Learning the shape of protein microenvironments with a holographic convolutional neural network
PROCEEDINGS OF THE NATIONAL ACADEMY OF SCIENCES OF THE UNITED STATES OF AMERICA(2024)
摘要
Proteins play a central role in biology from immune recognition to brain activity. While major advances in machine learning have improved our ability to predict protein structure from sequence, determining protein function from its sequence or structure remains a major challenge. Here, we introduce holographic convolutional neural network (H-CNN) for proteins, which is a physically motivated machine learning approach to model amino acid preferences in protein structures. H-CNN reflects physical interactions in a protein structure and recapitulates the functional information stored in evolutionary data. H-CNN accurately predicts the impact of mutations on protein stability and binding of protein complexes. Our interpretable computational model for protein structure-function maps could guide design of novel proteins with desired function.
更多查看译文
关键词
protein science,protein structure-function map,machine learning,geometric deep learning,rotationally equivariant convolutional neural network
AI 理解论文
溯源树
样例
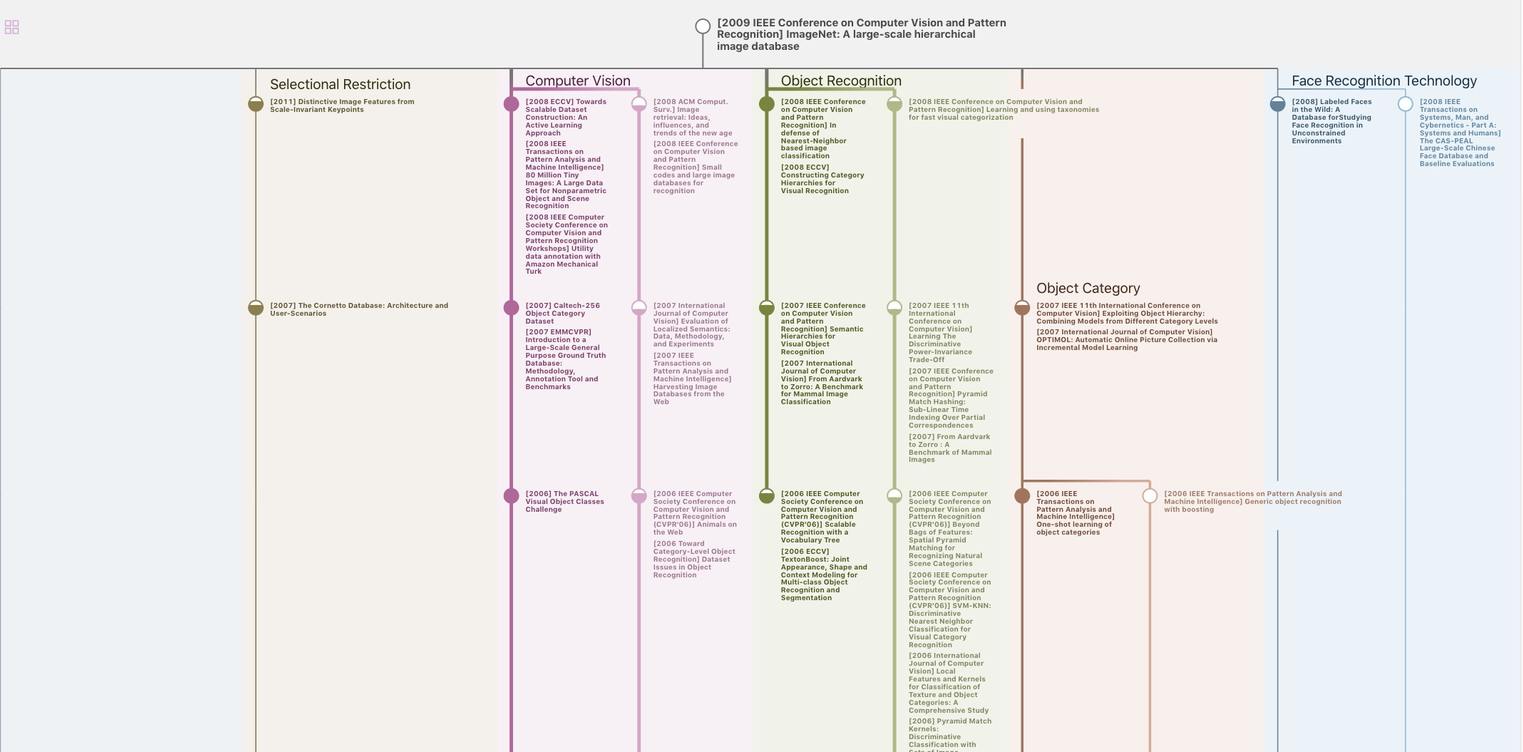
生成溯源树,研究论文发展脉络
Chat Paper
正在生成论文摘要