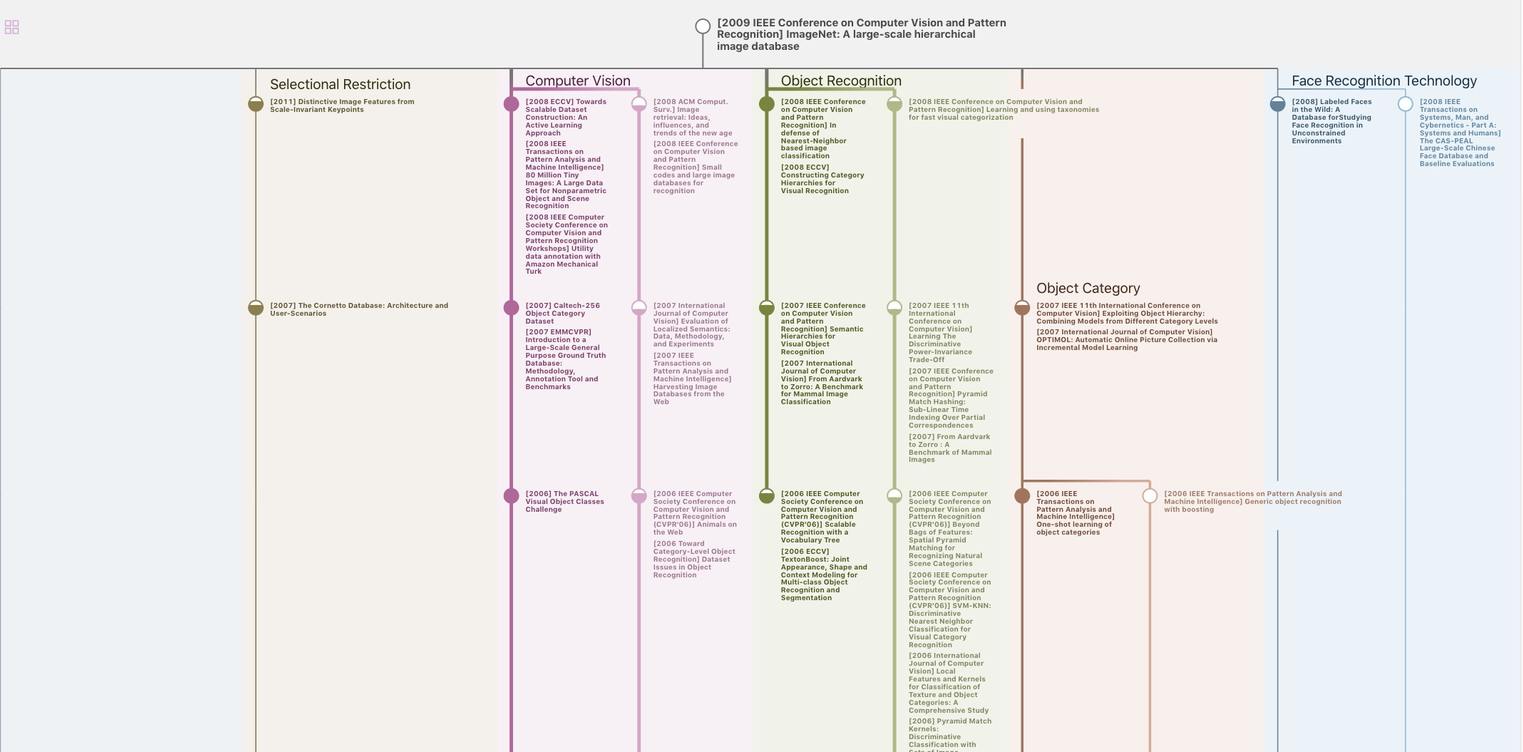
生成溯源树,研究论文发展脉络
Chat Paper
正在生成论文摘要