Reservoir Computing for Macroeconomic Forecasting with Mixed-Frequency Data
International journal of forecasting(2024)
摘要
Macroeconomic forecasting has recently started embracing techniques that can deal with large-scale datasets and series with unequal release periods. Mixed-data sampling (MIDAS) and dynamic factor models (DFMs) are the two main state-of-the-art approaches to modeling series with non-homogeneous frequencies. We introduce a new framework, called the multi-frequency echo state network (MFESN), based on a relatively novel machine learning paradigm called reservoir computing. Echo state networks (ESNs) are recurrent neural networks formulated as nonlinear state-space systems with random state coefficients where only the observation map is subject to estimation. MFESNs are considerably more efficient than DFMs and can incorporate many series, as opposed to MIDAS models, which are prone to the curse of dimensionality. All methods are compared in extensive multistep forecasting exercises targeting U.S. GDP growth. We find that our MFESN models achieve superior or comparable performance over MIDAS and DFMs at a much lower computational cost.
更多查看译文
关键词
Reservoir computing,Echo state networks,Forecasting,U.S. output growth,GDP,Mixed-frequency data,Time series,Multi-Frequency Echo State Network,MIDAS,DFM
AI 理解论文
溯源树
样例
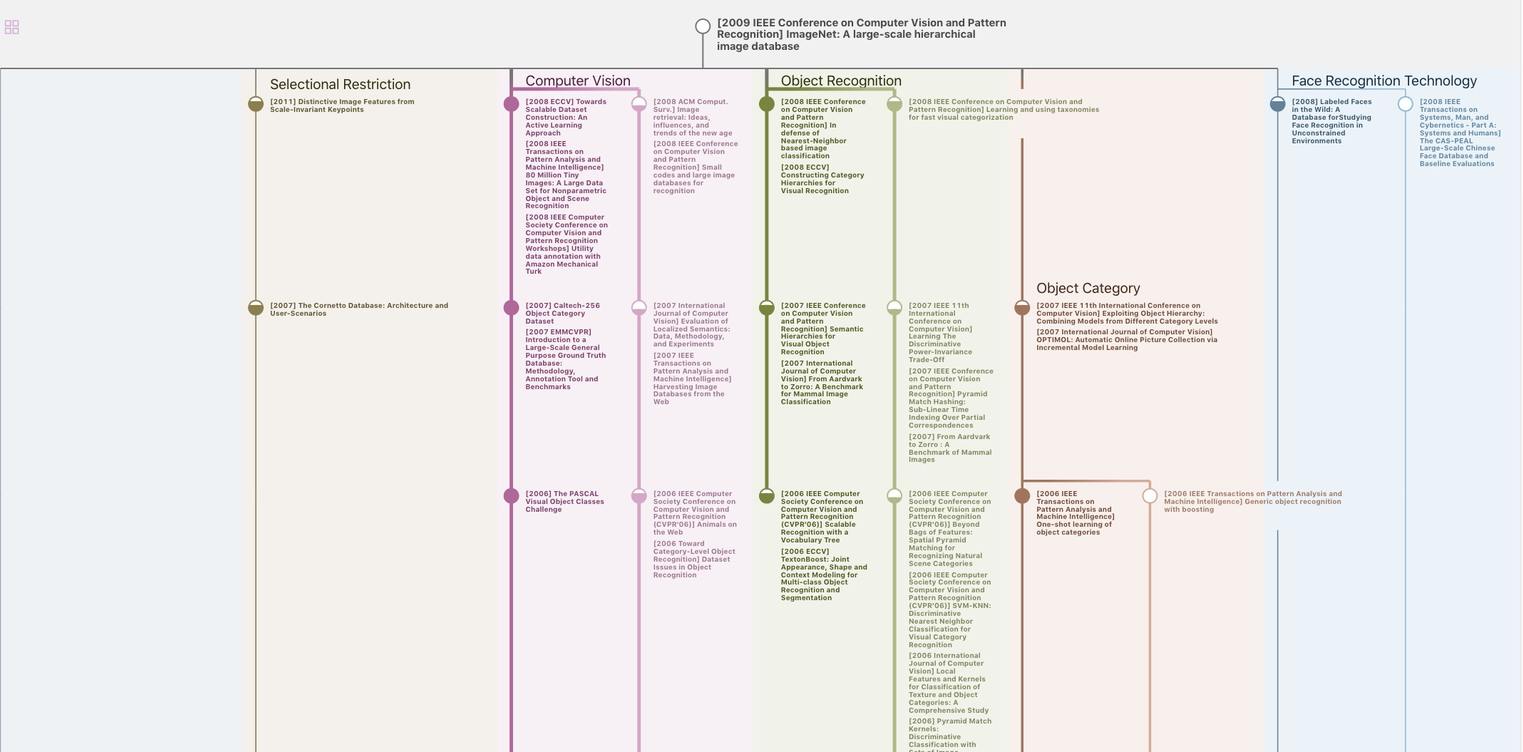
生成溯源树,研究论文发展脉络
Chat Paper
正在生成论文摘要