Disentangled (Un)Controllable Features
arxiv(2022)
摘要
In the context of MDPs with high-dimensional states, reinforcement learning can achieve better results when using a compressed, low-dimensional representation of the original input space. A variety of learning objectives have therefore been used to learn useful representations. However, these representations usually lack interpretability of the different features. We propose a representation learning algorithm that is able to disentangle latent features into a controllable and an uncontrollable part. The resulting representations are easily interpretable and can be used for learning and planning efficiently by leveraging the specific properties of the two parts. To highlight the benefits of the approach, the disentangling properties of the algorithm are illustrated in three different environments.
更多查看译文
关键词
features
AI 理解论文
溯源树
样例
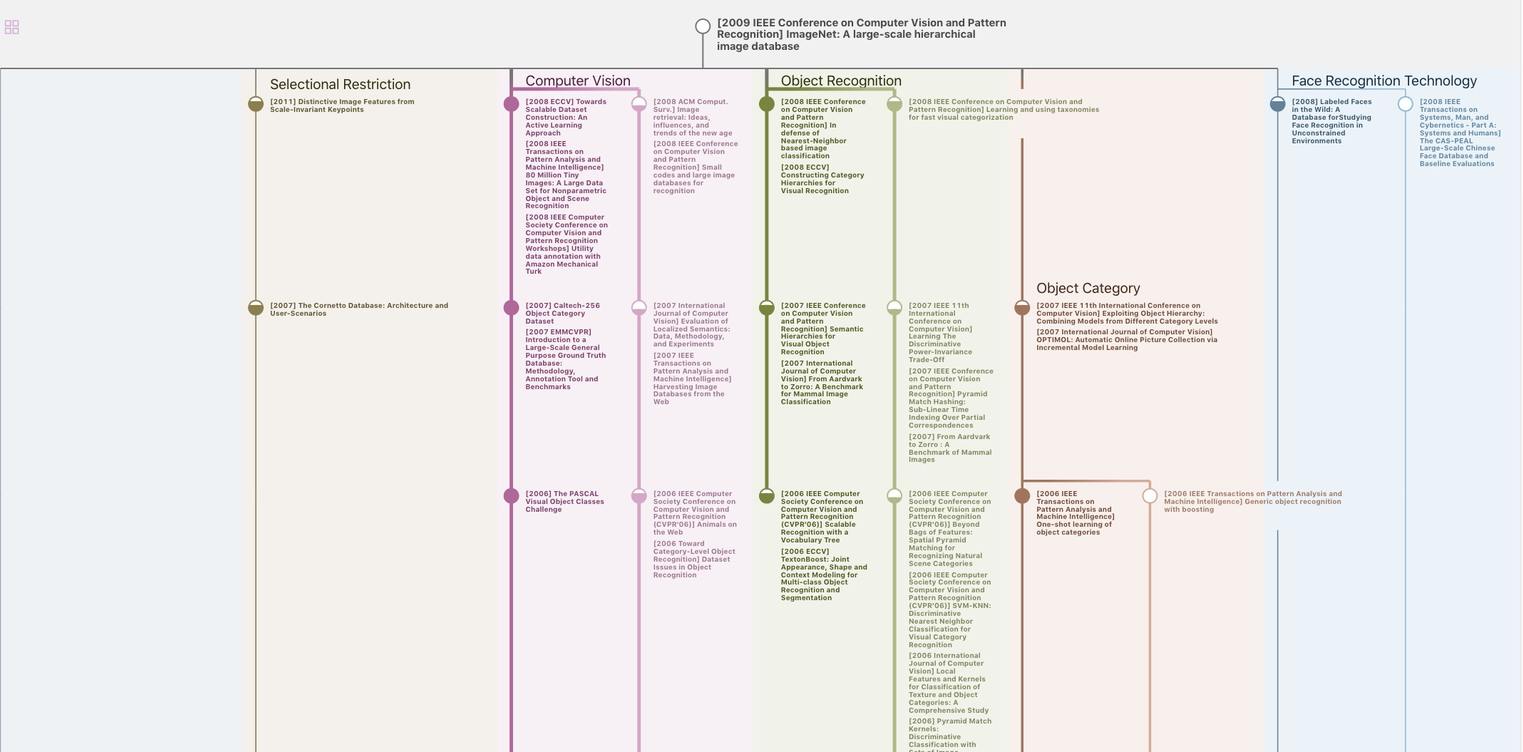
生成溯源树,研究论文发展脉络
Chat Paper
正在生成论文摘要