Deep Learning for Landslide Detection and Segmentation in High-Resolution Optical Images along the Sichuan-Tibet Transportation Corridor
REMOTE SENSING(2022)
摘要
Landslides pose a greater potential risk to the Sichuan-Tibet Transportation Project, and extensive landslide inventory and mapping are essential to prevent and control geological hazards along the Sichuan-Tibet Transportation Corridor (STTC). Recently proposed landslide detection methods mainly focused on new landslides with high vegetation. In addition, there are still challenges in automatic detection of old landslides using optical images. In this paper, two methods, namely mask region-based convolutional neural networks (Mask R-CNN) and transfer learning Mask R-CNN (TL-Mask R-CNN), are presented for detecting and segmenting new and old landslides, respectively. An optical remote sensing dataset for landslide recognition along the Sichuan-Tibet Transportation Corridor (LRSTTC) is constructed as an evaluation benchmark. Our experimental results show that the recall rate and F1-score of the proposed method for new landslide detection can reach 78.47% and 79.80%, respectively. Transfer learning is adopted to detect old landslides, and our experimental results show that evaluation indices can be further improved by about 10%. Furthermore, TL-Mask R-CNN has been applied to identify ice avalanches based on the characteristics of landslides. It appears that our proposed methods can detect and segment landslides effectively along the STTC with the constructed LRSTTC dataset, which is essential for studying and preventing landslide hazards in mountainous areas.
更多查看译文
关键词
landslide hazards,transfer learning,Mask R-CNN,detection and segmentation,Sichuan-Tibet transportation corridor
AI 理解论文
溯源树
样例
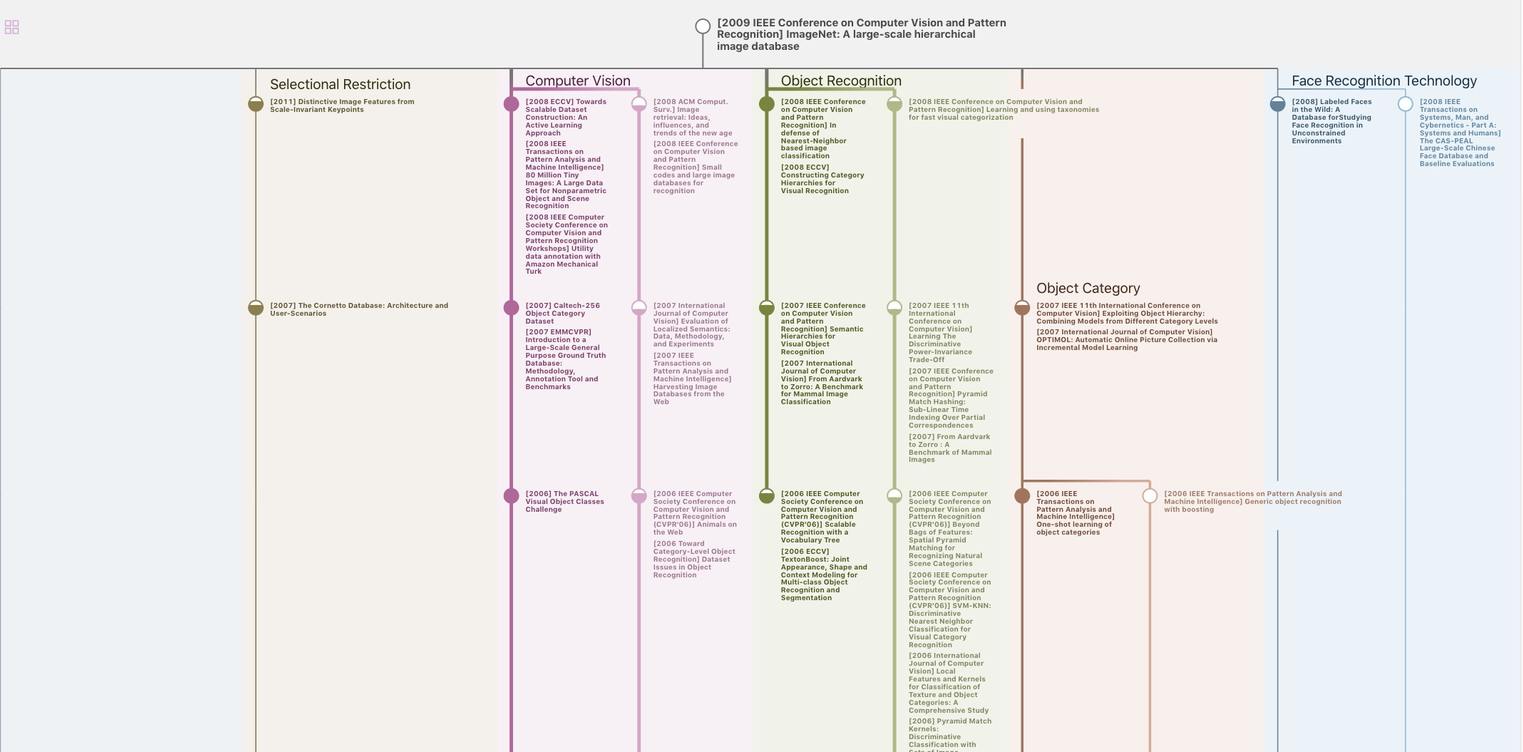
生成溯源树,研究论文发展脉络
Chat Paper
正在生成论文摘要