A Deep Curriculum Learner in an Active Learning Cycle for Polsar Image Classification
IGARSS 2022 - 2022 IEEE International Geoscience and Remote Sensing Symposium(2022)
摘要
The integration of deep learning and active learning has achieved great success in polarimetric synthetic aperture radar (PolSAR) image classification. However, the training samples provided by the active learning approach are inade-quate to promote the performance of deep learning methods. Also, in the initial learning stages, querying a small amount of informative and complex samples, which are plagued by significant speckle noise, not only increases the risk of overfitting, but also makes the further annotations of less importance. To alleviate these problems, by utilization of curriculum learning (CL), we propose a novel classification method for PolSAR images, considering the complexity of informative samples before applying them to the deep learning model. Furthermore, we develop a new lightweight 3D convolutional neural network with high-level feature extraction ability while having a very low computational cost. Experimental results with the two PolSAR benchmark data sets of AIRSAR Flevoland and ESAR Oberpfaffenhofen indicate our proposed method achieved the state-of-the-art classification results with a significantly smaller amount of training data.
更多查看译文
关键词
Active learning,Deep curriculum learning,SAR polarimetry data classification,Lightweight 3D convolution
AI 理解论文
溯源树
样例
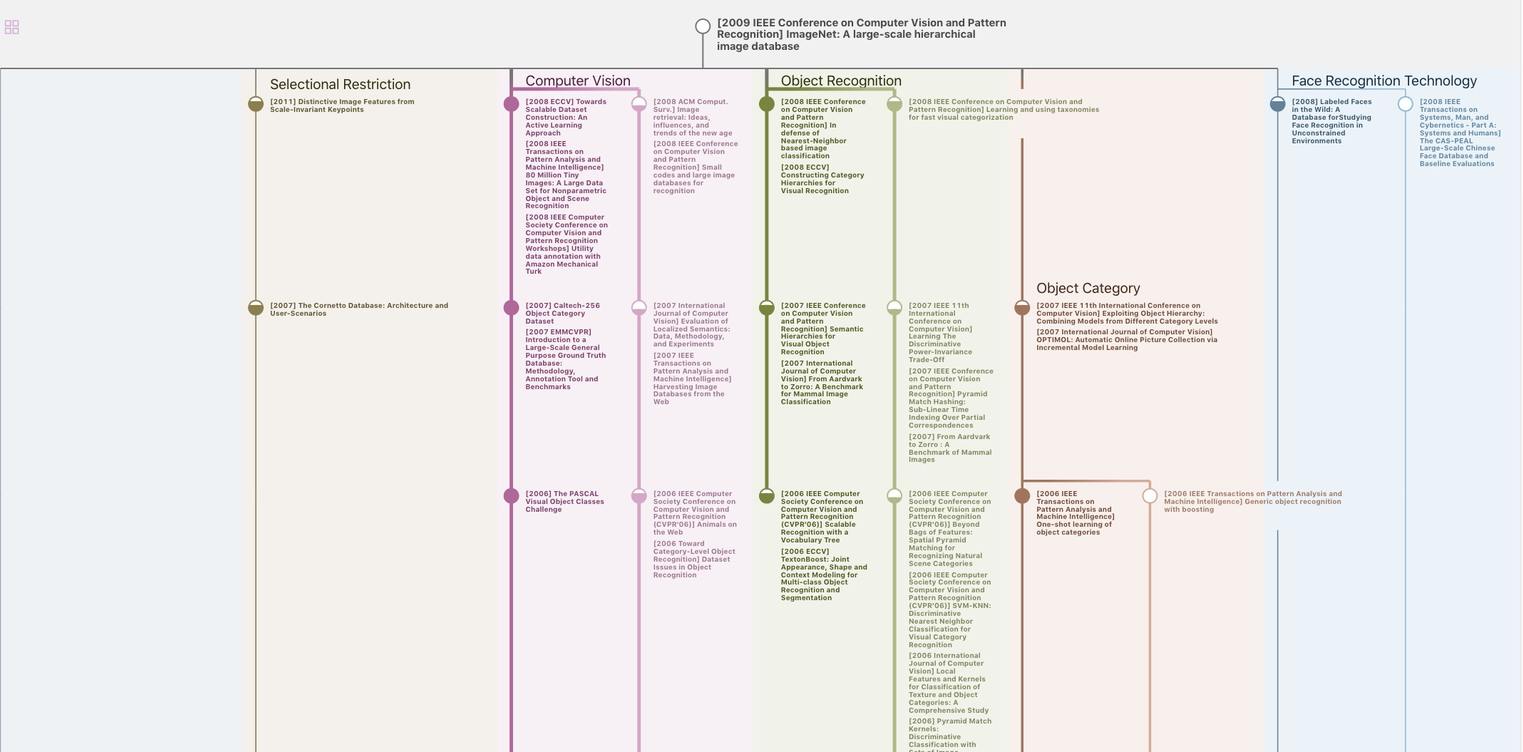
生成溯源树,研究论文发展脉络
Chat Paper
正在生成论文摘要