Chinese Grammatical Error Correction Using Pre-trained Models and Pseudo Data
ACM Transactions on Asian and Low-Resource Language Information Processing(2023)
摘要
In recent studies, pre-trained models and pseudo data have been key factors in improving the performance of the English grammatical error correction (GEC) task. However, few studies have examined the role of pre-trained models and pseudo data in the Chinese GEC task. Therefore, we develop Chinese GEC models based on three pre-trained models: Chinese BERT, Chinese T5, and Chinese BART, and then incorporate these models with pseudo data to determine the best configuration for the Chinese GEC task. On the natural language processing and Chinese computing (NLPCC) 2018 GEC shared task test set, all our single models outperform the ensemble models developed by the top team of the shared task. Chinese BART achieves an F score of 37.15, which is a state-of-the-art result. We then combine our Chinese GEC models with three kinds of pseudo data: Lang-8 (MaskGEC), Wiki (MaskGEC), and Wiki (Backtranslation). We find that most models can benefit from pseudo data, and BART+Lang-8 (MaskGEC) is the ideal setting in terms of accuracy and training efficiency. The experimental results demonstrate the effectiveness of the pre-trained models and pseudo data on the Chinese GEC task and provide an easily reproducible and adaptable baseline for future works. Finally, we annotate the error types of the development data; the results show that word-level errors dominate all error types, and word selection errors must be addressed even when using pre-trained models and pseudo data. Our codes are available at https://github.com/wang136906578/BERT-encoder-ChineseGEC .
更多查看译文
关键词
NLP education application,pre-trained model,pseudo data,Chinese grammatical error correction
AI 理解论文
溯源树
样例
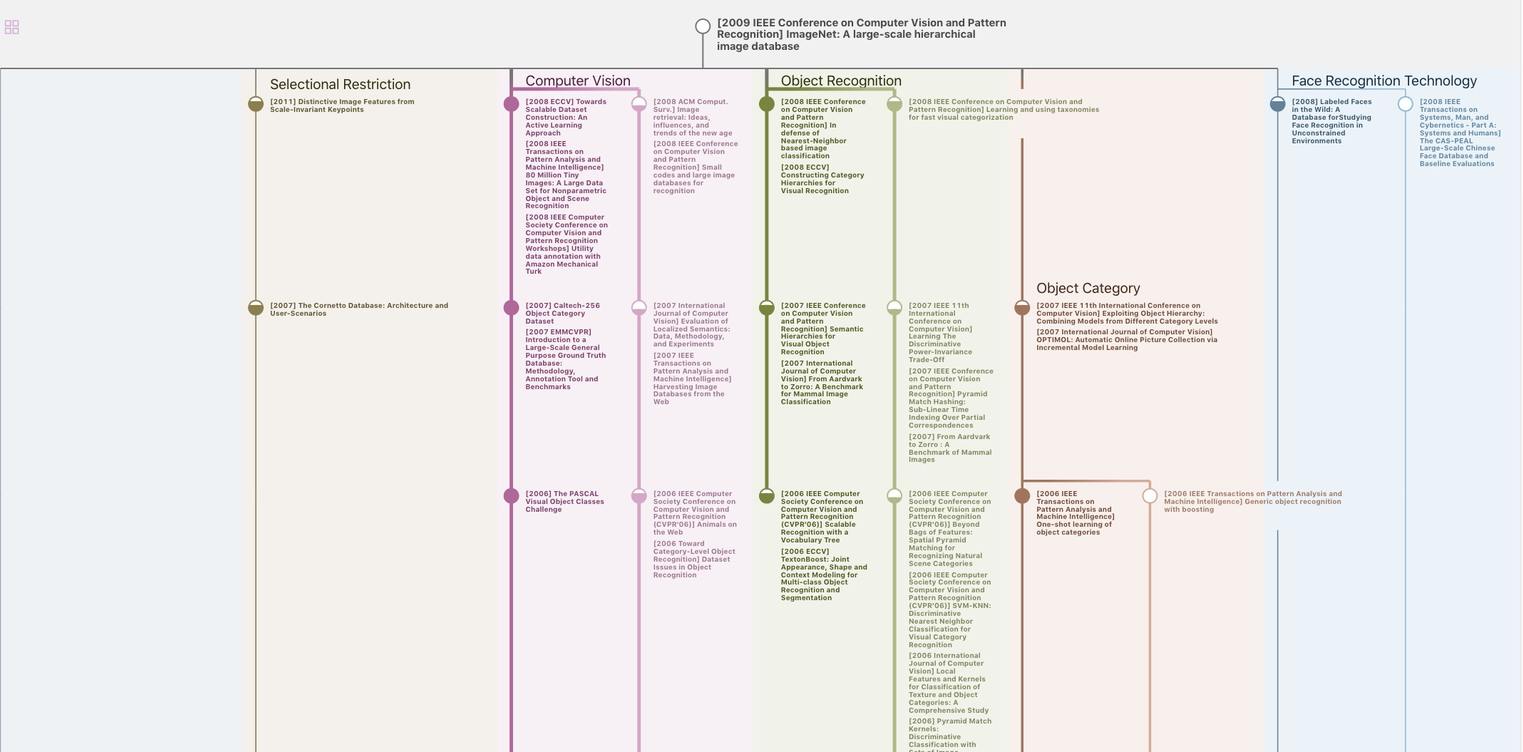
生成溯源树,研究论文发展脉络
Chat Paper
正在生成论文摘要