Adaptive Sampling for Efficient Acoustic Noise Monitoring: an Incremental Learning Approach
2022 IEEE International Conferences on Internet of Things (iThings) and IEEE Green Computing & Communications (GreenCom) and IEEE Cyber, Physical & Social Computing (CPSCom) and IEEE Smart Data (SmartData) and IEEE Congress on Cybermatics (Cybermatics)(2022)
摘要
Sensor nodes deployed for environmental monitoring usually have access to limited resources. They need a way to prioritize observations in real time in order to maximize the utility of its resource constrained system within its limited budget. Whereas static sampling policies fail to adapt in dynamic environments, we propose online sampling policies that autonomously adapt the sampling rate to the rate of change in the environment. In this paper, we study the use of incremental machine learning models to develop adaptive sensing policies based on incremental prediction models. We applied the proposed approach in the context of noise monitoring. Our numerical results show that we can extend the battery lifetime of such a sensing system from 9 days to over 2 years (790 days) with energy saving of 97%, while keeping the accuracy of the monitoring task at 94% of the accuracy obtained using the maximum sampling rate.
更多查看译文
关键词
Adaptive Sampling,Incremental Learning,Internet of Things,Machine Learning,Mondrian Forests,Pareto Front,Quantile Regression Forests,Random Forests,Sensors,Wireless Sensor Networks
AI 理解论文
溯源树
样例
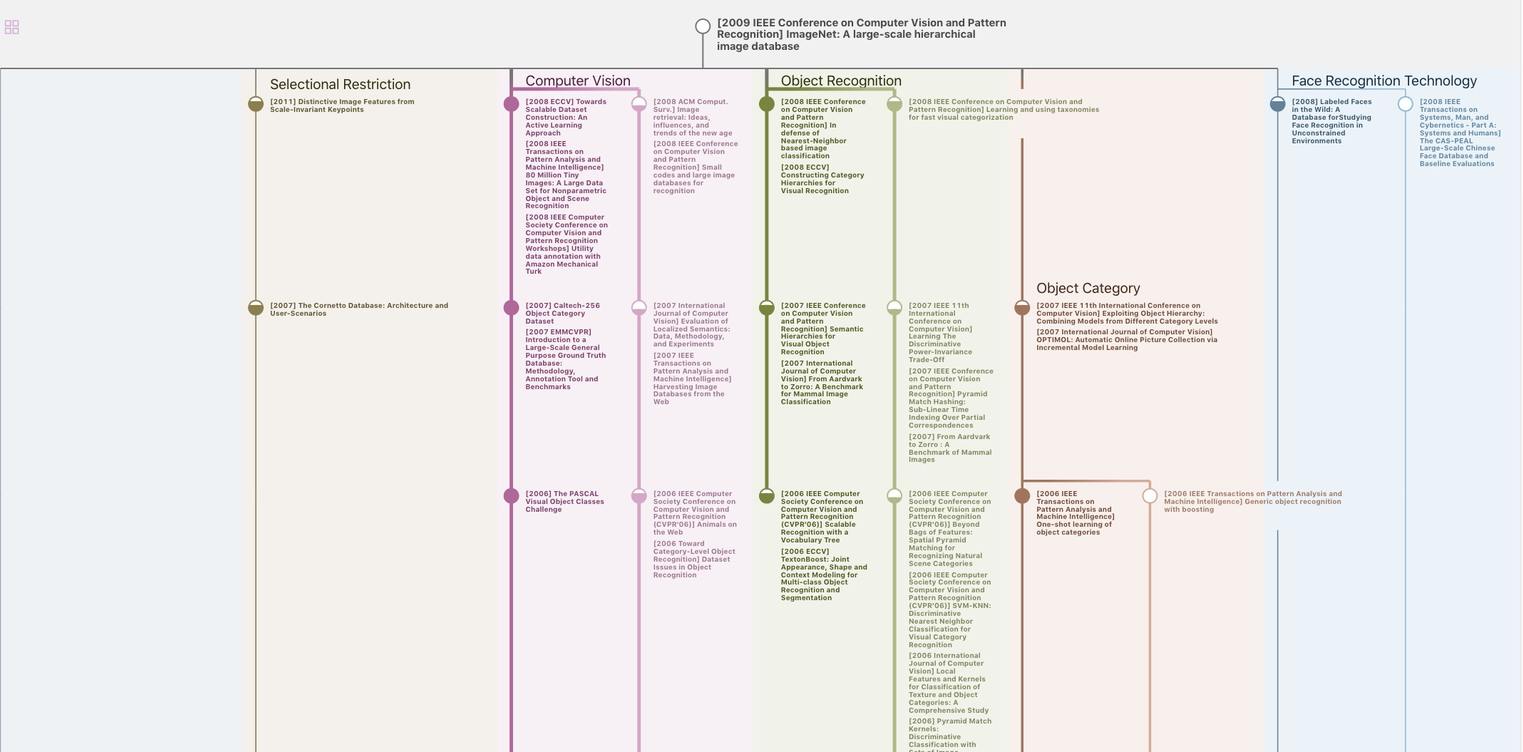
生成溯源树,研究论文发展脉络
Chat Paper
正在生成论文摘要