On-Ramp Merging for Connected Autonomous Vehicles using Deep Reinforcement Learning
2022 IEEE International Conferences on Internet of Things (iThings) and IEEE Green Computing & Communications (GreenCom) and IEEE Cyber, Physical & Social Computing (CPSCom) and IEEE Smart Data (SmartData) and IEEE Congress on Cybermatics (Cybermatics)(2022)
摘要
Ramp merging is a major challenge which leads to bottleneck congestion on the freeway, rear-end and side collisions. The automated vehicle must safely execute this complex action in order to optimize long-term objectives (e.g. successful and smooth merge). The lane merging is a joint behavior consisting of lateral steering maneuvers for lane merge and longitudinal throttle based motions with a continuous awareness about the road topology and neighboring vehicles. A reinforcement learning based Deep Deterministic Policy Gradient model is known to give smooth driving maneuvers in simulated environments which is used to control the vehicle throttle acceleration for a quality traffic flow. The Deep Q Network enables the ego vehicle to safely merge on the highway while monitoring the states of road topology and its own vehicle dynamics. Based on the discrete and continuous actions taken by these two methods, the simulation result validate the improvement in the trained model of ego vehicle dynamics under ramp merging conditions.
更多查看译文
关键词
Connected Autonomous vehicles,Ramp merging,vehicle dynamics,traffic flow,DQN,DDPG
AI 理解论文
溯源树
样例
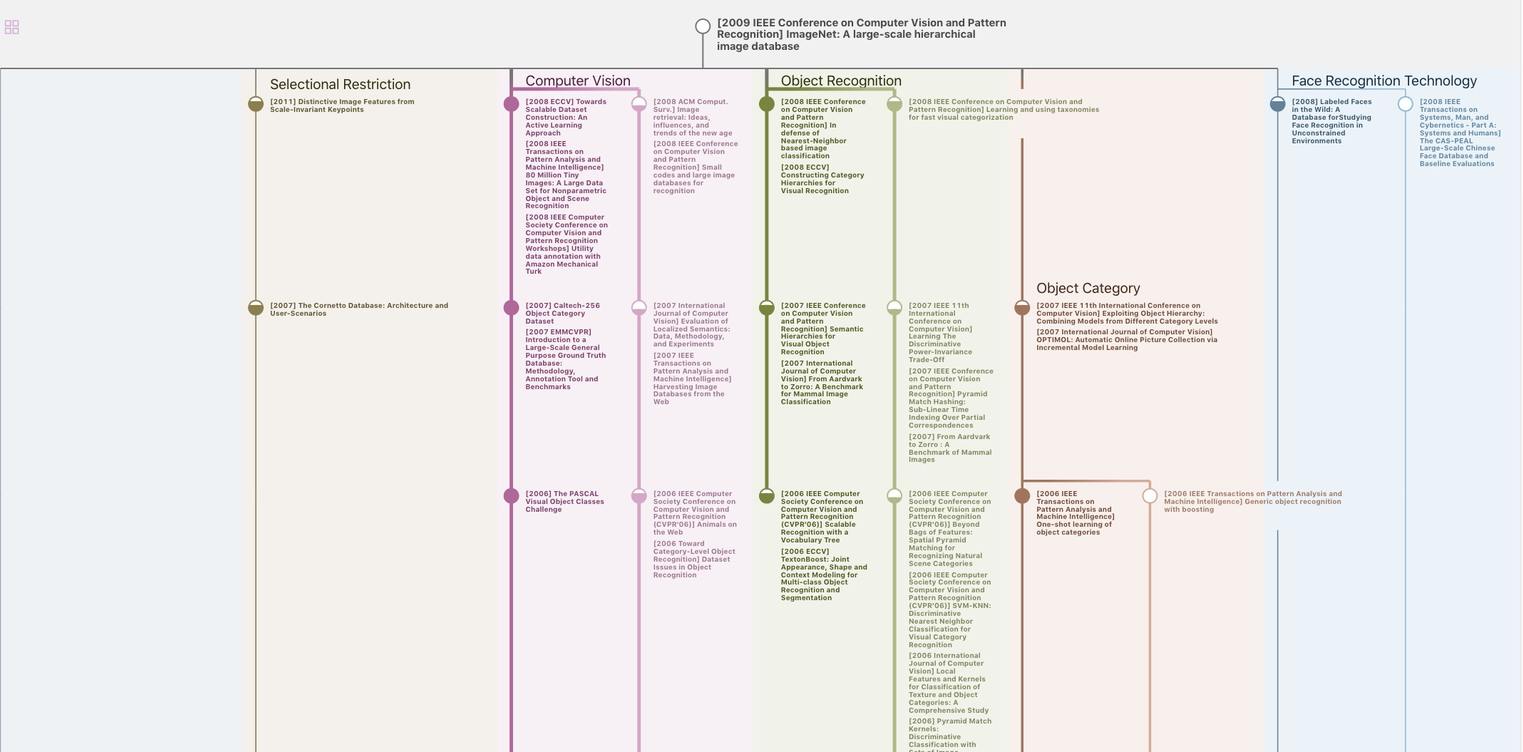
生成溯源树,研究论文发展脉络
Chat Paper
正在生成论文摘要